Review of improved A.I. based Image Segmentation for medical diagnosis applications
P. Ranjan1 , P.R. Khan2
Section:Review Paper, Product Type: Journal Paper
Volume-4 ,
Issue-11 , Page no. 75-81, Nov-2016
Online published on Nov 29, 2016
Copyright © P. Ranjan, P.R. Khan . This is an open access article distributed under the Creative Commons Attribution License, which permits unrestricted use, distribution, and reproduction in any medium, provided the original work is properly cited.
View this paper at Google Scholar | DPI Digital Library
How to Cite this Paper
- IEEE Citation
- MLA Citation
- APA Citation
- BibTex Citation
- RIS Citation
IEEE Style Citation: P. Ranjan, P.R. Khan, “Review of improved A.I. based Image Segmentation for medical diagnosis applications,” International Journal of Computer Sciences and Engineering, Vol.4, Issue.11, pp.75-81, 2016.
MLA Style Citation: P. Ranjan, P.R. Khan "Review of improved A.I. based Image Segmentation for medical diagnosis applications." International Journal of Computer Sciences and Engineering 4.11 (2016): 75-81.
APA Style Citation: P. Ranjan, P.R. Khan, (2016). Review of improved A.I. based Image Segmentation for medical diagnosis applications. International Journal of Computer Sciences and Engineering, 4(11), 75-81.
BibTex Style Citation:
@article{Ranjan_2016,
author = {P. Ranjan, P.R. Khan},
title = {Review of improved A.I. based Image Segmentation for medical diagnosis applications},
journal = {International Journal of Computer Sciences and Engineering},
issue_date = {11 2016},
volume = {4},
Issue = {11},
month = {11},
year = {2016},
issn = {2347-2693},
pages = {75-81},
url = {https://www.ijcseonline.org/full_paper_view.php?paper_id=1111},
publisher = {IJCSE, Indore, INDIA},
}
RIS Style Citation:
TY - JOUR
UR - https://www.ijcseonline.org/full_paper_view.php?paper_id=1111
TI - Review of improved A.I. based Image Segmentation for medical diagnosis applications
T2 - International Journal of Computer Sciences and Engineering
AU - P. Ranjan, P.R. Khan
PY - 2016
DA - 2016/11/29
PB - IJCSE, Indore, INDIA
SP - 75-81
IS - 11
VL - 4
SN - 2347-2693
ER -
![]() |
![]() |
![]() |
1490 | 1345 downloads | 1387 downloads |
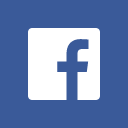
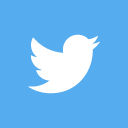
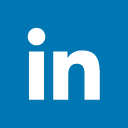
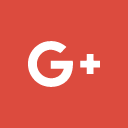
Abstract
Image segmentation is very important application in a biomedical diagnosis use image data analysis. In medical analysis the accuracy of image segmentation has a critical clinical requirement for the localization of body organs or pathologies in order to raise the quality of prediction of disease or infections. This paper covers review that includes several articles in which latest A.I biomedical image segmentation techniques are applied to different imaging color space models. This review article describes how various computer assisted diagnosis system works for achieving the goal of finding abnormal segments of body organs in biomedical images of the MRI, ultrasound etc. It has been observed that those segmentation approach are broadly giving accurate results in which the segmentation of the images is performed by defining an active shape model and then localization of potential area of interest using thresholding.
Key-Words / Index Term
Image processing, biomedical analysis, detection, pattern recognition
References
Rastgarpour M., and Shanbehzadeh J., Application of AI Techniques in Medical Image Segmentation and Novel Categorization of Available Methods and Tools, Proceedings of the International MultiConference of Engineers and Computer Scientists 2011 Vol I, IMECS 2011, March 16-18, 2011, Hong Kong.
[2] Zhang, Y. J, An Overview of Image and Video Segmentation in the last 40 years, Proceedings of the 6th International Symposium on Signal Processing and Its Applications, pp. 144-151, 2001.
[3] Wahba Marian, An Automated Modified Region Growing Technique for Prostate Segmentation in Trans Rectal Ultrasound Images, Master�s Thesis, Department of Electrical and Computer Engineering, University of Waterloo, Waterloo, Ontario, Canada, 2008.
[4] Li CH, Tam PKS (1998) An iterative algorithm for minimum cross-entropy thresholding. Pattern Recognit Lett 19(8):771�776.
[5] Sezgin M, Sankur B (2004) Survey over image thresholding techniques and quantitative performance evaluation. J Electron Imaging 13(1):146�168 1005
[6] Haralick RM, Shapiro LG (1985) Image segmentation techniques. Comput Vis Graph Image Process 29(1):100�132 1009
[7] Hojjatoleslami SA, Kruggel F (2001) Segmentation of large brain lesions. IEEE Trans Med Imaging 20:666�669 1011
[8] Wan S-Y, HigginsWE (2003) Symmetric region growing. IEEE Trans Image Process 12(9): 1007�1015 1013
[9] Mendonca AM, Campilho A (2006) Segmentation of retinal blood vessels by combining the detection of centerlines and morphological reconstruction. IEEE Trans Med Imaging 25: 1200�1213
[10] Dehmeshki J, Member HA, Valdivieso M, Ye X (2008) Segmentation of pulmonary nodules in thoracic CT scans: a region growing approach. IEEE Trans Image Process 27(4):467�480
[11] Rogowska J (2000) Overview and fundamentals of medical image segmentation. In: 962 Bankman I (ed) Handbook of medical image processing and analysis. Elsevier, Amsterdam, 963 The Netherlands, pp 69�85
[12] Geman S, Geman D (1984) Stochastic relaxation, Gibbs distributions, and Bayesian restoration of images. IEEE Trans Pattern Anal Mach Intell 6(6):721�741
[13] Scott DW (1992) Multivariate density estimation: theory, practice, and visualization. Wiley, New York
[14] Duda RO, Hart PE, Stork DG (2001) Pattern classification, 2nd edn. Wiley, New York.
[15] Rohlfing T, Brandt R, Menzel R, Russakoff DB,Maurer CR Jr (2005) Quo vadis, atlas-based segmentation? In: Suri JS, Wilson DL, Laxminarayan S (eds) Handbook of Biomedical Image Analysis, vol III: Registration Models. Kluwer Academic/Plenum Publishers, New York, pp 435�486, chapter 11
[16] Isgum I, Staring M, Rutten A, Prokop M, Viergever MA, van Ginneken B (2009) Multiatlas-based segmentation with local decision fusion-application to cardiac and aortic segmentation in CT scans. IEEE Trans Med Imaging 28(7):1000�1010.
[17] Stancanello J, Romanelli P, Modugno N, Cerveri P, Ferrigno G, Uggeri F, Cantore G (2006) Atlas-based identification of targets for functional radiosurgery. Med Phys 33(6):1603�1611.
[18] Li B, Christensen GE, Hoffman EA, McLennan G, Reinhardt JM (2003) Establishing a normative atlas of the human lung: intersubject warping and registration of volumetric CT images. Acad Radiol 10(3):255�265
[19] Park H, Bland PH, Meyer CR (2003) Construction of an abdominal probabilistic atlas and its application in segmentation. IEEE Trans Med Imaging 22(4):483�492
[20] Leemput KV (2009) Encoding probabilistic brain atlases using Bayesian inference. IEEE Trans Med Imaging 28(6):822�837.
[21] Kittler J, Hatef M, Duin RPW, Matas J (1998) On combining classifiers. IEEE Trans Pattern Anal Mach Intell 20(3):226�239
[22] Artaechevarria X, Munoz-Barrutia A, Ortiz-de-Solorzano C (2009) Combination strategies in multi-atlas image segmentation: application to brain MR data. IEEE Trans Med Imaging 28(8):1266�1277
[23] Rohlfing T, Russakoff DB, Maurer CR (2004) Performance-based classifier combination in atlas-based image segmentation using expectation-maximization parameter estimation. IEEE Trans Med Imaging 23(8):983�994.
[24] Rohlfing T, Maurer CR Jr (2007) Shape-based averaging. IEEE Trans Image Process 16(1): 153�161
[25] Kass M, Witkin A, Terzopoulos D (1987) Snakes: active contour models. Int J Comput Vis 1(4):321�331
[26] Duta N, Sonka M (1998) Segmentation and interpretation of MR brain images: an improved active shape model. IEEE Trans Med Imaging 17(6):1049�1062
[27] Tsai A, Yezzi A Jr, Wells W, Tempany C, Tucker D, Fan A, Grimson WE, Willsky A (2003) A shape based approach to the segmentation of medical imagery using level sets. IEEE Trans Med Imaging 22(2):137�154