Lung Cancer Classification
D.N. Sonar1 , U.V. Kulkarni2
Section:Research Paper, Product Type: Journal Paper
Volume-4 ,
Issue-12 , Page no. 51-55, Dec-2016
Online published on Jan 02, 2016
Copyright © D.N. Sonar, U.V. Kulkarni . This is an open access article distributed under the Creative Commons Attribution License, which permits unrestricted use, distribution, and reproduction in any medium, provided the original work is properly cited.
View this paper at Google Scholar | DPI Digital Library
How to Cite this Paper
- IEEE Citation
- MLA Citation
- APA Citation
- BibTex Citation
- RIS Citation
IEEE Style Citation: D.N. Sonar, U.V. Kulkarni, “Lung Cancer Classification,” International Journal of Computer Sciences and Engineering, Vol.4, Issue.12, pp.51-55, 2016.
MLA Style Citation: D.N. Sonar, U.V. Kulkarni "Lung Cancer Classification." International Journal of Computer Sciences and Engineering 4.12 (2016): 51-55.
APA Style Citation: D.N. Sonar, U.V. Kulkarni, (2016). Lung Cancer Classification. International Journal of Computer Sciences and Engineering, 4(12), 51-55.
BibTex Style Citation:
@article{Sonar_2016,
author = {D.N. Sonar, U.V. Kulkarni},
title = {Lung Cancer Classification},
journal = {International Journal of Computer Sciences and Engineering},
issue_date = {12 2016},
volume = {4},
Issue = {12},
month = {12},
year = {2016},
issn = {2347-2693},
pages = {51-55},
url = {https://www.ijcseonline.org/full_paper_view.php?paper_id=1131},
publisher = {IJCSE, Indore, INDIA},
}
RIS Style Citation:
TY - JOUR
UR - https://www.ijcseonline.org/full_paper_view.php?paper_id=1131
TI - Lung Cancer Classification
T2 - International Journal of Computer Sciences and Engineering
AU - D.N. Sonar, U.V. Kulkarni
PY - 2016
DA - 2017/01/02
PB - IJCSE, Indore, INDIA
SP - 51-55
IS - 12
VL - 4
SN - 2347-2693
ER -
![]() |
![]() |
![]() |
1814 | 1551 downloads | 1396 downloads |
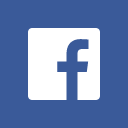
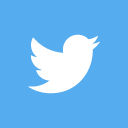
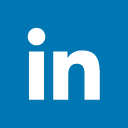
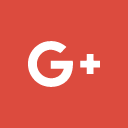
Abstract
Detection and diagnosis of lung cancer from chest radiographs is one of the most important and difficult task for the radiologists. In this paper, combination of statistical texture and moment invariant features are used to classify the lung cancer images. These features are extracted from JSRT raw chest X-ray images. The proposed approach is built on two-level architecture. In the first level architecture images are sharpened and segmented to extract the region of interest i.e. lung from the ribs using image processing techniques. In second level architecture, statistical texture and moment invariant based features are extracted depending on the shape characteristics of the region. These features are used as input pattern to the Fuzzy Hypersphere Neural Network (FHSNN) classifier. The experimental result shows that proposed approach is superior in comparison with only statistical texture features in terms of recognition rate, training and testing time.
Key-Words / Index Term
Chest Radiography, Computer Tomography (CT), Fuzzy Hypersphere Neural Network (FHSNN), Lung Nodule, Gray level co-occurrence matrix (GLCM)
References
[1] M.N. Gurcan, B. Sahiner, N. Petrick, H. Chan, E.A. Kazerooni, P.N. Cascade, and L. Hadjiiski, �Lung Nodule Detection on Thoracic Computed Tomography Images: Preliminary Evaluation of a Computer-Aided Diagnosis System�, Medical Physics, Vol. 29, No. 11, pp. 2552- 2558, 2002.
[2] K. Kanazawa, Y. Kawata, N. Niki, H. Satoh, H. Ohmatsu, R. Kakinuma, M. Kaneko, N. Moriyama, and K. Eguchi, �Computer-Aided Diagnosis for Pulmonary Nodules Based on Helical CT Images�, Compute. Med. Image Graph, Vol. 22, No. 2, pp. 157-167, 1998.
[3] K. Kanazawa, M. Kubo, and N. Niki, �Computer Aided Diagnosis System for Lung Cancer Based on Helical CT Images,� International Conference on Pattern Recognition, pp. 381- 385, 1996.
[4] YSP Chiou, YMF Lure, PA Ligomenides, Neural networks image analysis and classification in hybrid lung nodule detection (HLND) system, in the Proceedings of the IEEE-SP workshop on Neural Networks for Signal Processing, 1993.
[5] Yamomoto, H. Jiang, M. Matsumoto, Y. Tateno, T. Iinuma, and T. Matsumoto, �Image Processing for Computer-Aided Diagnosis of Lung Cancer by CT (LSCT)�, IEEE Workshop on Applications of Computer Vision, pp. 236 � 241, 1996.
[6] Z.H. Zhou, Y. Jiang, Y.B. Yang and S.F. Chen, �Lung cancer cell identification based on artificial neural network ensembles,� Artificial Intelligence in Medicine, pp. 25 � 36, 2002.
[7] Y. Yeny, H. Helen and G.S. Yeong, �Hybrid Lung Segmentation in Chest CT Images for Computer-Aided Diagnosis�, International Workshop on Enterprise networking and Computing in Healthcare Industry, pp. 378 � 383, 2005.
[8] M.G. Penedo, M.J. Carreira, A. Mosquera, and D. Cabello, �Computer-Aided Diagnosis: A Neural-Network-Based Approach to Lung Nodule Detection�, IEEE Transactions on Medical Imaging, pp. 872 � 880, 1998.
[9] D. Lin and C. Yan, �Lung Nodules Identification Rules Extraction with Neural Fuzzy Network�, IEEE Processing on Neural Information, Vol. 4, pp. 2049- 2053, 2002.
[10] P.K. Simpson, �Fuzzy min-max neural networks-Part 1:Classification�, IEEE Trans. neural networks, Vol. 3, No. 5, pp. 776 � 786, 1992.
[11] P.K. Simpson, �Fuzzy min-max neural networks-Part 2:Clustering�, IEEE Trans. fuzzy systems, Vol. 1, No. 1, pp. 32 � 45, 1993.
[12] U.V. Kulkarni and T.R. Sontakke, �Fuzzy Hypersphere Neural Network Classifier�, In 10th Int. IEEE Conference on fuzzy systems, University of Melbourne, Australia, 2001.
[13] U.V. Kulkarni, D.D. Doye and T.R. Sontakke, �General fuzzy hypersphere neural network�, IEEE, IJCNN, pp. 2369 � 2374, 2002.
[14] D. Mundada,A. Murade, O. Vaidya and Swathi J.N., �Software fault prediction using artificial neural network and resilient back Propagation,� International Journal on Computer Science and Engineering (IJCSE), Vol. 5, No.03, pp. 2319-7323, 2016.
[15] R.C. Gonzalez, R.E. Woods and S.L. Eddins. �Digital Image Processing�, Addison-Wesley, M. Young, The Technical Writer�s Handbook. Mill Valley, CA: University Science, 2002.
[16] Japanese Society of Radiological Technology, (JSRT), http://www.jsrt.or.jp