DWT Based PCA and K-Means Clustering Block Level Approach for SAR Image De-Noising
D. Tripathi1 , V.K. Shukla2
Section:Research Paper, Product Type: Journal Paper
Volume-4 ,
Issue-12 , Page no. 87-91, Dec-2016
Online published on Jan 02, 2016
Copyright © D. Tripathi, V.K. Shukla . This is an open access article distributed under the Creative Commons Attribution License, which permits unrestricted use, distribution, and reproduction in any medium, provided the original work is properly cited.
View this paper at Google Scholar | DPI Digital Library
How to Cite this Paper
- IEEE Citation
- MLA Citation
- APA Citation
- BibTex Citation
- RIS Citation
IEEE Citation
IEEE Style Citation: D. Tripathi, V.K. Shukla, “DWT Based PCA and K-Means Clustering Block Level Approach for SAR Image De-Noising,” International Journal of Computer Sciences and Engineering, Vol.4, Issue.12, pp.87-91, 2016.
MLA Citation
MLA Style Citation: D. Tripathi, V.K. Shukla "DWT Based PCA and K-Means Clustering Block Level Approach for SAR Image De-Noising." International Journal of Computer Sciences and Engineering 4.12 (2016): 87-91.
APA Citation
APA Style Citation: D. Tripathi, V.K. Shukla, (2016). DWT Based PCA and K-Means Clustering Block Level Approach for SAR Image De-Noising. International Journal of Computer Sciences and Engineering, 4(12), 87-91.
BibTex Citation
BibTex Style Citation:
@article{Tripathi_2016,
author = {D. Tripathi, V.K. Shukla},
title = {DWT Based PCA and K-Means Clustering Block Level Approach for SAR Image De-Noising},
journal = {International Journal of Computer Sciences and Engineering},
issue_date = {12 2016},
volume = {4},
Issue = {12},
month = {12},
year = {2016},
issn = {2347-2693},
pages = {87-91},
url = {https://www.ijcseonline.org/full_paper_view.php?paper_id=1138},
publisher = {IJCSE, Indore, INDIA},
}
RIS Citation
RIS Style Citation:
TY - JOUR
UR - https://www.ijcseonline.org/full_paper_view.php?paper_id=1138
TI - DWT Based PCA and K-Means Clustering Block Level Approach for SAR Image De-Noising
T2 - International Journal of Computer Sciences and Engineering
AU - D. Tripathi, V.K. Shukla
PY - 2016
DA - 2017/01/02
PB - IJCSE, Indore, INDIA
SP - 87-91
IS - 12
VL - 4
SN - 2347-2693
ER -
![]() |
![]() |
![]() |
1551 | 1371 downloads | 1336 downloads |
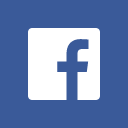
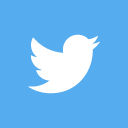
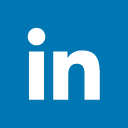
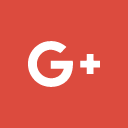
Abstract
Visual data are transmitted as the high quality digital images in the major fields of communication in all of the modern applications. These images on receiving after transmission are most of the times corrupted with noise. This thesis focused on the work which works on the received image processing before it is used for particular applications. We applied image denoising which involves the manipulation of the DWT coefficients of noisy image data to produce a visually high standard denoised image. This works consist of extensive reviews of the various parametric and non parametric existing denoising algorithms based on statistical estimation approach related to wavelet transforms connected processing approach and contains analytical results of denoising under the effect of various noises at different intensities .These different noise models includes additive and multiplicative type�s distortions in images used. It includes Gaussian noise and speckle noise. The denoising algorithm is application independent and giving a very high speed performance with desired noise less image even in the presence of high level distortion. Hence, it is not required to have prior knowledge about the type of noise present in the image because of the adaptive nature of the proposed denoising algorithm.
Key-Words / Index Term
Image - denoising, DWT, Gaussian noise , PCA ,K mean clustering
References
[1] Z. Cai, T. H. Cheng, C. Lu, and K. R. Subramanian, �Efficient waveletbased image denoising algorithm,� Electron. Lett., vol. 37, no. 11, pp. 683�685, May 2001.
[2] S. Chang, B. Yu, and M. Vetterli, �Adaptive wavelet thresholding for image denoising and compression,� IEEE Trans. Image Processing, vol. 9, pp. 1532�1546, Sept. 2000.
[3] F. Luisier, T. Blu, and M. Unser, �A new SURE approach to image denoising: Interscale orthonormal wavelet thresholding,� IEEE Trans. Image Process., vol. 16, pp. 593-606, 2007.
[4] T. Cai and B.W. Silverman, �Incorporating information on neighboring coefficients into wavelet estimation,� Sankhya, vol. 63, pp. 127�148, 2001.
[5] M. S. Crouse, R. D. Nowak, and R. G. Baraniuk, �Wavelet-based signal processing using hidden Markov models,� IEEE Trans. Signal Processing, vol. 46, pp. 886�902, Apr. 1998.
[6] D. L. Donoho and I. M. Johnstone, �Ideal spatial adaptation by wavelet shrinkage,� Biometrika, vol. 81, no. 3, pp. 425�455, 1994.
[7] H. Rabbani, �Image denoising in steerable pyramid domain based on a local Laplace prior,� Pattern Recognition, vol. 42, pp. 2181-2193, 2009.
[8] A. Achim and E. E. Kuruoglu, �Image denoising using bivariate α-stable distributions in the complex wavelet domain,� IEEE Signal Process. Lett., vol. 12, pp. 17-20, 2005.
[9] A. Pizurica and W. Philips, �Estimating the probability of the presence of a signal of interest in multi resolution single- and multiband image denoising,� IEEE Trans. Image Process., vol. 15, pp. 654-665, 2006.
[10] M. K. Mihcak, I.Kozintsev, K. Ramchandran, and P. Moulin, �Low-complexity image denoising based on statistical modeling of wavelet coefficients,� IEEE Signal Processing Lett., vol. 6, pp. 300�303, Dec. 1999.
[11] Q. Guo and S. Yu, �Image denoising using a multivariate shrinkage function in the curvelet domain,� IEICE Electron. Express, vol. 7, pp. 126-131, 2010.
[12] L. Sendur and I. W. Selesnick, �A bivariate shrinkage function for wavelet based denoising,� in IEEE ICASSP, 2002.