Cross-Domain Sentiment Classification Using SST
Megha P.K.1 , Hima K.G.2
- Dept of CSE, Royal College of Engineering and Technology, Thrissur, India.
- Dept of CSE, Royal College of Engineering and Technology, Thrissur, India.
Correspondence should be addressed to: meghalighi@gmail.com .
Section:Review Paper, Product Type: Journal Paper
Volume-5 ,
Issue-6 , Page no. 249-252, Jun-2017
Online published on Jun 30, 2017
Copyright © Megha P.K., Hima K.G. . This is an open access article distributed under the Creative Commons Attribution License, which permits unrestricted use, distribution, and reproduction in any medium, provided the original work is properly cited.
View this paper at Google Scholar | DPI Digital Library
How to Cite this Paper
- IEEE Citation
- MLA Citation
- APA Citation
- BibTex Citation
- RIS Citation
IEEE Style Citation: Megha P.K., Hima K.G., “Cross-Domain Sentiment Classification Using SST,” International Journal of Computer Sciences and Engineering, Vol.5, Issue.6, pp.249-252, 2017.
MLA Style Citation: Megha P.K., Hima K.G. "Cross-Domain Sentiment Classification Using SST." International Journal of Computer Sciences and Engineering 5.6 (2017): 249-252.
APA Style Citation: Megha P.K., Hima K.G., (2017). Cross-Domain Sentiment Classification Using SST. International Journal of Computer Sciences and Engineering, 5(6), 249-252.
BibTex Style Citation:
@article{P.K._2017,
author = {Megha P.K., Hima K.G.},
title = {Cross-Domain Sentiment Classification Using SST},
journal = {International Journal of Computer Sciences and Engineering},
issue_date = {6 2017},
volume = {5},
Issue = {6},
month = {6},
year = {2017},
issn = {2347-2693},
pages = {249-252},
url = {https://www.ijcseonline.org/full_paper_view.php?paper_id=1335},
publisher = {IJCSE, Indore, INDIA},
}
RIS Style Citation:
TY - JOUR
UR - https://www.ijcseonline.org/full_paper_view.php?paper_id=1335
TI - Cross-Domain Sentiment Classification Using SST
T2 - International Journal of Computer Sciences and Engineering
AU - Megha P.K., Hima K.G.
PY - 2017
DA - 2017/06/30
PB - IJCSE, Indore, INDIA
SP - 249-252
IS - 6
VL - 5
SN - 2347-2693
ER -
![]() |
![]() |
![]() |
594 | 425 downloads | 576 downloads |
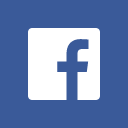
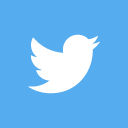
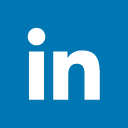
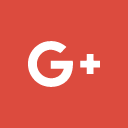
Abstract
Sentiment analysis refers to the use of natural language processing and machine learning techniques to identify and extract subjective information in a source material like product reviews. Due to revolutionary development in web technology and social media reviews can span so many different domains that it is difficult to gather annotated training data for all of them. Across domain sentiment analysis invokes adaptation of learned information of some (labeled) source domain to unlabelled target domain. The method proposed in this project uses an automatically created sentiment sensitive thesaurus(SST) for domain adaptation. Based on the survey conducted on related literature, we identified L1 regularized logistic regression is a good binary classifier for our area of interest.This makes our project more accurate in sentiment classification.We can use this as an application for product reviews. In addition to the previous work we propose the use of senti wordnet and adjective adverb combinations for those effective feature learning.
Key-Words / Index Term
Sentiment Analysis, SST
References
[1] Andrea Esuli , Fabrizio Sebastiani, ” SENTIWORDNET: A Pub-licly Available Lexical Resource for Opinion Mining”, Proc. of the 5th Conf. on Language Resources and Evaluation (LREC06), 2006.
[2] Bo Pang, Lillian Lee, and Shivakumar Vaithyanathan.”Thumbs up? sentiment classification using machine learning techniques”. In EMNLP 2002, pages 79-86.
[3] Danushka Bolegalla ,David Weir, John Caroll ”Using Multiple Sources to Construct a Sentiment Sensitive Thesaurus for Cross-Domain Sentiment Classification”.
[4] John Blitzer, Ryan McDonald, and Fernando Pereira.”Domain adaptation with structural correspondence learning”. In EMNLP 2006.
[5] Farah Benamara, Sabatier Irit, Carmine Cesarano, Napoli Fed-erico, Diego Reforgiato, ” Sentiment Analysis: Adjectives and Adverbs are better than Adjectives Alone ”, In Proc of Int Conf on Weblogs and Social Media , 2007.