Comparison of Structure Based Models for Handwritten English Character Recognition
Bhavana Shastry M.1 , Pradeep N.2
- Dept. of CSE, Bapuji Institute of Engineering and Technology, Davanagere, India.
- Dept. of CSE, Bapuji Institute of Engineering and Technology, Davanagere, India.
Correspondence should be addressed to: bhavanashastry13@gmail.com.
Section:Research Paper, Product Type: Journal Paper
Volume-5 ,
Issue-8 , Page no. 126-130, Aug-2017
CrossRef-DOI: https://doi.org/10.26438/ijcse/v5i8.126130
Online published on Aug 30, 2017
Copyright © Bhavana Shastry M., Pradeep N. . This is an open access article distributed under the Creative Commons Attribution License, which permits unrestricted use, distribution, and reproduction in any medium, provided the original work is properly cited.
View this paper at Google Scholar | DPI Digital Library
How to Cite this Paper
- IEEE Citation
- MLA Citation
- APA Citation
- BibTex Citation
- RIS Citation
IEEE Style Citation: Bhavana Shastry M., Pradeep N., “Comparison of Structure Based Models for Handwritten English Character Recognition,” International Journal of Computer Sciences and Engineering, Vol.5, Issue.8, pp.126-130, 2017.
MLA Style Citation: Bhavana Shastry M., Pradeep N. "Comparison of Structure Based Models for Handwritten English Character Recognition." International Journal of Computer Sciences and Engineering 5.8 (2017): 126-130.
APA Style Citation: Bhavana Shastry M., Pradeep N., (2017). Comparison of Structure Based Models for Handwritten English Character Recognition. International Journal of Computer Sciences and Engineering, 5(8), 126-130.
BibTex Style Citation:
@article{M._2017,
author = {Bhavana Shastry M., Pradeep N.},
title = {Comparison of Structure Based Models for Handwritten English Character Recognition},
journal = {International Journal of Computer Sciences and Engineering},
issue_date = {8 2017},
volume = {5},
Issue = {8},
month = {8},
year = {2017},
issn = {2347-2693},
pages = {126-130},
url = {https://www.ijcseonline.org/full_paper_view.php?paper_id=1400},
doi = {https://doi.org/10.26438/ijcse/v5i8.126130}
publisher = {IJCSE, Indore, INDIA},
}
RIS Style Citation:
TY - JOUR
DO = {https://doi.org/10.26438/ijcse/v5i8.126130}
UR - https://www.ijcseonline.org/full_paper_view.php?paper_id=1400
TI - Comparison of Structure Based Models for Handwritten English Character Recognition
T2 - International Journal of Computer Sciences and Engineering
AU - Bhavana Shastry M., Pradeep N.
PY - 2017
DA - 2017/08/30
PB - IJCSE, Indore, INDIA
SP - 126-130
IS - 8
VL - 5
SN - 2347-2693
ER -
![]() |
![]() |
![]() |
695 | 446 downloads | 622 downloads |
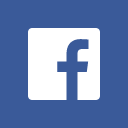
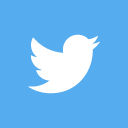
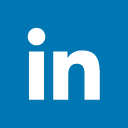
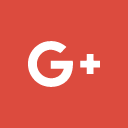
Abstract
Characters are the symbols made by man that are composed of different structure and strokes for easy communication. The intrinsic characteristics of the characters can be utilized to design the stroke and structure based models for handwritten character recognition. This paper focus to learn the part based and the stroke detector based models to recognize the characters by detecting the elastic strokes. The Tree Structured Model (TSM) and the Mixture of parts Tree Structured Model (MTSM) are the part based models that uses the trained part models on the images to recognize the characters. These models require manually labelled key points. In order to learn the discriminative stroke detectors automatically, the discriminative spatiality embedded dictionary learning-based representation (DSEDR) is used for character recognition. A comparative study is made on all the three models on the chars74k dataset to determine the model that shows the best performance.
Key-Words / Index Term
Character recognition, stroke detector, codewords, spatially embedded dictionary, part based model.
References
[1] Cun-Zhao Shi, Song Gao, Meng-Tao Liu, Cheng-Zuo Qi, Chun-Heng Wang, "Stroke detector and structure based models for Character Recognition: A Comparative Study" in IEEE transactions on image processing, volume 24, no. 12, Dec 2015.
[2] C. Yao, X. Bai, B. Shi, and W. Liu, “Strokelets: A learned multi-scale representation for scene text recognition,” in the Proceedings CVPR, Jun. 2014, pp. 4042–4049.
[3] S. Gao, C. Wang, B. Xiao, C. Shi, and Z. Zhang, “Stroke bank: A high level representation for scene character recognition,” in the Proceedings on 22nd International Conerence Pattern Recognition (ICPR), Aug. 2014, pp. 2909–2913.
[4] C. Shi, C. Wang, B. Xiao, Y. Zhang, S. Gao, and Z. Zhang, “Scene text recognition using part-based tree-structured character detection,” In the Proceedings of IEEE Conference Computer Vision and Pattern Recognition (CVPR), Jun. 2013, pp. 2961–2968.
[5] T. E. de Campos, B. R. Babu, and M. Varma, “Character recognition in natural images,” In the Proceedings of VISAPP, 2013, pp. 273–280.
[6] S. Tian, S. Lu, B. Su, and C. L. Tan, “Scene text recognition using co-occurrence of histogram of oriented gradients,” in the Proceedings on 12th International Conference Document Analytical Recognition. (ICDAR), Aug. 2013, pp. 912–916.
[7] L. Wang, M. Zeiler, S. Zhang, Y. L. Cun, and R. Fergus, “Regularization of Neural Networks using drop connect,” in Proceedings 30th International Conference Machine Learning (ICML), 2013, pp. 1058–1066.
[8] X. Zhu and D. Ramanan, “Face detection, pose estimation, and landmark
localization in the wild,” in Proceedings CVPR, Jun. 2011, pp. 2879–2886.
[9] D. L. Smith, J. Field, and E. Learned-Miller, “Enforcing similarity constraints with integer programming for better scene text recognition,” in Proceedings CVPR, Jun. 2011, pp. 73–80.
[10] L. Neumann and J. Matas, “A method for text localization and recognition in real-world images,” in Proceedings Asian Conference Computer Vision, 2011, pp. 770–783.
[11] A. Bissacco, M. Cummins, Y. Netzer, and H. Neven, “PhotoOCR: Reading text in uncontrolled conditions,” in Proceedings IEEE International Conference Computer Vision, Dec. 2011, pp. 785–792.
[ 12] Y. Yang and D. Ramanan, “Articulated pose estimation with flexible mixtures-of- parts,” in Proceedings CVPR, Jun. 2011, pp. 1385–1392.
[13] T. E. de Campos, B. R. Babu, and M. Varma, “Character recognition in natural images,” in Proceedings VISAPP, 2009, pp. 273–280.
[14] C. Yi, X. Yang, and Y. Tian, “Feature representations for scene text character recognition: A comparative study,” in Proceedings IEEE 12th International Conference Document Analytics Recognition Aug. 2011, pp. 907–911.
[15] T. Wang, D. J. Wu, A. Coates, and A. Y. Ng, “End-to-end text recognition with convolutional neural networks,” in Proceedings 21st International Conference Pattern Recognition (ICPR), Nov. 2012, pp. 3304–3308.
[16] P. F. Felzenszwalb and D. P. Huttenlocher, “Pictorial structures for object recognition” in Proceedings Computer Vision., vol. 61, no. 1, pp. 55–79, Jan. 2005.
[17] N. Dalal and B. Triggs, “Histograms of oriented gradients for human detection,” in Proceedings CVPR, vol. 1. Jun. 2005, pp. 886–893.
[18] S. M. Lucas, A. Panaretos, L. Sosa, A. Tang, S. Wong, and R. Young, “ICDAR 2003 robust reading competitions,” in Proceedings ICDAR, vol. 2. Aug. 2003, pp. 682–687.
[19] C.-L. Liu, K. Nakashima, H. Sako, and H. Fujisawa, “Handwritten digit recognition: Benchmarking of state-of-the-art techniques,” Pattern Recognition., volume 36, no. 10, pp. 2271–2285, Oct. 2003.