A Comparative Review of Various Approaches for Skin Cancer Detection
R. Maurya1 , A. Singh2 , V. Srivastava3 , R. Yadav4
- Computer Science and Engineering, Shri Ramswaroop Memorial University, Barabanki, India.
- Computer Science and Engineering, Shri Ramswaroop Memorial University, Barabanki, India.
- Computer Science and Engineering, Shri Ramswaroop Memorial University, Barabanki, India.
- Computer Science and Engineering, Shri Ramswaroop Memorial University, Barabanki, India.
Correspondence should be addressed to: maurya123ritesh47@gmail.com.
Section:Review Paper, Product Type: Journal Paper
Volume-5 ,
Issue-10 , Page no. 146-152, Oct-2017
CrossRef-DOI: https://doi.org/10.26438/ijcse/v5i10.146152
Online published on Oct 30, 2017
Copyright © R. Maurya, A. Singh, V. Srivastava, R. Yadav . This is an open access article distributed under the Creative Commons Attribution License, which permits unrestricted use, distribution, and reproduction in any medium, provided the original work is properly cited.
View this paper at Google Scholar | DPI Digital Library
How to Cite this Paper
- IEEE Citation
- MLA Citation
- APA Citation
- BibTex Citation
- RIS Citation
IEEE Style Citation: R. Maurya, A. Singh, V. Srivastava, R. Yadav, “A Comparative Review of Various Approaches for Skin Cancer Detection,” International Journal of Computer Sciences and Engineering, Vol.5, Issue.10, pp.146-152, 2017.
MLA Style Citation: R. Maurya, A. Singh, V. Srivastava, R. Yadav "A Comparative Review of Various Approaches for Skin Cancer Detection." International Journal of Computer Sciences and Engineering 5.10 (2017): 146-152.
APA Style Citation: R. Maurya, A. Singh, V. Srivastava, R. Yadav, (2017). A Comparative Review of Various Approaches for Skin Cancer Detection. International Journal of Computer Sciences and Engineering, 5(10), 146-152.
BibTex Style Citation:
@article{Maurya_2017,
author = {R. Maurya, A. Singh, V. Srivastava, R. Yadav},
title = {A Comparative Review of Various Approaches for Skin Cancer Detection},
journal = {International Journal of Computer Sciences and Engineering},
issue_date = {10 2017},
volume = {5},
Issue = {10},
month = {10},
year = {2017},
issn = {2347-2693},
pages = {146-152},
url = {https://www.ijcseonline.org/full_paper_view.php?paper_id=1491},
doi = {https://doi.org/10.26438/ijcse/v5i10.146152}
publisher = {IJCSE, Indore, INDIA},
}
RIS Style Citation:
TY - JOUR
DO = {https://doi.org/10.26438/ijcse/v5i10.146152}
UR - https://www.ijcseonline.org/full_paper_view.php?paper_id=1491
TI - A Comparative Review of Various Approaches for Skin Cancer Detection
T2 - International Journal of Computer Sciences and Engineering
AU - R. Maurya, A. Singh, V. Srivastava, R. Yadav
PY - 2017
DA - 2017/10/30
PB - IJCSE, Indore, INDIA
SP - 146-152
IS - 10
VL - 5
SN - 2347-2693
ER -
![]() |
![]() |
![]() |
898 | 454 downloads | 314 downloads |
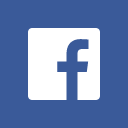
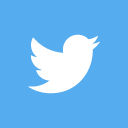
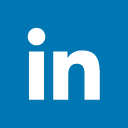
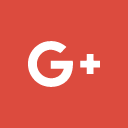
Abstract
Classification of skin cancer gives the best chance of being diagnosed early. Biopsy method for skin cancer detection is much painful. Human interpretation contains difficulty and subjectivity therefore automated analysis of skin cancer affected images has become important. This paper proposes an automatic medical image classification method to classify two major type skin cancers: Melanoma and Non-melanoma. In this paper, we have used the color and texture features in combination which give better results than using color or gray level information alone. We have used k-means clustering algorithm to segment the lesion. The features are extracted by four different color-texture feature extractors from the segmented images. DullRazor technique is used to eliminate surrounding hairs. Classification accuracy of our proposed system is evaluated on four different types of classifiers and their values are compared with one another. The results of the proposed system are computed on five different classification rates in order to perform better analysis of our proposed system. SVM outperforms among all other classifiers with accuracy of 76.69%.
Key-Words / Index Term
Grey Level Co-occurrence Matrices, Support Vector Ma-chine, Local Binary Patterns, Texture features, color percentiles, K-means clustering, Cooccurence Matrix, Color Features, Integrative Cooccurence Matrix, Gabor Features, Linear Classifier, NN Classifiers, NMC Classifiers, Cross-validation.
References
[1] E. Linos, S.M. Swetter, M.G. Cockburn, G.A. Colditz, and C.A. Clarke, “Increasing burden of melanoma in the United States,” Journal of Investigative Dermatology, vol. 129, no. 7, pp. 1666– 1674, 2009.
[2] J. Mayer, “Systematic review of the diagnostic accuracy of dermatoscopy in detecting malignant melanoma,” Medical Journal of Australia, vol. 167, no. 4, pp. 206–210, 1997.
[3] J. K. Robinson and R. Turrisi, “Skills training to learn discrimination of ABCDE criteria by those at risk of developing melanoma,” Archives of Dermatology, vol. 142, no. 4, pp. 447– 452, 2006.
[4] M. E. Celebi, H. Iyatomi, G. Schaefer, and W.V. Stoecker, “Lesion Border Detection in Dermoscopy Images," Computerized Medical Imaging and Graphics, vol. 33, no. 2, pp. 148-153, 2009.
[5] H. Iyatomi, et al., “An Improved Internet-based Melanoma Screening System with Dermatologist-like Tumor Area Extraction Algorithm," Computerized Medical Imaging and Graphics, vol. 32, no. 7, pp. 566-579, 2008.
[6] R. Garnavi, M. Aldeen, M.E. Celebi, G. Varigos, and S. Finch, “Border Detection in Dermoscopy Images Using Hybrid Thresholding on Optimized Color Channels," Computerized Medical Imaging and Graphics, vol. 35, no. 2, pp. 105-115, 2011.
[7] H. Zhou, et al., “Spatially Constrained Seg- mentation of Dermoscopy Images," in Proceedings of the 5th IEEE International Symposium on Biomedical Imaging, pp. 800-803, 2008.
[8] P. Schmid, “Segmentation of Digitized Dermatoscopic Images by Two-Dimensional Color Clustering," IEEE Transactions on Medical Imaging, vol. 18, no. 2, pp. 164-171, 1999.
[9] H. Zhou, G. Schaefer, A. Sadka, and M. E. Celebi, “Anisotropic Mean Shift Based Fuzzy C-Means Segmentation of Dermoscopy Images," IEEE Journal of Selected Topics in Signal Processing, vol. 3, no. 1, pp. 26-34, 2009.
[10] M. Mete, S. Kockara, and K. Aydin, “Fast Density-Based Lesion Detection in Der-moscopy Images," Computerized Medical Imaging and Graphics, vol. 35, no. 2, pp. 128-136, 2011.
[11] R. Melli, C. Grana, and R. Cucchiara, “Comparison of Color Clustering Algorithms for Segmentation of Dermatological Images," in Proceedings of the SPIE Medical Imaging Conference, pp. 1211-1219, 2006.
[12] B. Erkol, R. H. Moss, R. J. Stanley, W. V. Stoecker, and E. Hvatum, “Automatic Lesion Boundary Detection in Dermoscopy Images Using Gradient Vector Flow Snakes," Skin Research and Technology, vol. 11, no. 1, pp. 17-26, 2005.
[13] H. Zhou, et al., “Gradient Vector Flow with Mean Shift for Skin Lesion Segmentation," Computerized Medical Imaging and Graphics, vol. 35, no. 2, pp. 121-127, 2011.
[14] Q. Abbas, M. E. Celebi, and I. F. Garcia, “A Novel Perceptually-Oriented Approach for Skin Tumor Segmentation," International Journal of Innovative Computing, In-formation and Control, vol. 8, no. 3, pp. 1837-1848, 2012.
[15] M. E. Celebi, et al., “Unsupervised Border Detection in Dermoscopy Images," Skin Research and Technology, vol. 13, no. 4, pp. 454-462, 2007.
[16] M. E. Celebi, et al., “Border detection in Dermoscopy Images Using Statistical Region Merging”, Kins Research and Technology, vol. 14,no. 3, pp. 347-353, 2008.
[17] T. Wang, et al., “Modified Watershed Technique and Post-Processing for Segmentation of Skin Lesions in Dermoscopy Images," Computerized Medical Imaging and Graphics, vol. 35, no. 2, pp. 116-120, 2011.
[18] Q. Abbas, M. E. Celebi, I. F. Garcia, and M. Rashid, ”Lesion Border Detection in Dermoscopy Images Using Dynamic Programming," Skin Research and Technology, vol. 17, no. 1, pp. 91-100, 2011.
[19] Q. Abbas, M. E. Celebi, and I. F. Garcia, “Skin Tumor Area Extraction Using an Improved Dynamic Programming Approach," Skin Research and Technology, vol. 18, no. 2, pp. 133-142, 2012.
[20] G. Schaefer, M.I. Rajab, M.E. Celebi, and H. Iyatomi, “Colour and Contrast En-hancement for Improved Skin Lesion Segmentation," Computerized Medical Imag-ing and Graphics, vol. 35, no. 2, pp. 99-104, 2011.
[21] P. Wighton, T. K. Lee, H. Lui, D. I. McLean, and M. S. Atkins, “Generalizing Common Tasks in Automated Skin Lesion Diagnosis," IEEE Transactions on Infor-mation Technology in Biomedicine, vol. 15, no. 4, pp. 622-629, 2011.
[22] V. K. Dehariya, S. K. Shrivastava, R. C. Jain,“Clustering of Image Data Set Using K-Means and Fuzzy K-Means Algorithms ” , International conference on CICN, pp. 386- 391, 2010.
[23] Vijay Jumb, Mandar Sohani, Avinash Shrivas, “Color Image Segmentation Using K-Means Clustering and Otsu’s Adaptive Thresholding,” International Journal of Innovative Technology and Exploring Engineering (IJITEE), February 2014.
[24] J. F. Aitken, et al., “Reliability of computer image analysis of Pigmented skin lesions of Australian adolescents,” Journal of Cancer, vol. 78, no. 2, pp. 252–257, 1996.
[25] A.Green, N. Martin, J. Pfitzner, M. O’Rourke, and N.Knight, “Computer image analysis in the diagnosis of melanoma,” Journal of the American Academy of Dermatology, vol. 31, no. 6, pp. 958–964, 1994.
[26] H. C. Lee, Skin Cancer Diagnosis Using Hierarchical Neural Networks and Fuzzy Logic, Department of Computer Science, University of Missouri, Rolla, Mo, USA, 1994.
[27] S. Seidenari, M. Burroni, G. Dell’Éva, P.Pepe, and B.Belletti, “Computerized evaluation of pigmented skin lesion images recorded by a videomicroscope: comparison between polarizing mode observation and oil/slide mode observation,” Skin Research and Technology, vol. 1, no. 4, pp. 187–191, 1995.
[28] K. Ramlakhan and Y. Shang, “A mobile automated skin lesion classification sys-tem,” in Proceedings of the 23rd IEEE International Conference on Tools with Artificial Intelligence (ICTAI ’11), pp. 138–141, November 2011.
[29] N. Cascinelli, et al., “Results obtained by using a com-puterized image analysis system designed as an aid to diagnosis of cutaneous melanoma,” Melanoma Research, vol. 2, no. 3, pp. 163–170, 1992.
[30] Francesco Bianconia, et al., “Automatic classification of granite tiles through colour and texture features”, Preprint submitted to Expert Systems with Applications, February 20, 2012.
[31] Ammara Masood and Adel Ali Al-Jumaily, “Computer Aided Diagnostic Support System for Skin Cancer: A Review of Techniques and Algorithms” Hindawi Pub-lishing Corporation International Journal of Biomedical Imaging, Volume 2013.
[32] T. Ojala, M. Pietikainen, and T. Maenpaa, "Multiresolution gray-scale and rotation invariant texture classification with Local Binary Patterns". IEEE Transactions on Pattern Analysis and Machine Intelligence, 24:971-987, 2002.
[33] Niskanen, M., Silven, O., Kauppinen, H., June 2001. “Color and texture based wood inspection with non-supervised clustering”, Proceedings of the 12th Scandivanian Conference on Image Analysis. Bergen, Norway, pp. 336–342, 2001 .
[34] Arvis, V., et al., Generalization of the cooccurrence matrix for colour images: application to colour texture classification. Image Analysis and Stereology 23 (3), 63–72, 2004.
[35] Palm, C., “Colour texture classification by integrative co-occurrence matrices”, Pat-tern Recognition 37 (5), 965–976, 2004.
[36] F. Bianconi and A. Fernandez., "Evaluation of the effects of Gabor filter parameters on texture classification". Pattern Recognition, 40:3325-3335, 2007.
[37] F. Bianconi, et al., Robust color texture features based on ranklets and discrete Fourier transform. Journal of Electronic Imaging, 18:043012-1-8, 2009.
[38] M. Masotti and R. Campanini., "Texture classification using invariant ranklet fea-tures", Pattern Recognition Letters, 29:1980-1986, 2008.
[39] Scholkopf, B., Smola, A., “Learning with Kernels: Support Vector Machines, Regularization, Optimization, and Beyond”, MIT Press, 2002.
[40] Hsu, et al., “A Practical Guide to Support Vector Classification”, Tech. rep., Department of Computer Science National Taiwan University, 2010.
[41] B.Gohila vani, A. Selvaraj, M. Vijayapriya, N.Anandh, “Segmentation and Classification of Skin Lesions Based on Texture Features”, Int. Journal of Engineering Research and Applications, December 2014
[42] R. Maurya ,S.K. Singh, A. K. Maurya, A. Kumar, "GLCM and Multi Class Support vector machine based automated skin cancer classification IEEE Con-ference", Page(s):444 - 447Print ISBN:978-93-80544-10-6 INSPEC Accession Num-ber:14382783, 5-7 March 2014