A Relative Study of Data Mining and Big Data on IoT `s Data Streams
Sallauddin Mohmmad1 , Syed Nawaz Pasha2 , Dadi Ramesh3 , Shabana 4
- Computer Science and Engineering, SR Engineering College, Warangal, India.
- Computer Science and Engineering, SR Engineering College, Warangal, India.
- Computer Science and Engineering, SR Engineering College, Warangal, India.
- Computer Science and Engineering, SR Engineering College, Warangal, India.
Correspondence should be addressed to: sallauddin.md@gmail.com.
Section:Research Paper, Product Type: Journal Paper
Volume-5 ,
Issue-10 , Page no. 200-209, Oct-2017
CrossRef-DOI: https://doi.org/10.26438/ijcse/v5i10.200209
Online published on Oct 30, 2017
Copyright © Sallauddin Mohmmad, Syed Nawaz Pasha, Dadi Ramesh ,Shabana . This is an open access article distributed under the Creative Commons Attribution License, which permits unrestricted use, distribution, and reproduction in any medium, provided the original work is properly cited.
View this paper at Google Scholar | DPI Digital Library
How to Cite this Paper
- IEEE Citation
- MLA Citation
- APA Citation
- BibTex Citation
- RIS Citation
IEEE Citation
IEEE Style Citation: Sallauddin Mohmmad, Syed Nawaz Pasha, Dadi Ramesh ,Shabana, “A Relative Study of Data Mining and Big Data on IoT `s Data Streams,” International Journal of Computer Sciences and Engineering, Vol.5, Issue.10, pp.200-209, 2017.
MLA Citation
MLA Style Citation: Sallauddin Mohmmad, Syed Nawaz Pasha, Dadi Ramesh ,Shabana "A Relative Study of Data Mining and Big Data on IoT `s Data Streams." International Journal of Computer Sciences and Engineering 5.10 (2017): 200-209.
APA Citation
APA Style Citation: Sallauddin Mohmmad, Syed Nawaz Pasha, Dadi Ramesh ,Shabana, (2017). A Relative Study of Data Mining and Big Data on IoT `s Data Streams. International Journal of Computer Sciences and Engineering, 5(10), 200-209.
BibTex Citation
BibTex Style Citation:
@article{Mohmmad_2017,
author = {Sallauddin Mohmmad, Syed Nawaz Pasha, Dadi Ramesh ,Shabana},
title = {A Relative Study of Data Mining and Big Data on IoT `s Data Streams},
journal = {International Journal of Computer Sciences and Engineering},
issue_date = {10 2017},
volume = {5},
Issue = {10},
month = {10},
year = {2017},
issn = {2347-2693},
pages = {200-209},
url = {https://www.ijcseonline.org/full_paper_view.php?paper_id=1499},
doi = {https://doi.org/10.26438/ijcse/v5i10.200209}
publisher = {IJCSE, Indore, INDIA},
}
RIS Citation
RIS Style Citation:
TY - JOUR
DO = {https://doi.org/10.26438/ijcse/v5i10.200209}
UR - https://www.ijcseonline.org/full_paper_view.php?paper_id=1499
TI - A Relative Study of Data Mining and Big Data on IoT `s Data Streams
T2 - International Journal of Computer Sciences and Engineering
AU - Sallauddin Mohmmad, Syed Nawaz Pasha, Dadi Ramesh ,Shabana
PY - 2017
DA - 2017/10/30
PB - IJCSE, Indore, INDIA
SP - 200-209
IS - 10
VL - 5
SN - 2347-2693
ER -
![]() |
![]() |
![]() |
1192 | 509 downloads | 334 downloads |
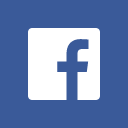
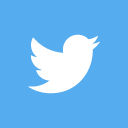
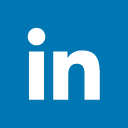
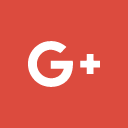
Abstract
The huge amount of data produced and captured by the Internet of Things (IoT) in current situations. IoT data created by several applications such as from smart cities, monitoring system, health care, object detecting systems and smart systems etc which all produce the continues data streams.But data of IoT recognized with RFID signal their size is near to 20 bytes. So that it is requiring of special procedures for analyzing, managing and mining the IoT stream data which is identified by RFID. Several software tools have been developed for mining the stream data relatively Big Data tools. While most current research looking into data mining and relative big data tools which are on machine learning of distributed and non-distributed systems with respect to IoT stream data.This paper focused on IoT data format, different kind stream data mining tools according to mining steps and few Big Data tools relative with data mining for handling the stream data of IoT.
Key-Words / Index Term
IoT, Data mining ,Big Data , RFID, Stream Data
References
[1] C.-W. Tsai, C.-F. Lai, and A. V. Vasilakos, “Future internet of things: open issues and challenges,” Wireless Networks, vol. 20, no. 8, pp. 2201–2217, 2014.
[2] A. Mukhopadhyay, U. Maulik, S. Bandyopadhyay, and C. A. C. Coello, “A survey of multiobjective evolutionary algorithms for data mining: part I,” IEEE Transactions on Evolutionary Computation, vol. 18, no. 1, pp. 4–19, 2014.
[3] J. Zhang, B. Iannucci, M. Hennessy, K. Gopal, S. Xiao,S. Kumar, D. Pfeffer, B. Aljedia, Y. Ren, M. Griss et al.,“Sensor Data as a Service–A Federated Platform for MobileData-centric Service Development and Sharing,” in ServicesComputing (SCC), 2013 IEEE International Conference on.IEEE, 2013, pp. 446–453.
[4] Y. Zhang, M. Chen, S. Mao, L. Hu, and V. Leung, “CAP: crowd activity prediction based on big data analysis,” IEEE Network, vol. 28, no. 4, pp. 52–57, 2014.
[5] L. Ramaswamy, V. Lawson, and S. Gogineni, “Towards a Quality-centric Big Data Architecture for Federated Sensor Services,” in Big Data (BigData Congress), 2013 IEEE International Congress on, June 2013, pp. 86–93.
[6] S. Haller, S. Karnouskos, and C. Schroth, “The Internet of Things in an enterprise context,” Future Internet Systems (FIS), LCNS, vol. 5468. Springer, 2008, pp. 14-8.
[7] Shahid Tufail, M. Abdul Qadeer, "Cloud Computing in Bioinformatics: Solution to Big Data Challenge", International Journal of Computer Sciences and Engineering, Vol.5, Issue.9, pp.232-236, 2017.
[8] M. Chen, S. Mao, Y. Zhang, and V. Leung, Big Data: Related Technologies, Challenges and Future Prospects, SpringerBriefs in Computer Science, Springer, 2014.
[9] J. Wan, D. Zhang, Y. Sun, K. Lin, C. Zou, and H. Cai, “VCMIA: a novel architecture for integrating vehicular cyber-physical systems and mobile cloud computing,” Mobile Networks and Applications, vol. 19, no. 2, pp. 153–160, 2014
[10] X. H. Rong, F.Chen, P. Deng, and S. L.Ma, “Alarge-scale device collaboration mechanism,” Journal of Computer Research and Development, vol. 48, no. 9, pp. 1589–1596, 2011.
[11] F. Chen, X.-H. Rong, P. Deng, and S.-L.Ma, “A survey of device collaboration technology and system software,” Acta Electronica Sinica, vol. 39, no. 2, pp. 440–447, 2011.
[12] L. Zhou, M. Chen, B. Zheng, and J. Cui, “Green multimedia communications over Internet of Things,” in Proceedings of the IEEE International Conference on Communications (ICC’12), pp. 1948–1952, Ottawa, Canada, June 2012.
[13] P. Deng, J. W. Zhang, X. H. Rong, and F. Chen, “A model of large-scale Device Collaboration system based on PI-Calculus for green communication,” Telecommunication Systems, vol. 52, no. 2, pp. 1313–1326, 2013.
[14] P. Deng, J. W. Zhang, X. H. Rong, and F. Chen, “Modeling the large-scale device control system based on PI-Calculus,” Advanced Science Letters, vol. 4, no. 6-7, pp. 2374–2379, 2011.
[15] J. Zhang, P. Deng, J. Wan, B. Yan, X. Rong, and F. Chen, “A novel multimedia device ability matching technique for ubiquitous computing environments,” EURASIP Journal on Wireless Communications and Networking, vol. 2013, no. 1, article 181, 12 pages, 2013.
[16] G. Kesavaraj and S. Sukumaran, “A study on classification techniques in data mining,” in Proceedings of the 4th International Conference on Computing, Communications and Networking Technologies (ICCCNT ’13), pp. 1–7, July 2013.
[17] S.Babu, G.Fathima , "Decision Trees for Mining Data Streams Based on the Gaussian Approximation", International Journal of Computer Sciences and Engineering, Vol.4, Issue.3, pp.35-38, 2016.
[18] Q. Jing, A. V. Vasilakos, J. Wan, J. Lu, and D. Qiu, “Security of the internet of things: perspectives and challenges,” Wireless Networks, vol. 20, no. 8, pp. 2481–2501, 2014.
[19] ] B. Chandra and P. P. Varghese, “Fuzzy SLIQ decision tree algorithm,” IEEE Transactions on Systems, Man, and Cybernetics, Part B: Cybernetics, vol. 38, no. 5, pp. 1294–1301, 2008.
[20] J. Shafer, R.Agrawal, and M.Mehta, “SPRINT: a scalable parallel classifier for data mining,” in Proceedings of 22nd International Conference on Very Large Data Bases, pp. 544–555, 1996.
[21] Chang, V., 2015. Towards a big data system disaster recovery in a Private cloud. Ad Hoc Networks, 000, pp.1–18.
[22] [23]Sakr, S. & Gaber, M.M., 2014. Large Scale and big data: Processing and Management Auerbach, ed., Schilling, D.R., 2014. Exaflop Computing Will Save the World ... If We Can Afford It - Industry Tap.
[23] González-Martínez, J. a. et al., 2015. cloud computing and education: A state-of-the-art survey. Computers & Education, 80, pp.132–151.
[24] D. Matolak: Channel Modeling for Vehicle-to-Vehicle Communications. IEEE Comm.Mag., pp. 76-83, May 2008
[25] Palaghat Yaswanth Sai, Pabolu Harika, "Illustration of IOT with Big Data Analytics", International Journal of Computer Sciences and Engineering, Vol.5, Issue.9, pp.221-223, 2017.
[26] R.S. Barga, J. Ekanayake, W. Lu, Project Daytona: Data Analytics as a Cloud Service, in: A. Kementsietsidis, M. A. V. Salles (Eds.), Proceedings of the International Conference of Data Engineering (ICDE 2012), IEEE Computer Society, 2012, pp. 1317–1320.
[27] Zhang, X., Wu, J., Yang, X., Ou, H., And Lv, T. 2009. A novel pattern extraction method for time series classification. Optimiz. Engin. 10, 2, 253–271.
[28] Zhong, S.,Khoshgoftaar, T., And Seliya, N. 2007. Clustering-Based network intrusion detection. Int. J. Reliab.Qual. Safety Engin. 14, 2, 169–187.
[29] Yankov, D., Keogh, E., And Rebbapragada, U. 2008. Disk aware discord discovery: Finding unusual time series in terabyte sized datasets. Knowl. Info. Syst. 17, 2, 241–262.
[30] Chhieng, V. And Wong, R. 2010. Adaptive distance measurement for time series databases. In Lecture Notes in Computer Science, vol. 4443. Springer, 598–610.
[31] Ouyang, R., Ren, L., Cheng, W., And Zhou, C. 2010. Similarity search and pattern discovery in hydrological time series data mining. Hydrol. Process. 24, 9, 1198–1210.
[32] Fuchs, E., Gruber, T., Pree, H., And Sick, B. 2010. Temporal data mining using shape space representations of time series. Neurocomput. 74, 1-3, 379–393.
[33] Assent, I., Wichterich, M., Krieger, R., Kremer, H., And Seidl, T. 2009. Anticipatory DTW for efficient similarity search in time series databases. Proc. VLDB Endowm. 2, 1, 826–837.
[34] Barone, P., Carfora, M., And March, R. 2009. Segmentation, classification and denoising of a time series field by A Variational Method. J. Math. Imag. Vis. 34, 2, 152–164.
[35] Gullo, F., Ponti, G., Tagarelli, A., And Greco, S. 2009. A time series representation model for accurate and fast similarity detection. Pattern Recogn. 42, 11, 2998–3014.
[36] Reeves, G., Liu, J., Nath, S., And Zhao, F. 2009. Managing massive time series streams with multi-scale compressed trickles. Proc. VLDB Endow. 2, 1, 97–108.
[37] Thu Vu, G. De Francisci Morales, J. Gama, and A. Bifet, “Distributed Adaptive Model Rules for Mining Big Data Streams,” in BigData ’14: Second IEEE International Conference on Big Data, 2014.
[38] J. Gama, I. Zliobaite, A. Bifet, M. Pechenizkiy, and A. Bouchachia, “A Survey on Concept Drift Adaptation,” ACM Computing Surveys, vol. 46, no. 4, 2014.
[39] A. Bifet, G. Holmes, R. Kirkby, and B. Pfahringer, “MOA: Massive Online Analysis,” The Journal of Machine Learning Research, vol. 11, pp. 1601–1604, 2010.
[40] Charu C. Aggarwal, Jiawei Han, Jianyong Wang, and Philip S. Yu. A framework for clustering evolving data streams. In VLDB ’03: 29th International Conference on Very Large Data Bases, pages 81–92, 2003.
[41] D. Schall, M. Aiello, and S. Dustdar, "Web services on embedded devices," International Journal of Web Information Systems, vol. 2, no. I, pp. 45-50, 2006.
[42] L. Johnsrud, D. Hadzic, T. Hafsoe, F. T. Johnsen, and K. Lund, "Efficient Web Services in Mobile Networks;` in Proceedings of 2008 Sixth European Conference on Web Services. IEEE, Nov. 200S, pp. 197-204.
[43] N. Priyantha, A. Kansal, M. Goraczko, and F. Zhao, "Tiny web services: design and implementation of interoperable and evolvable sensor networks," in Proceedings of the 6th ACM conference on Embedded network sensor systems. ACM, 200S, pp. 253-266.
[44] S. Nastic, S. Sehic, M. Vögler, H.-L. Truong, and S. Dustdar, “PatRICIA -- A Novel Programming Model for IoT Applications on Cloud Platforms,” 2013 IEEE 6th International Conference on Service-Oriented Computing and Applications, 2013, pp. 53–60.