An Extensive Investigate the MapReduce Technology
Yusuf Perwej1 , Md. Husamuddin2 , Fokrul Alom Mazarbhuiya3
- Department of Information Technology, Al Baha University, Al Baha, Kingdom of Saudi Arabia(KSA).
- Department of Information Technology, Al Baha University, Al Baha, Kingdom of Saudi Arabia(KSA).
- Department of Information Technology, Al Baha University, Al Baha, Kingdom of Saudi Arabia(KSA).
Correspondence should be addressed to: yusufperwej@gmail.com.
Section:Review Paper, Product Type: Journal Paper
Volume-5 ,
Issue-10 , Page no. 218-225, Oct-2017
CrossRef-DOI: https://doi.org/10.26438/ijcse/v5i10.218225
Online published on Oct 30, 2017
Copyright © Yusuf Perwej, Md. Husamuddin, Fokrul Alom Mazarbhuiya . This is an open access article distributed under the Creative Commons Attribution License, which permits unrestricted use, distribution, and reproduction in any medium, provided the original work is properly cited.
View this paper at Google Scholar | DPI Digital Library
How to Cite this Paper
- IEEE Citation
- MLA Citation
- APA Citation
- BibTex Citation
- RIS Citation
IEEE Citation
IEEE Style Citation: Yusuf Perwej, Md. Husamuddin, Fokrul Alom Mazarbhuiya, “An Extensive Investigate the MapReduce Technology,” International Journal of Computer Sciences and Engineering, Vol.5, Issue.10, pp.218-225, 2017.
MLA Citation
MLA Style Citation: Yusuf Perwej, Md. Husamuddin, Fokrul Alom Mazarbhuiya "An Extensive Investigate the MapReduce Technology." International Journal of Computer Sciences and Engineering 5.10 (2017): 218-225.
APA Citation
APA Style Citation: Yusuf Perwej, Md. Husamuddin, Fokrul Alom Mazarbhuiya, (2017). An Extensive Investigate the MapReduce Technology. International Journal of Computer Sciences and Engineering, 5(10), 218-225.
BibTex Citation
BibTex Style Citation:
@article{Perwej_2017,
author = {Yusuf Perwej, Md. Husamuddin, Fokrul Alom Mazarbhuiya},
title = {An Extensive Investigate the MapReduce Technology},
journal = {International Journal of Computer Sciences and Engineering},
issue_date = {10 2017},
volume = {5},
Issue = {10},
month = {10},
year = {2017},
issn = {2347-2693},
pages = {218-225},
url = {https://www.ijcseonline.org/full_paper_view.php?paper_id=1501},
doi = {https://doi.org/10.26438/ijcse/v5i10.218225}
publisher = {IJCSE, Indore, INDIA},
}
RIS Citation
RIS Style Citation:
TY - JOUR
DO = {https://doi.org/10.26438/ijcse/v5i10.218225}
UR - https://www.ijcseonline.org/full_paper_view.php?paper_id=1501
TI - An Extensive Investigate the MapReduce Technology
T2 - International Journal of Computer Sciences and Engineering
AU - Yusuf Perwej, Md. Husamuddin, Fokrul Alom Mazarbhuiya
PY - 2017
DA - 2017/10/30
PB - IJCSE, Indore, INDIA
SP - 218-225
IS - 10
VL - 5
SN - 2347-2693
ER -
![]() |
![]() |
![]() |
696 | 493 downloads | 322 downloads |
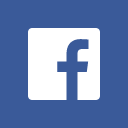
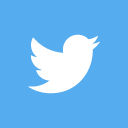
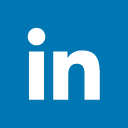
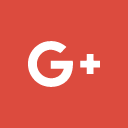
Abstract
Since, the last three or four years, the field of “big data” has appeared as the new frontier in the wide spectrum of IT-enabled innovations and favorable time allowed by the information revolution. Today, there is a raise necessity to analyses very huge datasets, that have been coined big data, and in need of uniqueness storage and processing infrastructures. MapReduce is a programming model the goal of processing big data in a parallel and distributed manner. In MapReduce, the client describes a map function that processes a key/value pair to procreate a set of intermediate value pairs & key, and a reduce function that merges all intermediate values be associated with the same intermediate key. In this paper, we aimed to demonstrate a close-up view about MapReduce. The MapReduce is a famous framework for data-intensive distributed computing of batch jobs. This is over-simplify fault tolerance, many implementations of MapReduce materialize the overall output of every map and reduce task before it can be consumed. Finally, we also discuss the comparison between RDBMS and MapReduce, and famous scheduling algorithms in this field.
Key-Words / Index Term
Big Data, MapReduce, Scheduling, Processing Layer, Indexing, Data Layout
References
[1]. Kim, G.-H., Trimi, S., & Chung, J.-H. (2014). Big-data applications in the government sector. Communicationsof the ACM, 57(3), pp 78–85.
[2]. Dr. Yusuf Perwej, “An Experiential Study of the Big Data,” for published in the International Transaction of Electrical and Computer Engineers System (ITECES), USA, ISSN (Print): 2373-1273 ISSN (Online): 2373-1281, Vol. 4, No. 1, page 14-25, March 2017, DOI:10.12691/iteces-4-1-3.
[3]. R. Murugesh, I. Meenatchi, "A Study Using PI on: Sorting Structured Big Data In Distributed Environment Using Apache Hadoop MapReduce", International Journal of Computer Sciences and Engineering, Vol.2, Issue.8, pp.35-38, 2014.
[4]. “Apache Hadoop,” Apache. [Online]. Available: http://hadoop.apache.org/. [Accessed: 18-Feb-2015].
[5]. M. Khan, P. M. Ashton, M. Li, G. A. Taylor, I. Pisica, and J. Liu, “Parallel Detrended Fluctuation Analysis for Fast Event Detection on Massive PMU Data,” Smart Grid, IEEE Trans., vol. 6, no. 1, pp. 360–368, Jan. 2015.
[6]. K. Parimala1 G. Rajkumar, A. Ruba, S. Vijayalakshmi, "Challenges and Opportunities with Big Data", International Journal of Scientific Research in Computer Science and Engineering, Vol.5, Issue.5, pp.16-20, 2017.
[7]. Lee, D., Kim, J.-S., & Maeng, S. “Large-scale incremental processing with MapReduce”, Future Generation Computer Systems, 36, pp 66–79, (2014), doi:10.1016/j.future.2013.09.010.
[8]. M. Khan, M. Li, P. Ashton, G. Taylor, and J. Liu, “Big data analytics on PMU measurements,” in Fuzzy Systems and Knowledge Discovery (FSKD), 2014 11th International Conference on, 2014, pp. pp 715–719.
[9]. Qi, C., Cheng, L., & Zhen, X. (2014). Improving mapreduce performance using smart speculative execution strategy. IEEE Transactions on Computers, Vol. 63(4), pp 954–967. Doi:10.1109/TC.2013.15.
[10]. J. Kwon, K. Park, D. Lee, S. Lee, PSR: Pre-computing Solutions in RDBMS for Fast Web services Composition Search, in: Proceedings of the 2nd International Conference on Web Services, Salt Lake City, Utah, USA, ICWS 2007, pp. 808-815.
[11]. Yan, F., Cherkasova, L., Zhang, Z., & Smirni, E. (2014). Heterogeneous cores for mapreduce processing: Opportunity or challenge? Paper presented at the proceedings of IEEE/IFIP NOMS.
[12]. Chen, R., & Chen, H. , Tiled-MapReduce: Efficient and flexible MapReduce processing on multicore with tiling. ACM Transactions on Architecture and Code Optimization (TACO), Volume 10 Issue 1, April 2013, pp 3.
[13]. Dean, J. & S. Ghemawat (2004). Mapreduce: simpli_ed data processing on large clusters. In Proceedings of the 6th conference on Symposium on Opearting Systems Design & Imple-mentation - Volume 6, OSDI`04, Berkeley, CA, USA, pp. 10-10. USENIX Association.
[14]. Lee, K.-H., Y.-J. Lee, H. Choi, Y. D. Chung, & B. Moon “Parallel data processing with mapreduce: a survey”, SIGMOD Rec. vol 40 (4), pp 11-20. (2012).
[15]. S. Sakr, A. Liu, A. Fayoumi, "The family of mapreduce and large-scale data processing systems", ACM Computing Surveys, vol. 46, no. 1, pp. 1-44, 2013.
[16]. Q. He, Q. Tan, X. Ma, Z. Shi, "The high-activity parallel implementation of data preprocessing based on MapReduce", Proc. of the 5th International Conference on Rough Set and Knowledge Technology, 2010.
[17]. Google developers: Web metrics - size and number of resources,” https:// developers.google.com/speed/articles/web-metrics, accessed: 11/04/2013.
[18]. Mapreduce:Chainingjobs,http://developer.yahoo.com / hadoop/ tutorial/ module4.html#chaining, accessed: 11/04/2013.
[19]. Richter, S., J.-A. Quian_e-Ruiz, S. Schuh, & J. Dittrich. “Towards zero-overhead adaptive indexing in hadoop”, (2012), CoRR abs/1212.3480.
[20]. Dittrich, J., J.-A. Quian_e-Ruiz, A. Jindal, Y. Kargin, V. Setty, & J. Schad (2010, September). Hadoop++: making a yellow elephant run like a cheetah (without it even noticing). Proc. VLDB Endow.vol 3 (1-2), 515-529.
[21]. Eltabakh, M. Y., F. Ozcan, Y. Sismanis, P. J. Haas, H. Pirahesh, & J. Vondrak (2013). Eagleeyed elephant: Split-oriented indexing in hadoop. In Proceedings of the 16th International Conference on Extending Database Technology, EDBT `13, New York, NY, USA, pp. 89-100. ACM.
[22]. Ailamaki, A., D. J. DeWitt, M. D. Hill, & M. Skounakis (2001). Weaving relations for cache performance. In Proceedings of the 27th International Conference on Very Large Data Bases, VLDB `01, San Francisco, CA, USA, pp. 169-180. Morgan Kaufmann Publishers Inc.
[23]. https://hadoop.apache.org/docs/r1.2.1/fair_scheduler.html
[24]. Xingwu Zheng, Zhou Zhou, Xu Yang, Zhiling Lan, Jia Wang, "Exploring Plan-Based Scheduling for Large-Scale Computing Systems", Cluster Computing (CLUSTER) 2016 IEEE International Conference on, pp. 259-268, 2016, ISSN 2168-9253.
[25]. Y. Tao Y, Q. Zhang, L. Shi and P. Chen, “ Job scheduling optimization for multi-user MapReduce clusters ”, In: The fourth international symposium on parallel architectures, algorithms and programming. IEEE; 2011. pp 213–17.
[26]. Nikolaos D. Doulamis, Panagiotis Kokkinos, Emmanouel Varvarigos, "Resource Selection for Tasks with Time Requirements Using Spectral Clustering", Computers IEEE Transactions on, vol. 63, pp. 461-474, 2014, ISSN 0018-9340.
[27]. Mohammad Hammoud, M. Suhail Rehman, Majd F. Sakr, "Center-of-Gravity Reduce Task Scheduling to Lower MapReduce Network Traffic", IEEE, 2012, pp.49–58. doi:10.1109/CLOUD.2012.92
[28]. P. Nguyen, T. Simon, M. Halem, D. Chapman and Q. Le, “ A hybrid scheduling algorithm for data intensive workloads in aMapReduce environment”, In: Proceedings of the 2012 IEEE/ ACM fifth international conference on utility and cloud computing. Washington, DC, USA: IEEE computer society; UCC`12, 2012, pp 161-168.