Dimensionality Reduction and Comparison of Classification Models for Breast Cancer Prognosis
R. Garg1 , V. Mongia2
- Computer Science, Guru Nanak College, Moga, India.
- Computer Science, Guru Nanak College, Moga, India.
Correspondence should be addressed to: 87rajnigarg@gmail.com.
Section:Research Paper, Product Type: Journal Paper
Volume-6 ,
Issue-1 , Page no. 308-312, Jan-2018
CrossRef-DOI: https://doi.org/10.26438/ijcse/v6i1.308312
Online published on Jan 31, 2018
Copyright © R. Garg, V. Mongia . This is an open access article distributed under the Creative Commons Attribution License, which permits unrestricted use, distribution, and reproduction in any medium, provided the original work is properly cited.
View this paper at Google Scholar | DPI Digital Library
How to Cite this Paper
- IEEE Citation
- MLA Citation
- APA Citation
- BibTex Citation
- RIS Citation
IEEE Citation
IEEE Style Citation: R. Garg, V. Mongia, “Dimensionality Reduction and Comparison of Classification Models for Breast Cancer Prognosis,” International Journal of Computer Sciences and Engineering, Vol.6, Issue.1, pp.308-312, 2018.
MLA Citation
MLA Style Citation: R. Garg, V. Mongia "Dimensionality Reduction and Comparison of Classification Models for Breast Cancer Prognosis." International Journal of Computer Sciences and Engineering 6.1 (2018): 308-312.
APA Citation
APA Style Citation: R. Garg, V. Mongia, (2018). Dimensionality Reduction and Comparison of Classification Models for Breast Cancer Prognosis. International Journal of Computer Sciences and Engineering, 6(1), 308-312.
BibTex Citation
BibTex Style Citation:
@article{Garg_2018,
author = {R. Garg, V. Mongia},
title = {Dimensionality Reduction and Comparison of Classification Models for Breast Cancer Prognosis},
journal = {International Journal of Computer Sciences and Engineering},
issue_date = {1 2018},
volume = {6},
Issue = {1},
month = {1},
year = {2018},
issn = {2347-2693},
pages = {308-312},
url = {https://www.ijcseonline.org/full_paper_view.php?paper_id=1675},
doi = {https://doi.org/10.26438/ijcse/v6i1.308312}
publisher = {IJCSE, Indore, INDIA},
}
RIS Citation
RIS Style Citation:
TY - JOUR
DO = {https://doi.org/10.26438/ijcse/v6i1.308312}
UR - https://www.ijcseonline.org/full_paper_view.php?paper_id=1675
TI - Dimensionality Reduction and Comparison of Classification Models for Breast Cancer Prognosis
T2 - International Journal of Computer Sciences and Engineering
AU - R. Garg, V. Mongia
PY - 2018
DA - 2018/01/31
PB - IJCSE, Indore, INDIA
SP - 308-312
IS - 1
VL - 6
SN - 2347-2693
ER -
![]() |
![]() |
![]() |
1237 | 475 downloads | 283 downloads |
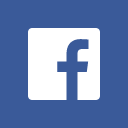
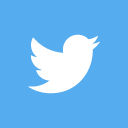
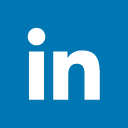
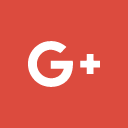
Abstract
Cancer is a most prevailing problem in the society now days. Generally cancer specifically Breast cancer is a major problem in women. On among three cases of cancer is a Breast cancer. There are many factors that affect the cancer. All these factors and the symptoms in the patient can be recorded using hardware and software. Now days, due to advancement in technology data of patient is recorded and processed by using analytical method. Data mining provides various methods to process this data effectively and efficiently. This processed data can be proven very useful in earlier detection of diseases. The earlier detection of these symptoms can be proven helpful to save life of a patient. In our research, original data on Breast cancer from Winconsin has been taken. This data set has 10 attribute and 699 instances. In this study, a comparative model has been developed that compare performance of various data mining technique on the dataset. The study reveals that BayesNet is the best classifier that correctly predicts cancer survivability in the patient. Further, KStar is the fastest algorithm that takes lowest computation time for the classification. In the next step dimensionality reduction using gain ratio is performed to find out most dominant factors causing Breast cancer.
Key-Words / Index Term
Data Mining, Breast Cancer, Bayesian, SVM, Decision Tree, Regression Model
References
[1]. H. Trevor, T. Robert, and F. Jerome, The Elements of Statistical Learning: Data Mining, Inference, and Prediction, 2nd ed., vol. 2. Springer: New York, 2009, pp. 32-36.
[2]. N.T.Nghe, P. Janecek, and P. Haddawy, “A comparative analysis of techniques for predicting academic performance", ASEE/IEEE Frontiers in Education Conference, pp. T2G7-T2G12, 2007.
[3]. M. Lichman, UCI Machine Learning Repository, http://www.cs.waikato.ac.nz/ml/weka,2013
[4]. J. Han and M. Kamber, Data Mining: Concepts and Techniques, 2nd ed. University of Illinois at Urbana-Champaign Elsevier San Francisco, 2009, pp. 285-306
[5]. Bellaachia, Abdelghani, and Erhan Guven, "Predicting breast cancer survivability using data mining techniques", Age, Vol. 58, Issue 13, 2006, pp. 10-110
[6]. Anunciacao Orlando, Gomes C. Bruno, Vinga Susana, Gaspar Jorge, Oliveira L. Arlindo and Rueff Jose, “A Data Mining approach for detection of high-risk Breast Cancer groups,” Advances in Soft Computing, vol. 74, pp. 43-51, 2010.
[7]. Shelly Gupta, Dharminder Kumar,Anand Sharma, “DATA MINING CLASSIFICATION TECHNIQUES APPLIED FOR BREAST CANCER DIAGNOSIS AND PROGNOSIS “
Vol. 2 No. 2 Apr-May 2011
[8]. Ahmad LG*, Eshlaghy AT, Poorebrahimi A, Ebrahimi M and Razavi AR “Using Three Machine Learning Techniques for Predicting Breast Cancer Recurrence” Health and Medical Informatics 2013, 4:2
[9]. Htet Thazin Tike Thein1 and Khin Mo Mo Tun “An Approach For Breast Cancer Diagnosis Classification Using Neural Network” Advanced Computing: An International Journal (ACIJ), Vol.6, No.1, January 2015
[10]. Uma Ojha, Savita Goel, ”A study on prediction of breast cancer recurrence using data mining techniques” Cloud Computing, Data Science & Engineering - Confluence, 2017 , Noida Inida.
[11]. K. Saravanapriya and J. Bagyamani, “Performance analysis of Classification Algorithms on Diabetes DataSet” . International Journal of Computer Science and Engineering (IJCSE), Vol. 5, Issue-9, pp 15-20, sept-2017