Deforestation Identification Model using RBC-Region Based Clustering
B. Tamil Selvi1 , M. Parvathy2 , PL. Prabha3 , C.H. Sumalakshmi4
- Computer Science and Engineering, K.L.N College of Information Technology, Madurai, India.
- Computer Science and Engineering, K.L.N College of Information Technology, Madurai, India.
- Computer Science and Engineering, K.L.N College of Information Technology, Madurai, India.
- Computer Science and Engineering, K.L.N College of Information Technology, Madurai, India.
Section:Research Paper, Product Type: Journal Paper
Volume-6 ,
Issue-3 , Page no. 177-181, Mar-2018
CrossRef-DOI: https://doi.org/10.26438/ijcse/v6i3.177181
Online published on Mar 30, 2018
Copyright © B. Tamil Selvi, M. Parvathy, PL. Prabha, C.H. Sumalakshmi . This is an open access article distributed under the Creative Commons Attribution License, which permits unrestricted use, distribution, and reproduction in any medium, provided the original work is properly cited.
View this paper at Google Scholar | DPI Digital Library
How to Cite this Paper
- IEEE Citation
- MLA Citation
- APA Citation
- BibTex Citation
- RIS Citation
IEEE Style Citation: B. Tamil Selvi, M. Parvathy, PL. Prabha, C.H. Sumalakshmi, “Deforestation Identification Model using RBC-Region Based Clustering,” International Journal of Computer Sciences and Engineering, Vol.6, Issue.3, pp.177-181, 2018.
MLA Style Citation: B. Tamil Selvi, M. Parvathy, PL. Prabha, C.H. Sumalakshmi "Deforestation Identification Model using RBC-Region Based Clustering." International Journal of Computer Sciences and Engineering 6.3 (2018): 177-181.
APA Style Citation: B. Tamil Selvi, M. Parvathy, PL. Prabha, C.H. Sumalakshmi, (2018). Deforestation Identification Model using RBC-Region Based Clustering. International Journal of Computer Sciences and Engineering, 6(3), 177-181.
BibTex Style Citation:
@article{Selvi_2018,
author = {B. Tamil Selvi, M. Parvathy, PL. Prabha, C.H. Sumalakshmi},
title = {Deforestation Identification Model using RBC-Region Based Clustering},
journal = {International Journal of Computer Sciences and Engineering},
issue_date = {3 2018},
volume = {6},
Issue = {3},
month = {3},
year = {2018},
issn = {2347-2693},
pages = {177-181},
url = {https://www.ijcseonline.org/full_paper_view.php?paper_id=1781},
doi = {https://doi.org/10.26438/ijcse/v6i3.177181}
publisher = {IJCSE, Indore, INDIA},
}
RIS Style Citation:
TY - JOUR
DO = {https://doi.org/10.26438/ijcse/v6i3.177181}
UR - https://www.ijcseonline.org/full_paper_view.php?paper_id=1781
TI - Deforestation Identification Model using RBC-Region Based Clustering
T2 - International Journal of Computer Sciences and Engineering
AU - B. Tamil Selvi, M. Parvathy, PL. Prabha, C.H. Sumalakshmi
PY - 2018
DA - 2018/03/30
PB - IJCSE, Indore, INDIA
SP - 177-181
IS - 3
VL - 6
SN - 2347-2693
ER -
![]() |
![]() |
![]() |
537 | 364 downloads | 262 downloads |
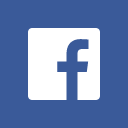
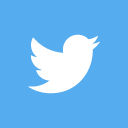
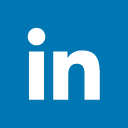
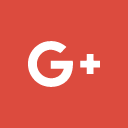
Abstract
Deforestation is the permanent ruin of forests in order to make the land available for other uses. Deforestation activities come in many ways including forest fire, logging, and mining. There is a need to detect deforestation to protect available resources. Satellites remote sensing provides fundamental information for accessing deforestation effect. In this paper, we proposed two method to detect deforestation 1.Extraction based RGB bands - with the help of clustering pixels combined with RGB color bands. Vegetation coverage estimated by scheming of RGB pixels .Region clustering were demonstrated to be more suitable for identifying the waste, sand, cultivated region. Classification accuracy of the features of interest varied from 80 to 95 percent.2.Decorrelation stretching- technique to improve the visual distinction of an image and representing the deforestation effect which understood by human through image. Finally, Histogram of an RGB bands was proposed to estimate the distribution of each pixel in an image which could give the clarity of vegetation coverage and make forest detection more efficient. The sample forest area around Madurai district was taken through the satellite and selected as a test site.
Key-Words / Index Term
Satellite remote sensing, Vegetation coverage , Region clustering, Decorrelation stretching, Histogram
References
[1] Tucker, Compton J.,John RG Townshend. "Strategies for monitoring tropical deforestation using satellite data." International Journal of Remote Sensing 21.6-7 (2000): 1461-1471.
[2] Rachmawan, Irene Erlyn Wina, Yasushi Kiyoki, and Shiori Sasaki. "An automatic feature extraction method of satellite multispectral images for interpreting deforestation effects in soil degradation." International Journal on Advanced Science, Engineering and Information Technology 7.2 (2017): 676-687.
[3] Geyer, Philipp, Arno Schlüter, and Sasha Cisar. "Application of clustering for the development of retrofit strategies for large building stocks." Advanced Engineering Informatics 31 (2017): 32-47.
[4] Pan, Bin, et al. "A novel spectral-unmixing-based green algae area estimation method for GOCI data." IEEE Journal of Selected Topics in Applied Earth Observations and Remote Sensing 10.2 (2017): 437-449.
[5] Badamasi, M. M., et al. "NDVI threshold classification and change detection of vegetation cover at the Falgore Game Reserve in Kano State, Nigeria." Sokoto Journal of the social sciences 2.2 (2010): 174-194.
[6] Emrah Hancer, Dervis Karaboga, “A Comprehensive Survey of Traditional, Merge-Split and Evolutionary Approaches Proposed for Determination of Cluster Number”, Swarm and Evolutionary Computation, S2210-6502(16)30047-5.
[7] Collins, Curtis A., David W. Wilkinson, and David L. Evans. "Multi-temporal analysis of landsat data to determine forest age classes for the mississippi statewide forest inventory~ preliminary results." Analysis of Multi-Temporal Remote Sensing Images, 2005 International Workshop on the. IEEE, 2005.
[8] Bosch, J. Mv, and J. D. Hewlett. "A review of catchment experiments to determine the effect of vegetation changes on water yield and evapotranspiration." Journal of hydrology 55.1-4 (1982): 3-23.
[9] Lingli Yu, Kaijun Zhou , Yongliang Yang, Haichu Chen,Bionic “RSTN invariant feature extraction method for image recognition and its application”, The Institute of Engineering and Technology. ISSN 1751-9659.
[10] Mello, Marcio Pupin, et al. "Spectral-Temporal Analysis by Response Surface applied to detect deforestation in the Brazilian Amazon." Analysis of Multi-temporal Remote Sensing Images (Multi-Temp), 2011 6th International Workshop on the. IEEE, 2011.
[11] S.P. Kullarkar, S.V. Jain “Review on Rain drop detection and removal using k-means clustering and Hough transformation” International Journal of Computer Sciences and Engineering, Vol.6(1), Jan 2017, E-ISSN: 2347-2693
[12] Lal, R. "Deforestation and land use effects on soil degradation and rehabilitation in western Nigeria. II. Soil chemical properties." Land Degradation & Development 7.2 (1996): 87-98.
[13] Glaser, Bruno, Johannes Lehmann, and Wolfgang Zech. "Ameliorating physical and chemical properties of highly weathered soils in the tropics with charcoal-a review." Biology and fertility of soils 35.4 (2002): 219-230.
[14] Downton, Mary W. "Measuring tropical deforestation: development of the methods." Environmental Conservation 22.3 (1995): 229-240.