An Effective Segmentation Approach of Medical Images Based on Beta Mixture Model
K.Vanitha 1 , M. Suresh Kumar2 , Sk. Althaf Rahaman3
- Department of Computer Science, GITAM (Deemed to be UNIVERSITY),Visakhapatnam, INDIA.
- Department of Computer Science, GITAM (Deemed to be UNIVERSITY),Visakhapatnam, INDIA.
- Department of Computer Science, GITAM (Deemed to be UNIVERSITY),Visakhapatnam, INDIA.
Section:Research Paper, Product Type: Journal Paper
Volume-6 ,
Issue-3 , Page no. 225-229, Mar-2018
CrossRef-DOI: https://doi.org/10.26438/ijcse/v6i3.225229
Online published on Mar 30, 2018
Copyright © K.Vanitha, M. Suresh Kumar, Sk. Althaf Rahaman . This is an open access article distributed under the Creative Commons Attribution License, which permits unrestricted use, distribution, and reproduction in any medium, provided the original work is properly cited.
View this paper at Google Scholar | DPI Digital Library
How to Cite this Paper
- IEEE Citation
- MLA Citation
- APA Citation
- BibTex Citation
- RIS Citation
IEEE Style Citation: K.Vanitha, M. Suresh Kumar, Sk. Althaf Rahaman, “An Effective Segmentation Approach of Medical Images Based on Beta Mixture Model,” International Journal of Computer Sciences and Engineering, Vol.6, Issue.3, pp.225-229, 2018.
MLA Style Citation: K.Vanitha, M. Suresh Kumar, Sk. Althaf Rahaman "An Effective Segmentation Approach of Medical Images Based on Beta Mixture Model." International Journal of Computer Sciences and Engineering 6.3 (2018): 225-229.
APA Style Citation: K.Vanitha, M. Suresh Kumar, Sk. Althaf Rahaman, (2018). An Effective Segmentation Approach of Medical Images Based on Beta Mixture Model. International Journal of Computer Sciences and Engineering, 6(3), 225-229.
BibTex Style Citation:
@article{Kumar_2018,
author = {K.Vanitha, M. Suresh Kumar, Sk. Althaf Rahaman},
title = {An Effective Segmentation Approach of Medical Images Based on Beta Mixture Model},
journal = {International Journal of Computer Sciences and Engineering},
issue_date = {3 2018},
volume = {6},
Issue = {3},
month = {3},
year = {2018},
issn = {2347-2693},
pages = {225-229},
url = {https://www.ijcseonline.org/full_paper_view.php?paper_id=1787},
doi = {https://doi.org/10.26438/ijcse/v6i3.225229}
publisher = {IJCSE, Indore, INDIA},
}
RIS Style Citation:
TY - JOUR
DO = {https://doi.org/10.26438/ijcse/v6i3.225229}
UR - https://www.ijcseonline.org/full_paper_view.php?paper_id=1787
TI - An Effective Segmentation Approach of Medical Images Based on Beta Mixture Model
T2 - International Journal of Computer Sciences and Engineering
AU - K.Vanitha, M. Suresh Kumar, Sk. Althaf Rahaman
PY - 2018
DA - 2018/03/30
PB - IJCSE, Indore, INDIA
SP - 225-229
IS - 3
VL - 6
SN - 2347-2693
ER -
![]() |
![]() |
![]() |
701 | 387 downloads | 316 downloads |
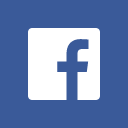
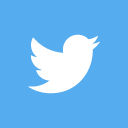
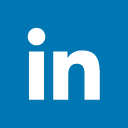
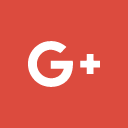
Abstract
Image segmentation aims at investigating and the Images with respect to the pattern of the pixels. Many Models are addressed in this direction. Among the various focused areas of segmentation, medical segmentation has gained importance; this is due to the fact that proper categorization of the pixels helps in the identification of the diseases. However, for successful segmentation one require to judge suitable features. In this paper, mainly we are proposing a Bivariate Beta Mixture Model (BMM) for segmenting the medical images by considering the Bivariate features. In order to implement the model, Berkley Bench Mark dataset is considered. This proposed model Performance is evaluated by using PSNR, MSE, IF, Average difference (AD).
Key-Words / Index Term
Beta Mixture Model, Berkley Images, IF, PSNR, MSE, Image Segmentation
References
[1] Adelino R. Ferreira da Silva: “Bayesian mixture models of variable dimension for image segmentation”, Computer methods and programs in biomedicine, pp.1-14, 2009.
[2] Ahmet M. Eskicioglu and Paul S. Fisher: “Image Quality Measures and Their Performance”, IEEE Transactions on Communications, Vol. 43, No.12, pp. 2959 – 2965, 1995.
[3] D. L. Pham, C. Y. Xu, and J. L. Prince: “A survey of current methods in medical image segmentation”, Annual Review of Biomedical Engineering, vol. 2, pp.315–337, 2000.
[4] David W. Shattuck, GautamPrasada, MubeenaMirzaa, Katherine L. Narra and Arthur W. Togaa: “Online resource for validation of brain segmentation methods”, ELSEVIER, NeuroImage Volume 45, Issue 2, 1, Pages 431-439, 2009.
[5] Dr.Samir Kumar Bandhyopadhyay, TuhinUtsab Paul: “Segmentation of Brain MRI Image – A Review”, International Journal of Advanced Research in Computer Science and Software Engineering, Vol. 2, Issue 3, pp.409-413, 2012.
[6] G. Dugas-Phocion, M. Á. González Ballester, G. Malandain, C. Lebrunand N. Ayache: “Improved EM-based tissue segmentation andpartial volume effect quantification in multi-sequence brain MRI”, International Conference Medical Image Computing and Computer-Assisted Intervention, Vol. 3216, pp. 26–33, 2004.
[7] GuangJianTian, Yong Xia, Yanning Zhang, Dagan Feng:“Hybrid Genetic and Variational Expectation-Maximization Algorithm for Gaussian-Mixture-Model-Based Brain MR Image Segmentation”, IEEE Transactions on Information Technology in Biomedicine, Vol. 15, No. 3, 2011.
[8] Herng-Hua Chang, Daniel J. Valentino, Gary R. Duckwiler and Arthur W. Toga: “Segmentation of Brain MR Images Using a Charged Fluid Model”, IEEE Transactions on Biomedical Engineering, Vol.54, No.10, pp. 1798-1813, 2007.
[9] J. Ashburner and K.J.Friston: “Multimodal image co registration and partitioning – A unified framework”, NeuroImage, Vol.6, pp 209-217, 1997.
[10] J.A. Hartigan: Clustering Algorithms, New York: Wiley, 1975.
[11] Shubhangi D.C., Sabahat Fatima, "Privacy-Preserving Outsourcing of Medical Image Data using SIFT Descriptor", International Journal of Scientific Research in Network Security and Communication, Vol.5, Issue.3, pp.141-145, 2017.
[12] P. Umorya, R. Singh, "A Comparative Based Review on Image Segmentation of Medical Image and its Technique", International Journal of Scientific Research in Computer Science and Engineering, Vol.5, Issue.2, pp.71-76, 2017
[13] Nagesh Vadaparthi, “Unsupervised Medical Image Segmentation on Brain MRI images using Skew Gaussian Distribution”, IEEE – International Conference on Recent Trends in Information Technology, pp.1293 – 1297, 2011.
[14] Sonam S. Gavhande, S.B.Jadhav, “Image Segmentation and Identification of Brain Tumor from MRI Image”, International Research Journal of Engineering and Technology (IRJET), Volume: 02 Issue: 02 . PP.167-170, 2015.
[15] Vrushali Borase, Gayatri Naik, Vaishali Londhe, “Brain MR Image Segmentation for Tumor Detection using Artificial Neural”, International Journal Of Engineering And Computer Science, Volume 6 Issue 1, pp. 20160-20163,2017.
[16] K Van Leemput, F. Maes, D. Vandeurmeulen, and P. Suetens: “Automated model-based tissue classification of MR images of the brain”, IEEE Transactions Medical Imaging, Vol. 18, no. 10, pp. 897–908, 1999.
[17] Nookala Venu, B. Anuradha: “Medical Image Segmentation Using Kernal Based Fuzzy”, International Journal of Engineering Innovation & Research, Vol. 4, Issue 1, pp-207-212, 2015.
[18] M. Prastawa, E. Bullitt, S. Ho, and G. Gerig: “Robust estimation forbrain tumor segmentation”, in International Conference Medical Image Computing and Computer-Assisted Intervention, LNCS 2879, pp. 530–537, 2003.
[19] Yazid Cherfa, Assia Cherfa, Yacine Kabir, Sammyh Kassous, Assia Jaillard, Michel Dojat, and Catherine Garbay: “Segmentation of Magnetic Resonance Brain Images Using Edge and Region Cooperation Characterization of Stroke Lesions”, International Arab Journal of Information Technology, Vol. 4, No 3. pp. 281-288, 2007.
[20] Ruchi,” Study of Different Brain Tumor MRI Image Segmentation Techniques”, International Journal of Computer Science Engineering and Technology (IJCSET), Vol 4, Issue 4, PP.133-136, 2014.
[21] Azzeddine Riahi, “Image Segmentation Techniques Based on Fuzzy C-Means and Otsu, Applied to the Brain MRI in Tumor Detection”, International Journal of Computer Sciences and Engineering (IJCSE), Vol 3, Issue 12, PP. 89-101, 2015.