Prediction Model for Diabetes Mellitus Using Machine Learning Techniques
N.A. Farooqui1 , Ritika 2 , A. Tyagi3
- Computer Applications, DIT University, Dehradun, India.
- Computer Applications, DIT University, Dehradun, India.
- Computer Applications, DIT University, Dehradun, India.
Section:Research Paper, Product Type: Journal Paper
Volume-6 ,
Issue-3 , Page no. 292-296, Mar-2018
CrossRef-DOI: https://doi.org/10.26438/ijcse/v6i3.292296
Online published on Mar 30, 2018
Copyright © N.A. Farooqui, Ritika, A. Tyagi . This is an open access article distributed under the Creative Commons Attribution License, which permits unrestricted use, distribution, and reproduction in any medium, provided the original work is properly cited.
View this paper at Google Scholar | DPI Digital Library
How to Cite this Paper
- IEEE Citation
- MLA Citation
- APA Citation
- BibTex Citation
- RIS Citation
IEEE Style Citation: N.A. Farooqui, Ritika, A. Tyagi, “Prediction Model for Diabetes Mellitus Using Machine Learning Techniques,” International Journal of Computer Sciences and Engineering, Vol.6, Issue.3, pp.292-296, 2018.
MLA Style Citation: N.A. Farooqui, Ritika, A. Tyagi "Prediction Model for Diabetes Mellitus Using Machine Learning Techniques." International Journal of Computer Sciences and Engineering 6.3 (2018): 292-296.
APA Style Citation: N.A. Farooqui, Ritika, A. Tyagi, (2018). Prediction Model for Diabetes Mellitus Using Machine Learning Techniques. International Journal of Computer Sciences and Engineering, 6(3), 292-296.
BibTex Style Citation:
@article{Farooqui_2018,
author = {N.A. Farooqui, Ritika, A. Tyagi},
title = {Prediction Model for Diabetes Mellitus Using Machine Learning Techniques},
journal = {International Journal of Computer Sciences and Engineering},
issue_date = {3 2018},
volume = {6},
Issue = {3},
month = {3},
year = {2018},
issn = {2347-2693},
pages = {292-296},
url = {https://www.ijcseonline.org/full_paper_view.php?paper_id=1798},
doi = {https://doi.org/10.26438/ijcse/v6i3.292296}
publisher = {IJCSE, Indore, INDIA},
}
RIS Style Citation:
TY - JOUR
DO = {https://doi.org/10.26438/ijcse/v6i3.292296}
UR - https://www.ijcseonline.org/full_paper_view.php?paper_id=1798
TI - Prediction Model for Diabetes Mellitus Using Machine Learning Techniques
T2 - International Journal of Computer Sciences and Engineering
AU - N.A. Farooqui, Ritika, A. Tyagi
PY - 2018
DA - 2018/03/30
PB - IJCSE, Indore, INDIA
SP - 292-296
IS - 3
VL - 6
SN - 2347-2693
ER -
![]() |
![]() |
![]() |
690 | 477 downloads | 287 downloads |
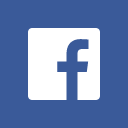
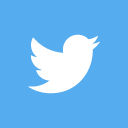
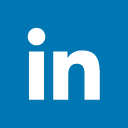
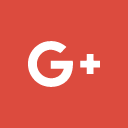
Abstract
In today’s world diabetes is the major health challenges in India. It is a group of a syndrome that results in too much sugar in the blood. It is a protracted condition that affects the way the body mechanizes the blood sugar. Prevention and prediction of diabetes mellitus is increasingly gaining interest in medical sciences. The aim is how to predict at an early stage of diabetes using different machine learning techniques. In this paper basically, we use well-known classification that are Decision tree, K- Nearest Neighbors, Support Vector Machine, and Random forest. These classification techniques used with Pima Indians diabetes dataset. Therefore, we predict diabetes at different stage and analyze the performance of different classification techniques. We Also proposed a conceptual model for the prediction of diabetes mellitus using different machine learning techniques. In this paper we also compare the accuracy of the different machine learning techniques to finding the diabetes mellitus at early stage.
Key-Words / Index Term
Diabetes; Decision Tree, K-Nearest Neighbors, Machine Learning, Random Forest, Support Vector Machine.
References
[1] International Diabetes federation. Diabetic Atlas Fifth Edition 2011.
[2] American Diabetes Association. Diagnosis and classification of diabetes mellitus. Diabetes Care 2009;32(Suppl. 1): S62–7.
[3] Krentz AJ, Bailey CJ. Oral antidiabetic agents: current role in type 2 diabetes mellitus. Drugs 2005;65(3):385–411.
[4] Tsave O, Halevas E, Yavropoulou MP, Kosmidis Papadimitriou A, Yovos JG, Hatzidimitriou A, et al. Structure-specific adipogenic capacity of novel, welldefined ternary Zn(II)-Schiff base materials. Biomolecular correlations in zincinduced differentiation of 3T3-L1 pre-adipocytes to adipocytes. J Inorg Biochem Nov 2015; 152:123–37.
[5] Halevas E, Tsave O, Yavropoulou MP, Hatzidimitriou A, Yovos JG, Psycharis V, et al. Design, synthesis and characterization of novel binary V(V)-Schiff base materials linked with insulin-mimetic vanadium-induced differentiation of 3T3-L1 fibroblasts to adipocytes. Structure–function correlations at the molecular level. J Inorg Biochem Jun 2015; 147:99–115.
[6] Tsave O, Yavropoulou MP, Kafantari M, Gabriel C, Yovos JG, Salifoglou A. The adipogenic potential of Cr(III). A molecular approach exemplifying metalinduced enhancement of insulin mimesis in diabetes mellitus II. J Inorg Biochem Oct 2016; 163:323–31.
[7] Sakurai H, Kojima Y, Yoshikawa Y, Kawabe K, Yasui H. Antidiabetic vanadium(IV) and zinc(II) complexes review article coordination. Chem Rev March 2002; 226(1–2):187–98.
[8] Nongyao Nai-arun, Rungruttikarn Moungmai(2015)Comparison of classifiers for the risk of diabetes ELSEVIER Procedia Computer Science 69 (2015) 132-142.
[9] Pima Indian diabetes datasets from UCI Repository.
[10] Çalisir D, Dogantekin E. An automatic diabetes diagnosis system based on LDA Wavelet Support Vector Machine Classifier. Expert Syst Appl 2011;38(7):8311–5.
[11] Ganji MF, Abadeh MS. A fuzzy classification system based on ant colony optimization for diabetes disease diagnosis. Expert Syst Appl 2011;38(12):14650–9.
[12] Georga EI, Protopappas VC, Ardigò D, Marina M, Zavaroni I, Polyzos D, et al. Multivariate prediction of subcutaneous glucose concentration in type 1 diabetes patients based on support vector regression. IEEE J Biomed Health Inform 2013; (1):71–81.
[13] Agarwal V, Podchiyska T, Banda JM, Goel V, Leung TI, Minty EP, et al. Learning statistical models of phenotypes using noisy labeled training data. J Am Med Inform Assoc May 12, 2016.
[14] El-Sappagh S, Elmogy M, Riad AM. A fuzzy-ontology-oriented case-based reasoning framework for semantic diabetes diagnosis. Artif Intell Med Nov 2015;65(3):179208.
[15] Chin-Yuan Fan, Pei-Chann Chang, Jyun-Jie Lin, J.C. Hsieh. A Hybrid model combining Case-based reasoning and Fuzzy Decision Tree for Medical Data Classification. Applied Soft Computing; 2011; 11(1); pp.632–644.
[16] Nihat Yilmaz, Onur Inan, Mustafa Serter Uzer. A New Data Preparation Method Based on Clustering Algorithms for Diagnosis Systems of Heart and Diabetes Diseases. Springer: Transaction Processing Systems:J Med Syst; April 2014;38:48.
[17] Yuan R, Guangchen B. Determination of Optimal SVM Parameters by Using Genetic Algorithm/Particle Swarm Optimization. Journal of Computers; 2010; No.5;1160-1169.
[18] Patil B M, Joshi R C, Toshniwal D. Impact of K-Means on the performance of classifiers for labeled data. Comm. Com. Inf. Sc.94;2010; 423-434.
[19] Aishwarya S, Anto S. A Medical Decision Support System based on Genetic Algorithm and Least Square Support Vector Machine for Diabetes Disease Diagnosis. International Journal of Engineering Sciences & Research Technology; April2014; 3(4).
[20] OhW, KimE, CastroMR, Caraballo PJ, Kumar V, Steinbach MS, et al. Type 2 diabetes mellitus trajectories and associated risks. Big Data Mar 1 2016;4(1):25–30.
[21] Ioannis Kavakiotis, Olga Tsave, Athanasios Salifoglou, Nicos Maglaveras, Ioannis Vlahavas, Ioanna Chouvarda Machine learning and datamining methods in diabetes research ELSEVIER Computational and Structural Biotechnology journal 15(2017) 104-116.
[22] J. Pradeep Kandhasamy, S. Balamurali(2015) Performance analysis of classifier models to predict diabetes mellitus ELSEVIER Procedia Computer Science 47 (2015) 45-5.
[23] N. Radha and S. Ramya “Performance Analysis of Machine Learning Algorithms for Predicting Chronic Kidney Disease”, International Journal of Computer Sciences and Engineering Vol.-3, Issue -8, pp(72-76) Aug 2015 E-ISSN: 2347-2693.