Modeling the Process Parameters of Roller Burnishing using RSM and Prediction of Micro Hardness using Artificial Neural Network
Vijay Kurkute1 , Sandip Chavan2
- Sinhgad College of Engineering Pune, Savitribai Phule Pune University, Pune (India).
- Dept. Mechanical Engineering MAEER’s Maharashtra Institute of Technology, Pune (India).
Section:Research Paper, Product Type: Journal Paper
Volume-6 ,
Issue-4 , Page no. 43-50, Apr-2018
CrossRef-DOI: https://doi.org/10.26438/ijcse/v6i4.4350
Online published on Apr 30, 2018
Copyright © Vijay Kurkute, Sandip Chavan . This is an open access article distributed under the Creative Commons Attribution License, which permits unrestricted use, distribution, and reproduction in any medium, provided the original work is properly cited.
View this paper at Google Scholar | DPI Digital Library
How to Cite this Paper
- IEEE Citation
- MLA Citation
- APA Citation
- BibTex Citation
- RIS Citation
IEEE Citation
IEEE Style Citation: Vijay Kurkute, Sandip Chavan, “Modeling the Process Parameters of Roller Burnishing using RSM and Prediction of Micro Hardness using Artificial Neural Network,” International Journal of Computer Sciences and Engineering, Vol.6, Issue.4, pp.43-50, 2018.
MLA Citation
MLA Style Citation: Vijay Kurkute, Sandip Chavan "Modeling the Process Parameters of Roller Burnishing using RSM and Prediction of Micro Hardness using Artificial Neural Network." International Journal of Computer Sciences and Engineering 6.4 (2018): 43-50.
APA Citation
APA Style Citation: Vijay Kurkute, Sandip Chavan, (2018). Modeling the Process Parameters of Roller Burnishing using RSM and Prediction of Micro Hardness using Artificial Neural Network. International Journal of Computer Sciences and Engineering, 6(4), 43-50.
BibTex Citation
BibTex Style Citation:
@article{Kurkute_2018,
author = {Vijay Kurkute, Sandip Chavan},
title = {Modeling the Process Parameters of Roller Burnishing using RSM and Prediction of Micro Hardness using Artificial Neural Network},
journal = {International Journal of Computer Sciences and Engineering},
issue_date = {4 2018},
volume = {6},
Issue = {4},
month = {4},
year = {2018},
issn = {2347-2693},
pages = {43-50},
url = {https://www.ijcseonline.org/full_paper_view.php?paper_id=1844},
doi = {https://doi.org/10.26438/ijcse/v6i4.4350}
publisher = {IJCSE, Indore, INDIA},
}
RIS Citation
RIS Style Citation:
TY - JOUR
DO = {https://doi.org/10.26438/ijcse/v6i4.4350}
UR - https://www.ijcseonline.org/full_paper_view.php?paper_id=1844
TI - Modeling the Process Parameters of Roller Burnishing using RSM and Prediction of Micro Hardness using Artificial Neural Network
T2 - International Journal of Computer Sciences and Engineering
AU - Vijay Kurkute, Sandip Chavan
PY - 2018
DA - 2018/04/30
PB - IJCSE, Indore, INDIA
SP - 43-50
IS - 4
VL - 6
SN - 2347-2693
ER -
![]() |
![]() |
![]() |
1189 | 779 downloads | 378 downloads |
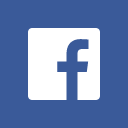
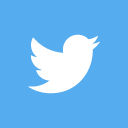
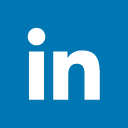
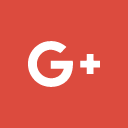
Abstract
Neural network computational techniques are a new alternative to conventional numerical modeling. This paper presents modeling using response surface methodology (RSM). Box and Wilson Central Composite Design (CCD) is used for preparing experiment matrix. The independent parameters in the experiment are speed, feed, force and number of tool passes. These variables are controlled during the burnishing process. The response parameter is micro hardness. Experimental samples are prepared using Single Roller Burnishing Tools (Carbide). Vickers micro hardness tester is used to measure micro hardness. A quadratic mathematical model is developed using RSM. An Artificial neural network (ANN) model is developed using three-layer feed-forward back-propagation. The neural network model is trained using measured values of micro hardness. The different algorithms are used to train the model. Best performance is achieved with correlation coefficient 0.9. This study concludes that an artificial neural network is the best alternative to fit the nonlinear data.
Key-Words / Index Term
Burnishing , RSM, Micro Hardness, ANN
References
[1] B. Bhushan, Introduction to tribology: John Wiley & Sons, 2013.
[2] P. Benardos and G. C. Vosniakos, "Prediction of surface roughness in CNC face milling using neural networks and Taguchi`s design of experiments," Robotics and Computer-Integrated Manufacturing, vol. 18, pp. 343-354, 2002.
[3] T. Özel and Y. Karpat, "Predictive modeling of surface roughness and tool wear in hard turning using regression and neural networks," International Journal of Machine Tools and Manufacture, vol. 45, pp. 467-479, 2005.
[4] N. B. Fredj and R. Amamou, "Ground surface roughness prediction based upon experimental design and neural network models," The International Journal of Advanced Manufacturing Technology, vol. 31, pp. 24-36, 2006.
[5] C.-F. J. Kuo and Y.-S. Wu, "Application of a Taguchi-based neural network prediction design of the film coating process for polymer blends," The International Journal of Advanced Manufacturing Technology, vol. 27, pp. 455-461, 2006.
[6] M. Mia and N. R. Dhar, "Prediction of surface roughness in hard turning under high pressure coolant using Artificial Neural Network," Measurement, vol. 92, pp. 464-474, 2016.
[7] K. Çelebi, E. Uludamar, E. Tosun, Ş. Yıldızhan, K. Aydın, and M. Özcanlı, "Experimental and artificial neural network approach of noise and vibration characteristic of an unmodified diesel engine fuelled with conventional diesel, and biodiesel blends with natural gas addition," Fuel, vol. 197, pp. 159-173, 2017.
[8] R. Sharda and R. B. Patil, "Connectionist approach to time series prediction: an empirical test," Journal of Intelligent Manufacturing, vol. 3, pp. 317-323, October 01 1992.
[9] D. Kim, "Normalization methods for input and output vectors in backpropagation neural networks," International Journal of Computer Mathematics, vol. 71, pp. 161-171, 1999/01/01 1999.
[10] I. El-Sonbaty, U. Khashaba, A. Selmy, and A. Ali, "Prediction of surface roughness profiles for milled surfaces using an artificial neural network and fractal geometry approach," Journal of Materials Processing Technology, vol. 200, pp. 271-278, 2008.
[11] G. Zhang, B. E. Patuwo, and M. Y. Hu, "Forecasting with artificial neural networks:: The state of the art," International journal of forecasting, vol. 14, pp. 35-62, 1998.
[12] A. M. Zain, H. Haron, and S. Sharif, "Prediction of surface roughness in the end milling machining using Artificial Neural Network," Expert Systems with Applications, vol. 37, pp. 1755-1768, 2010.
[13] S. Makridakis and R. L. Winkler, "Averages of forecasts: Some empirical results," Management Science, vol. 29, pp. 987-996, 1983.