Cloud based Predictive Model for Detection of ‘Chronic Kidney Disease’ Risk
Stuti Nathaniel1 , Anand Motwani2 , Arpit Saxena3
- Department of computer science, Sagar Institute of Science & Technology Research, Bhopal.
- Department of computer science, Sagar Institute of Science & Technology Research, Bhopal.
- Department of computer science, Sagar Institute of Science & Technology Research, Bhopal.
Section:Research Paper, Product Type: Journal Paper
Volume-6 ,
Issue-4 , Page no. 185-188, Apr-2018
CrossRef-DOI: https://doi.org/10.26438/ijcse/v6i4.185188
Online published on Apr 30, 2018
Copyright © Stuti Nathaniel, Anand Motwani, Arpit Saxena . This is an open access article distributed under the Creative Commons Attribution License, which permits unrestricted use, distribution, and reproduction in any medium, provided the original work is properly cited.
View this paper at Google Scholar | DPI Digital Library
How to Cite this Paper
- IEEE Citation
- MLA Citation
- APA Citation
- BibTex Citation
- RIS Citation
IEEE Citation
IEEE Style Citation: Stuti Nathaniel, Anand Motwani, Arpit Saxena, “Cloud based Predictive Model for Detection of ‘Chronic Kidney Disease’ Risk,” International Journal of Computer Sciences and Engineering, Vol.6, Issue.4, pp.185-188, 2018.
MLA Citation
MLA Style Citation: Stuti Nathaniel, Anand Motwani, Arpit Saxena "Cloud based Predictive Model for Detection of ‘Chronic Kidney Disease’ Risk." International Journal of Computer Sciences and Engineering 6.4 (2018): 185-188.
APA Citation
APA Style Citation: Stuti Nathaniel, Anand Motwani, Arpit Saxena, (2018). Cloud based Predictive Model for Detection of ‘Chronic Kidney Disease’ Risk. International Journal of Computer Sciences and Engineering, 6(4), 185-188.
BibTex Citation
BibTex Style Citation:
@article{Nathaniel_2018,
author = {Stuti Nathaniel, Anand Motwani, Arpit Saxena},
title = {Cloud based Predictive Model for Detection of ‘Chronic Kidney Disease’ Risk},
journal = {International Journal of Computer Sciences and Engineering},
issue_date = {4 2018},
volume = {6},
Issue = {4},
month = {4},
year = {2018},
issn = {2347-2693},
pages = {185-188},
url = {https://www.ijcseonline.org/full_paper_view.php?paper_id=1866},
doi = {https://doi.org/10.26438/ijcse/v6i4.185188}
publisher = {IJCSE, Indore, INDIA},
}
RIS Citation
RIS Style Citation:
TY - JOUR
DO = {https://doi.org/10.26438/ijcse/v6i4.185188}
UR - https://www.ijcseonline.org/full_paper_view.php?paper_id=1866
TI - Cloud based Predictive Model for Detection of ‘Chronic Kidney Disease’ Risk
T2 - International Journal of Computer Sciences and Engineering
AU - Stuti Nathaniel, Anand Motwani, Arpit Saxena
PY - 2018
DA - 2018/04/30
PB - IJCSE, Indore, INDIA
SP - 185-188
IS - 4
VL - 6
SN - 2347-2693
ER -
![]() |
![]() |
![]() |
731 | 514 downloads | 358 downloads |
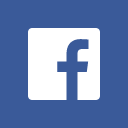
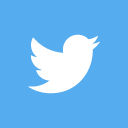
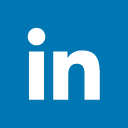
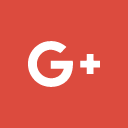
Abstract
Chronic kidney disease (CKD) is an increasing and serious disease impacting public health worldwide. The symptoms of CKD are often appearing too late and many patients inevitably face pain and expensive medical treatments. The ultimate treatment is frequent dialysis or Kidney transplant. Early detection of disease through symptoms can prevent the disease progression by referral to appropriate health care services. Machine Learning (ML) techniques can help in identifying the potential risk by discovering knowledge from medical reports of patient. Thus helps in preventing the disease progression. Several models for detecting the risk of CKD, proposed in the literature are based on Data Mining (DM) techniques like classification, clustering and regression etc. These models are demonstrated using variety of languages like Python, Java and tools like Weka and RapidMiner.This research aims at developing a Cloud based Predictive Model to detect the possibilities of CKD and its progression in patients with some health issues like hypertension and diabetes.
Key-Words / Index Term
Chronic Kidney Disease (CKD), Health Care, Microsoft Azure, Logistic Regression, Machine Learning, Predictive Model
References
[1] Jameson K, Jick S, Hagberg KW, Ambegaonkar B, Giles A, O’Donoghue D., “Prevalence and management of chronic kidney disease in primary care patients in the UK”. Int J Clin Pract. 2014;68 (9):1110–21.
[2] www.google.co.in/search?q=Chronic+kidney+disease
[3] Collins GS, Omar O, Shanyinde M, Yu L-M. A systematic review finds prediction models for chronic kidney disease were poorly reported and often developed using inappropriate methods. J Clin Epidemiol. 2013; 66(3):268–77.
[4] Echouffo-Tcheugui JB, Kengne AP. Risk models to predict chronic kidney disease and its progression: a systematic review. Remuzzi G, editor. PLoS Med. 2012; 9(11):e1001344.
[5] https://msdn.microsoft.com/en-us/library/azure/dn905930.aspx
[6] Sumit Basu, “Empirical Results on the Generalization Capabilities and Convergence Properties of the Bayes Point Machine”, Technical Report, December, 1999.
[7] https://msdn.microsoft.com/en-us/library/azure/dn905994.aspx
[8] http://www.stat.cmu.edu/~cshalizi/uADA/12/lectures/ch12.pdf
[9] Paolo Fraccaro, Sabine van der Veer, Benjamin Brown, Mattia Prosperi, Donal O’Donoghue, Gary S. Collins, Iain Buchan and Niels Peek, “An external validation of models to predict the onset of chronic kidney disease using population-based electronic health records from Salford, UK”, RESEARCH ARTICLE, BMC Medicine (2016) 14:104.
[10] V. Kunwar, K. Chandel, A. S. Sabitha and A. Bansal, "Chronic Kidney Disease analysis using data mining classification techniques," 2016 6th International Conference - Cloud System and Big Data Engineering (Confluence), Noida, 2016, pp. 300-305.
[11] M. D. Başar, P. Sarı, N. Kılıç and A. Akan, "Detection of chronic kidney disease by using Adaboost ensemble learning approach," 2016 24th Signal Processing and Communication Application Conference (SIU), Zonguldak, 2016, pp. 773-776.
[12] P. Soundarapandian and L. J. Rubini, http://archive.ics.uci.edu/ml/datasets/Chronic_Kidney_Disease, UCI Machine Learning Repository, Irvine, 2015.
[13] Z. Sedighi, H. Ebrahimpour-Komleh and S. J. Mousavirad, "Featue selection effects on kidney desease analysis," 2015 International Congress on Technology, Communication and Knowledge (ICTCK), Mashhad, 2015, pp. 455-459.
[14] Subasi A., Alickovic E., Kevric J. (2017) Diagnosis of Chronic Kidney Disease by Using Random Forest. In: Badnjevic A. (eds) CMBEBIH 2017. IFMBE Proceedings, vol 62. Springer, Singapore
[15] N. Chetty, K. S. Vaisla and S. D. Sudarsan, "Role of attributes selection in classification of Chronic Kidney Disease patients," 2015 International Conference on Computing, Communication and Security (ICCCS), Pamplemousses, 2015, pp. 1-6.
[16] https://studio.azureml.net/