Review on Machine Learning Based Suggestion System
Ankit Sharma1 , Ekta 2
- Dept. of CSE, Baba Farid College of Engineering and Technology, Bathinda, India.
- Dept. of CSE, Baba Farid College of Engineering and Technology, Bathinda, India.
Section:Review Paper, Product Type: Journal Paper
Volume-6 ,
Issue-4 , Page no. 242-244, Apr-2018
CrossRef-DOI: https://doi.org/10.26438/ijcse/v6i4.242244
Online published on Apr 30, 2018
Copyright © Ankit Sharma, Ekta . This is an open access article distributed under the Creative Commons Attribution License, which permits unrestricted use, distribution, and reproduction in any medium, provided the original work is properly cited.
View this paper at Google Scholar | DPI Digital Library
How to Cite this Paper
- IEEE Citation
- MLA Citation
- APA Citation
- BibTex Citation
- RIS Citation
IEEE Style Citation: Ankit Sharma, Ekta, “Review on Machine Learning Based Suggestion System,” International Journal of Computer Sciences and Engineering, Vol.6, Issue.4, pp.242-244, 2018.
MLA Style Citation: Ankit Sharma, Ekta "Review on Machine Learning Based Suggestion System." International Journal of Computer Sciences and Engineering 6.4 (2018): 242-244.
APA Style Citation: Ankit Sharma, Ekta, (2018). Review on Machine Learning Based Suggestion System. International Journal of Computer Sciences and Engineering, 6(4), 242-244.
BibTex Style Citation:
@article{Sharma_2018,
author = {Ankit Sharma, Ekta},
title = {Review on Machine Learning Based Suggestion System},
journal = {International Journal of Computer Sciences and Engineering},
issue_date = {4 2018},
volume = {6},
Issue = {4},
month = {4},
year = {2018},
issn = {2347-2693},
pages = {242-244},
url = {https://www.ijcseonline.org/full_paper_view.php?paper_id=1877},
doi = {https://doi.org/10.26438/ijcse/v6i4.242244}
publisher = {IJCSE, Indore, INDIA},
}
RIS Style Citation:
TY - JOUR
DO = {https://doi.org/10.26438/ijcse/v6i4.242244}
UR - https://www.ijcseonline.org/full_paper_view.php?paper_id=1877
TI - Review on Machine Learning Based Suggestion System
T2 - International Journal of Computer Sciences and Engineering
AU - Ankit Sharma, Ekta
PY - 2018
DA - 2018/04/30
PB - IJCSE, Indore, INDIA
SP - 242-244
IS - 4
VL - 6
SN - 2347-2693
ER -
![]() |
![]() |
![]() |
543 | 338 downloads | 258 downloads |
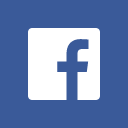
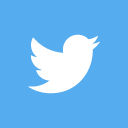
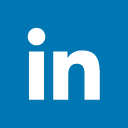
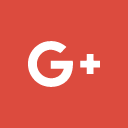
Abstract
Suggestion system plays vital role in WWW world and used in many applications. It has created the collection of many application, created global village and growth for numerous information. This paper represents the overview of Approaches and techniques generated in Suggestion system. Suggestion system is divided into three main types Collaborative Filtering, Content based and hybrid-based Method. The work in our categories collaborative filtering as Memory based type and Model based type. The paper discusses in detail the methods, their pros and cons. The paper proves to be a milestone in the research field of suggestion system.
Key-Words / Index Term
Suggestion, Collaborative filtering, Model based, Memory based, Content based, Hybrid.
References
[1] Alexandrin Popescu and Lyle H. Ungar, David M. Pennock and Steve Lawrence,” Probabilistic Models for Unified
[2] Collaborative and Content-Based Suggestion in Sparse-Data Environments”, POPESCUL ET Al,2001
[3] Greg Linden, Brent Smith, and Jeremy York,”Amazon.com Suggestions Item-to-Item Collaborative Filtering”, IEEE Computer Society 2003.
[4] Jun Wang, Arjen P. de Vries, Marcel J.T. Reinders,” Unifying Userbased and Itembased Collaborative Filtering Approaches by Similarity Fusion”, 2006 ACM.
[5] Panagiotis Symeonidis *, Alexandros Nanopoulos, Apostolos N. Papadopoulos,Yannis Manolopoulos,” Collaborative recommender systems: Combining effectiveness and efficiency”, 2007 Elsevier Ltd.
[6] Kazuyoshi Yoshii, Masataka Goto, Kazunori Komatani, Tetsuya Ogata, Hiroshi G. Okuno,” An Efficient Hybrid Music Recommender System Using an Incrementally TrainableProbabilistic Generative Model”,IEEE 2008
[7] Akhmed Umyarov, Alexander Tuzhilin,”Improving Collaborative Filtering Suggestions Using External Data”, 2008 IEEE.
[8] Zunping Cheng, Neil Hurley,” Effective Diverse and Obfuscated Attacks on Model-based Recommender Systems” 2009 ACM.
[9] Hyeong-Joon Kwon, Tae-Hoon Lee, and Kwang-Seok Hong,” Improved Memory-based Collaborative Filtering Using Entropy-based Similarity Measures”, 2009 ACADEMY PUBLISHER.
[10] Asela Gunawardana, Christopher Meek,” A Unified Approach to Building Hybrid Recommender Systems” 200X ACM
[11] Sergio Cleger-Tamayo, Juan M. Fern´andez-Luna, and Juan F. Huete, ”A New Criteria for Selecting Neighborhood in Memory-Based Recommender Systems*”,Springer-Verlag Berlin Heidelberg 2011.
[12] Pasquale Lops, Marco de Gemmis and Giovanni Semeraro,” Content-based Recommender Systems: State of the Art and Trends”, Springer 2011
[13] [12] Yoav Bergner,Stefan Dr¨oschlery, Gerd Kortemeyer, Saif Rayyan, Daniel Seaton, and David E. Pritchard,” ModelBased
[14] Collaborative Filtering Analysis of Student Response Data: MachineLearning Item Response Theory”,2012.
[15] Svetlin Bostandjiev, John O‟Donovan, Tobias Höllerer,” TasteWeights:A Visual Interactive Hybrid Recommender System” 2012 ACM
[16] Jiankai Sun, Shuaiqiang Wang, Byron J. Gao, Jun Ma,” Learning to Rank for Hybrid Suggestion”, 2012 ACM
[17] Meenakshi Sharma and Sandeep Mann, “A Survey of Recommender Systems: Approaches and Limitations” ,ICAECE- 2013.
[18] Royi Ronen, Noam Koenigstein, Elad Ziklik and Nir Nice,” Selecting Content-Based Features for Collaborative Filtering Recommenders”, ACM 2013