A PLANNED VIBRANT PROCEDURE FOR ASSOCIATION RULES IN BIG DATA
N.Subha 1 , N.Baby Kala2
- Department of Computer Science, KNG Arts College (W) Autonomous, Thanjavur, India.
- Department of Computer Science, KNG Arts College (W) Autonomous, Thanjavur, India.
Section:Research Paper, Product Type: Journal Paper
Volume-6 ,
Issue-4 , Page no. 383-388, Apr-2018
CrossRef-DOI: https://doi.org/10.26438/ijcse/v6i4.383388
Online published on Apr 30, 2018
Copyright © N.Subha, N.Baby Kala . This is an open access article distributed under the Creative Commons Attribution License, which permits unrestricted use, distribution, and reproduction in any medium, provided the original work is properly cited.
View this paper at Google Scholar | DPI Digital Library
How to Cite this Paper
- IEEE Citation
- MLA Citation
- APA Citation
- BibTex Citation
- RIS Citation
IEEE Citation
IEEE Style Citation: N.Subha, N.Baby Kala, “A PLANNED VIBRANT PROCEDURE FOR ASSOCIATION RULES IN BIG DATA,” International Journal of Computer Sciences and Engineering, Vol.6, Issue.4, pp.383-388, 2018.
MLA Citation
MLA Style Citation: N.Subha, N.Baby Kala "A PLANNED VIBRANT PROCEDURE FOR ASSOCIATION RULES IN BIG DATA." International Journal of Computer Sciences and Engineering 6.4 (2018): 383-388.
APA Citation
APA Style Citation: N.Subha, N.Baby Kala, (2018). A PLANNED VIBRANT PROCEDURE FOR ASSOCIATION RULES IN BIG DATA. International Journal of Computer Sciences and Engineering, 6(4), 383-388.
BibTex Citation
BibTex Style Citation:
@article{Kala_2018,
author = {N.Subha, N.Baby Kala},
title = {A PLANNED VIBRANT PROCEDURE FOR ASSOCIATION RULES IN BIG DATA},
journal = {International Journal of Computer Sciences and Engineering},
issue_date = {4 2018},
volume = {6},
Issue = {4},
month = {4},
year = {2018},
issn = {2347-2693},
pages = {383-388},
url = {https://www.ijcseonline.org/full_paper_view.php?paper_id=1906},
doi = {https://doi.org/10.26438/ijcse/v6i4.383388}
publisher = {IJCSE, Indore, INDIA},
}
RIS Citation
RIS Style Citation:
TY - JOUR
DO = {https://doi.org/10.26438/ijcse/v6i4.383388}
UR - https://www.ijcseonline.org/full_paper_view.php?paper_id=1906
TI - A PLANNED VIBRANT PROCEDURE FOR ASSOCIATION RULES IN BIG DATA
T2 - International Journal of Computer Sciences and Engineering
AU - N.Subha, N.Baby Kala
PY - 2018
DA - 2018/04/30
PB - IJCSE, Indore, INDIA
SP - 383-388
IS - 4
VL - 6
SN - 2347-2693
ER -
![]() |
![]() |
![]() |
692 | 448 downloads | 292 downloads |
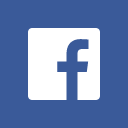
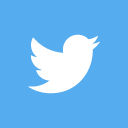
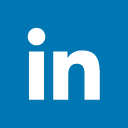
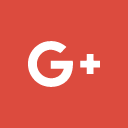
Abstract
In light of the touchy development of data that we are choking in, while we are starving for learning, mining data and data from generous databases has been seen as a key research point. In this way, Due to the gigantic size of data that exists in the databases and distribution centers and in light of the fact that these data are big, dynamic and change as often as possible it is troublesome and costly to do mining for visit examples and association rule starting with no outside help. In light of such an intrigue, this paper proposes a Dynamic Algorithm for Association Rules Mining in Big Data that is equipped for finding successive thing sets progressively and creating association rules from the thing sets by utilizing gathered learning put away in a database table, this table will be adjusted as often as possible when the framework runs each time and the new estimation of the table will be the aftereffect of preparing new embedded data added to the consequences of beforehand handled data. The proposed arrangement is executed utilizing C#.net and SQL server. The outcomes contrasted and the Apriori calculation. It was presume that Apriori calculation indicated preferable outcomes over the proposed calculation in the underlying runs, then again the proposed dynamic calculation gave comes about close to Apriori calculation on visit runs that utilization modest number of exchanges yet the proposed dynamic calculation took less handling time than Apriori calculation by 63.95% on the regular runs that utilization big number of exchanges.
Key-Words / Index Term
Big data, Apriori algorithm, Association rules, Data mining
References
[1] Golnar Assadat Afzali, Shahriar Mohammadi, “Privacy preserving big data mining: association rule hiding using fuzzy logic approach”, IET Information Security, 2018, Vol. 12, No. 1, PP. 15 – 24.
[2] Gehao Sheng, Huijuan Hou, Xiuchen Jiang, Yufeng Chen, “A Novel Association Rule Mining Method of Big Data for Power Transformers State Parameters Based on Probabilistic Graph Model”, IEEE Transactions on Smart Grid, Vol. 9, No. 2, PP. 695 – 702, 2018.
[3] Saurav Mallik, Anirban Mukhopadhyay, Ujjwal Maulik*, “RANWAR: Rank-Based Weighted Association Rule Mining From Gene Expression and Methylation Data”, IEEE Transactions on NanoBioscience, Vol. 14, No. 1, PP. 59 – 66, 2015.
[4] Qin Ding, Qiang Ding, William Perrizo, “PARM—An Efficient Algorithm to Mine Association Rules From Spatial Data”, IEEE Transactions on Systems, Man, and Cybernetics, Part B (Cybernetics), Vol. 38, No. 6, PP. 1513 – 1524, 2008.
[5] M. Kantarcioglu, C. Clifton, “Privacy-preserving distributed mining of association rules on horizontally partitioned data”, IEEE Transactions on Knowledge and Data Engineering, Vol. 16, No. 9, PP. 1026 – 1037, 2004.
[6] F. Coenen, P. Leng, S. Ahmed, “Data structure for association rule mining: T-trees and P-trees”, IEEE Transactions on Knowledge and Data Engineering, Vol. 16, No. 6, PP. 774 – 778, 2004.
[7] D.Ragupathi, N.Jayaveeran, “The Design & Implementation of Transportation Procedure using Migration Techiques”, International Journal of Computer Sciences and Engineering, Vol.5, Issue.6, pp.273-278, 2017.
[8] Eui-Hong Han, G. Karypis, V. Kumar, “Scalable parallel data mining for association rules”, IEEE Transactions on Knowledge and Data Engineering, Vol. 12, No. 3, PP. 337 – 352, 2000.
[9] José María Luna, Alberto Cano, Mykola Pechenizkiy, Sebastián Ventura, “Speeding-Up Association Rule Mining With Inverted Index Compression”, IEEE Transactions on Cybernetics, Vol. 46, No. 12, PP. 3059 – 3072, 2016.
[10] Chin-Chen Chang, Chih-Yang Lin, “Perfect Hashing Schemes for Mining Association Rules”, The Computer Journal, Vol. 48, No. 2, PP. 168 – 179, 2005.
[11] Ruizhi Wu, Guangchun Luo, Qinli Yang, Junming Shao, “Learning Individual Moving Preference and Social Interaction for Location Prediction”, IEEE Access, Vol. 6, PP. 10675 – 10687, 2018.
[12] Xu He, Fan Min, William Zhu, “Comparison of Discretization Approaches for Granular Association Rule Mining”, Canadian Journal of Electrical and Computer Engineering, Vol. 37, No. 3, PP. 157 – 167, 2014.