A Study on Crowd Detection and Density Analysis for Safety Control
Mayur D. Chaudhari1 , Archana S. Ghotkar2
- Computer, Pune Institute of Computer Technology, Savitribai Phule Pune University, Pune, India.
- Computer, Pune Institute of Computer Technology, Savitribai Phule Pune University, Pune, India.
Section:Survey Paper, Product Type: Journal Paper
Volume-6 ,
Issue-4 , Page no. 424-428, Apr-2018
CrossRef-DOI: https://doi.org/10.26438/ijcse/v6i4.424428
Online published on Apr 30, 2018
Copyright © Mayur D. Chaudhari, Archana S. Ghotkar . This is an open access article distributed under the Creative Commons Attribution License, which permits unrestricted use, distribution, and reproduction in any medium, provided the original work is properly cited.
View this paper at Google Scholar | DPI Digital Library
How to Cite this Paper
- IEEE Citation
- MLA Citation
- APA Citation
- BibTex Citation
- RIS Citation
IEEE Citation
IEEE Style Citation: Mayur D. Chaudhari, Archana S. Ghotkar, “A Study on Crowd Detection and Density Analysis for Safety Control,” International Journal of Computer Sciences and Engineering, Vol.6, Issue.4, pp.424-428, 2018.
MLA Citation
MLA Style Citation: Mayur D. Chaudhari, Archana S. Ghotkar "A Study on Crowd Detection and Density Analysis for Safety Control." International Journal of Computer Sciences and Engineering 6.4 (2018): 424-428.
APA Citation
APA Style Citation: Mayur D. Chaudhari, Archana S. Ghotkar, (2018). A Study on Crowd Detection and Density Analysis for Safety Control. International Journal of Computer Sciences and Engineering, 6(4), 424-428.
BibTex Citation
BibTex Style Citation:
@article{Chaudhari_2018,
author = {Mayur D. Chaudhari, Archana S. Ghotkar},
title = {A Study on Crowd Detection and Density Analysis for Safety Control},
journal = {International Journal of Computer Sciences and Engineering},
issue_date = {4 2018},
volume = {6},
Issue = {4},
month = {4},
year = {2018},
issn = {2347-2693},
pages = {424-428},
url = {https://www.ijcseonline.org/full_paper_view.php?paper_id=1913},
doi = {https://doi.org/10.26438/ijcse/v6i4.424428}
publisher = {IJCSE, Indore, INDIA},
}
RIS Citation
RIS Style Citation:
TY - JOUR
DO = {https://doi.org/10.26438/ijcse/v6i4.424428}
UR - https://www.ijcseonline.org/full_paper_view.php?paper_id=1913
TI - A Study on Crowd Detection and Density Analysis for Safety Control
T2 - International Journal of Computer Sciences and Engineering
AU - Mayur D. Chaudhari, Archana S. Ghotkar
PY - 2018
DA - 2018/04/30
PB - IJCSE, Indore, INDIA
SP - 424-428
IS - 4
VL - 6
SN - 2347-2693
ER -
![]() |
![]() |
![]() |
1227 | 535 downloads | 326 downloads |
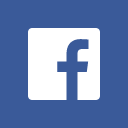
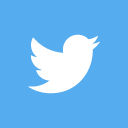
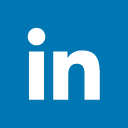
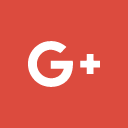
Abstract
Most of the studies based on tracking individuals, crowd counting, finding the region of motion and crowd detection. Crowd detection and density estimation from crowded images have a wide range of application such as crime detection, congestion, public safety, crowd abnormalities, visual surveillance and urban planning. The purpose of crowd density analysis is to calculate the concentration of the crowd in the videos of observers. Pattern recognition technique helps to estimate the crowd detection count and density by using face and detection. The job of detecting a face in the crowd is complicated due to its variability present in human faces including color, pose, expression, position, orientation, and illumination. The counting performance has been steadily improved because of Deep Convolutional Neural Network..
Key-Words / Index Term
Pattern Recognition, Computer Vision, Crowd Density Estimation, Detection, CNN
References
[1] Sami Abdulla, Mohsen Saleh, Shahrel Azmin Suandi, Haidi Ibrahim, “Recent survey on crowd density estimation and counting for visual surveillance”, Engineering Application of Artificial Intelligence 41 (2015) pp. 103-114, http://dx.doi.org/10.1016/j.e ngappai.2015.01.0 07
[2] Vishwanath A. Sindagi, Vishal M. Patel, “A Survey of Recent Advances in CNN-based Single Image Crowd Counting and Density Estimation”, Pattern Recognition Letters, elsevier 2017.
[3] Yingying Zhang, Desen Zhou, Siqin Chen, Shenghau Gao, Yi Ma, “Single-Image Crowd Counting via Multi-Column Convolutional Neural Network”, In CVPR, IEEE, pp. 589-597.
[4] Lingke Zeng, Xiangmin Xu, Bolun Cai, Suo, Qiu, Tong Zhang, “Multi-Scale Convolutional Neural Networks for Crowd Counting”, IEEE 2017.
[5] Di Kang, Zheng Ma, Antoni B. Chan, “Beyond Counting: Comparisons of Density Maps for Crowd Analysis Tasks - Counting, Detection, and Tracking”, IEEE 2017.
[6] Ankan Bansal, K S Venkatesh, “People Counting in high Density Crowds from Still Images”, IEEE 2015.
[7] Chuan Wang, Hua Zhang, Liang Yang, Si Liu, Ziaochun Cao, “Deep People Counting in Extremely Dense Crowds”, ACM 2015.
[8] Helbing, D., Brockmann, D., Chadefaux, T., Donnay, K., Blanke, U., Woolley-Meza, O., Moussaid, M., Johansson, A., Krause, J., Schutte, S., et al., 2014. “Saving human lives: what complexity science and information systems can contribute”. J. Stat. Phys., 147.
[9] Zhao, T., Nevatia, R., Wu, B., 2008. “Segmentation and tracking of multiple humans in crowded environments”. IEEE Trans. Pattern Anal. Mach. Intell. 30 (7), pp. 1198-1211.
[10] Zhan, B., Monekosso, D.N., Remagnino, P., Velastin, S.A., Xu, L.Q., 2008. “Crowd analysis: a survey. Machine Vision and Applications” 19, pp. 345-357.
[11] Junior, J.C.S.J., Musse, S.R., Jung, C.R., 2010. “ Crowd analysis using computer vision techniques”. IEEE Signal Processing Magazine 27, pp. 66-77.
[12] Li, T., Chang, H., Wang, M., Ni, B., Hong, R., Yan, S., 2015. “Crowded scene analysis: A survey. IEEE Transactions on Circuits and Systems for Video Technology” 25, pp. 367-386.
[13] Loy, C.C., Chen, K., Gong, S., Xiang, T., 2013. “Crowd counting and profiling: Methodology and evaluation, in: Modeling, Simulation and Visual Analysis of Crowds”. Springer, pp. 347-382.
[14] Jones, M.J., Snow, D., 2008. “Pedestrian detection using boosted features over many frames”. In: 19th International Conference on Pattern Recognition, 2008. ICPR 2008. IEEE, pp. 14, http://dx.doi.org/10.1109 /ICPR.2008.4761703.
[15] Viola, P., Jones, M.J., Snow, D., 2005. “Detecting pedestrians using patterns of motion and appearance”. Int. J. Comput. Vis. 63 (2), pp. 153-161.
[16] Schapire, R.E., Singer, Y., 1999. “Improved boosting algorithms using confidencerated predictions”. Mach. Learn. 37 (3), pp. 297-336
[17] Leibe, B., Seemann, E., Schiele, B., 2005. “Pedestrian detection in crowded scenes”. In: IEEE Computer Society Conference on Computer Vision and Pattern Recognition, 2005. CVPR 2005, vol. 1. IEEE, pp. 878-885, http://dx.doi.org/10.1109/CVPR.2005.272
[18] Lin, S.-F., Chen, J.-Y., Chao, H.-X., 2001. “Estimation of number of people in crowded scenes using perspective transformation”. IEEE Trans. Syst. Man Cybern. Part A Syst. Hum. 31 (6), pp. 645-654
[19] Lin, S.-F., Lin, C.-D., 2006. “Estimation of the pedestrians on a crosswalk”. In: International Joint Conference SICE-ICASE, 2006. IEEE, pp. 4931-4936, http:// dx.doi.org/10.1109/SICE.2006.314851
[20] Zhao, T., Nevatia, R., 2004. “Tracking multiple humans in complex situations”. IEEE Trans. Pattern Anal. Mach. Intell. 26 (9), 1208-1221.
[21] Rabaud, V., Belongie, S., 2006. “Counting crowded moving objects”. In: 2006 IEEE Computer Society Conference on Computer Vision and Pattern Recognition, vol. 1. IEEE, pp. 705-711, http://dx.doi.o rg/10.1109/CVPR.2006.92
[22] Shi, J., Tomasi, C., 1994. “Good features to track”. In: 1994 IEEE Computer Society Conference on Computer Vision and Pattern Recognition, 1994. Proceedings CVPR’94. IEEE, pp. 593-600, http://dx.doi.org/1 0.1109/CVPR.1994.323794
[23] Sidla, O., Lypetskyy, Y., Brandle, N., Seer, S., 2006. “Pedestrian detection and tracking for counting applications in crowded situations”. In: IEEE International Conference on Video and Signal Based Surveillance, 2006. AVSS’06. IEEE, p. 70, http://dx.doi.org/10.1109/AVSS.2006.91.
[24] Idrees, H., Saleemi, I., Seibert, C., Shah, M., 2013. “Multi-source multiscale counting in extremely dense crowd images”, in: Proceedings of the IEEE Conference on Computer Vision and Pattern Recognition, pp. 2547-2554.
[25] Pham, V.Q., Kozakaya, T., Yamaguchi, O., Okada, R., 2015. “Count forest: Co-voting uncertain number of targets using random forest for crowd density estimation”, in: Proceedings of the IEEE International Conference on Computer Vision, pp. 3253-3261
[26] Fu, M., Xu, P., Li, X., Liu, Q., Ye, M., Zhu, C., 2015. “Fast crowd density estimation with convolutional neural networks”. Engineering Applications of Artificial Intelligence 43, 81-88.
[27] Zhang, C., Li, H., Wang, X., Yang, X., 2015. “Cross-scene crowd counting via deep convolutional neural networks”, in: Proceedings of the IEEE Conference on Computer Vision and Pattern Recognition, pp. 833-841
[28] Shang, C., Ai, H., Bai, B., 2016. “End-to-end crowd counting via joint learning local and global count”, in: Image Processing (ICIP), 2016 IEEE International Conference on, IEEE. pp. 1215-1219
[29] Ankan Bansal and K. S. Venkatesh, 2015. “People Counting in High Density Crowds from Still Images”. International Journal of Computer and Electrical Engineering, pp. 316-324.