Machine-learning Techniques for Clinical Decision-making and Prediction: A Review
K. Jeberson1 , M. Kumar2 , R. Yadav3
- Dept. of Comp. Sci. & I.T, SIET, SHUATS, Allahabad, India.
- Indian Institute of Information Technology, Allahabad, India.
- Dept. of Comp. Sci. & I.T, SIET, SHUATS, Allahabad, India.
Section:Review Paper, Product Type: Journal Paper
Volume-6 ,
Issue-5 , Page no. 126-133, May-2018
CrossRef-DOI: https://doi.org/10.26438/ijcse/v6i5.126133
Online published on May 31, 2018
Copyright © K. Jeberson, M. Kumar, R. Yadav . This is an open access article distributed under the Creative Commons Attribution License, which permits unrestricted use, distribution, and reproduction in any medium, provided the original work is properly cited.
View this paper at Google Scholar | DPI Digital Library
How to Cite this Paper
- IEEE Citation
- MLA Citation
- APA Citation
- BibTex Citation
- RIS Citation
IEEE Style Citation: K. Jeberson, M. Kumar, R. Yadav, “Machine-learning Techniques for Clinical Decision-making and Prediction: A Review,” International Journal of Computer Sciences and Engineering, Vol.6, Issue.5, pp.126-133, 2018.
MLA Style Citation: K. Jeberson, M. Kumar, R. Yadav "Machine-learning Techniques for Clinical Decision-making and Prediction: A Review." International Journal of Computer Sciences and Engineering 6.5 (2018): 126-133.
APA Style Citation: K. Jeberson, M. Kumar, R. Yadav, (2018). Machine-learning Techniques for Clinical Decision-making and Prediction: A Review. International Journal of Computer Sciences and Engineering, 6(5), 126-133.
BibTex Style Citation:
@article{Jeberson_2018,
author = {K. Jeberson, M. Kumar, R. Yadav},
title = {Machine-learning Techniques for Clinical Decision-making and Prediction: A Review},
journal = {International Journal of Computer Sciences and Engineering},
issue_date = {5 2018},
volume = {6},
Issue = {5},
month = {5},
year = {2018},
issn = {2347-2693},
pages = {126-133},
url = {https://www.ijcseonline.org/full_paper_view.php?paper_id=1948},
doi = {https://doi.org/10.26438/ijcse/v6i5.126133}
publisher = {IJCSE, Indore, INDIA},
}
RIS Style Citation:
TY - JOUR
DO = {https://doi.org/10.26438/ijcse/v6i5.126133}
UR - https://www.ijcseonline.org/full_paper_view.php?paper_id=1948
TI - Machine-learning Techniques for Clinical Decision-making and Prediction: A Review
T2 - International Journal of Computer Sciences and Engineering
AU - K. Jeberson, M. Kumar, R. Yadav
PY - 2018
DA - 2018/05/31
PB - IJCSE, Indore, INDIA
SP - 126-133
IS - 5
VL - 6
SN - 2347-2693
ER -
![]() |
![]() |
![]() |
614 | 390 downloads | 267 downloads |
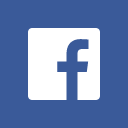
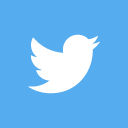
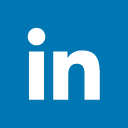
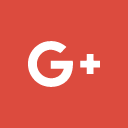
Abstract
The incredible growth in medical technologies has increased in accumulation of loads of data in various forms. Application of medical informatics techniques and tools transform data into various forms of stuff which are sutable for mining. Implementing data mining techniques on clinical data enable the discovery of priceless knowledge from the huge collection of information stored. This study aims to conduct a review systematically on the classification techniques applied on clinical data from the perspective of (i) Medicine and (ii) Health care. The outcomes of this study indicate that maximum amount of research was published in the years 2015 and 2016. In medical data mining research, the most popular algorithm used was decision tree. Elsevier was identified as the leading publisher, which has published plenty of articles in this domain. 75% of the articles belonged to the category ‘medicine’ and rest of the articles belonged to the category ‘health care’. Out of the 75% articles, most of them were related to prognosis and diagnosis of diseases and fewer studies have been conducted in treatment recommendation. Choosing the best therapy and identifying the ideal treatment plan is a challenging task in case of diseases like heart failure and cancer. Moreover there is insufficient machine-learning research conducted in kidney diseases especially in chronic kidney disease and end-stage renal disease which are considered a global threat nowadays.
Key-Words / Index Term
Classification, Data mining, Decision tree
References
[1] Afzal, M., Hussain, M., Khan, W. A., Ali, T., Lee, S., Huh, E. N., & Hydari, M. A., “Comprehensible knowledge model creation for cancer treatment decision making”, Computers in biology and medicine, Vol.82, pp.119-129.2017.
[2] Amaral, J. L., Lopes, A. J., Veiga, J., Faria, A. C., & Melo, P. L., “High-accuracy detection of airway obstruction in asthma using machine learning algorithms and forced oscillation measurements”, Computer methods and programs in biomedicine, Vol.144, pp.113-125, 2017.
[3] Armengol, E., “Classification of melanomas in situ using knowledge discovery with explained case-based reasoning”, Artificial Intelligence in Medicine, 51(2), pp.93-105, 2011.
[4] Askland, K. D., Garnaat, S., Sibrava, N. J., Boisseau, C. L., Strong, D., Mancebo, M., & Eisen, J., “Prediction of remission in obsessive compulsive disorder using a novel machine learning strategy”, International journal of methods in psychiatric research, 24(2), pp.156-169, 2015.
[5] Beaudoin, M., Kabanza, F., Nault, V., & Valiquette, L., “Evaluation of a machine learning capability for a clinical decision support system to enhance antimicrobial stewardship programs”, Artificial intelligence in medicine, 68, pp.29-36, 2016.
[6] Çakır, A., & Demirel, B., “A software tool for determination of breast cancer treatment methods using data mining approach”, Journal of medical systems, 35(6), pp.1503-1511, 2011.
[7] Cheruku, R., Edla, D. R., & Kuppili, V., “SM-RuleMiner: Spider monkey based rule miner using novel fitness function for diabetes classification”, Computers in biology and medicine, 81, pp.79-92, 2017.
[8] Chia, C. C., Rubinfeld, I., Scirica, B. M., McMillan, S., Gurm, H. S., & Syed, Z., “ Looking beyond historical patient outcomes to improve clinical models”, Science translational medicine, 4(131), 131ra49-131ra49, 2012.
[9] Christopher, J. J., Nehemiah, H. K., & Kannan, A., “A swarm optimization approach for clinical knowledge mining”, Computer methods and programs in biomedicine, 121(3), pp.137-148, 2015.
[10] Chuang, C. L., “Case-based reasoning support for liver disease diagnosis”, Artificial Intelligence in Medicine, 53(1), pp.15-23, 2011.
[11] Cruz-Ramirez, M., Hervas-Martinez, C., Fernandez, J. C., Briceno, J., & De La Mata, M., “Predicting patient survival after liver transplantation using evolutionary multi-objective artificial neural networks”, Artificial intelligence in medicine, 58(1), pp.37-49, 2011.
[12] Dean, J. A., Wong, K. H., Welsh, L. C., Jones, A. B., Schick, U., Newbold, K. L., ... & Gulliford, S. L., “Normal tissue complication probability (NTCP) modelling using spatial dose metrics and machine learning methods for severe acute oral mucositis resulting from head and neck radiotherapy”, Radiotherapy and Oncology, 120(1), pp.21-27, 2016.
[13] Deng, Y., Groll, M. J., & Denecke, K., “Rule-based Cervical Spine Defect Classification Using Medical Narratives”, Studies in health technology and informatics, 216, pp.1038-1038, 2015.
[14] Faria, B. M., Gonçalves, J., Reis, L. P., & Rocha, Á., “A clinical support system based on quality of life estimation”, Journal of medical systems, 39(10), pp.114, 2015.
[15] Gaebel, J., Kolter, T., Arlt, F., & Denecke, K., “Extraction Of Adverse Events From Clinical Documents To Support Decision Making Using Semantic Preprocessing”, Studies in health technology and informatics, 216, pp.1030-1036, 2015.
[16] Goodman, K. E., Lessler, J., Cosgrove, S. E., Harris, A. D., Lautenbach, E., Han, J. H., & Tamma, P. D., “A clinical decision tree to predict whether a bacteremic patient is infected with an extended-spectrum β-Lactamase–producing organism”, Clinical Infectious Diseases, 63(7), pp.896-903, 2016.
[17] Guerrero, J. M., Martínez-Tomás, R., Rincón, M., & Peraita, H., “Diagnosis of Cognitive Impairment Compatible with Early Diagnosis of Alzheimer’s Disease”, Methods of Information in Medicine, 55(01), pp.42-49, 2016.
[18] Ichikawa, D., Saito, T., Ujita, W., & Oyama, H., ”How can machine-learning methods assist in virtual screening for hyperuricemia? A healthcare machine-learning approach”, Journal of biomedical informatics, 64, pp.20-24, 2016.
[19] Lee, G., Gurm, H. S., & Syed, Z., “Predicting complications of percutaneous coronary intervention using a novel support vector method”, Journal of the American Medical Informatics Association, 20(4), pp.778-786, 2013.
[20] Liu, X., Lu, R., Ma, J., Chen, L., & Qin, B., “Privacy-preserving patient-centric clinical decision support system on naive Bayesian classification”, IEEE journal of biomedical and health informatics, 20(2), pp.655-668, 2016.
[21] Nahato, K. B., Harichandran, K. N., & Arputharaj, K., “Knowledge mining from clinical datasets using rough sets and backpropagation neural network”, Computational and mathematical methods in medicine, 2015.
[22] Ochab, M., & Wajs, W., “Expert system supporting an early prediction of the bronchopulmonary dysplasia”, Computers in biology and medicine, 69, pp.236-244, 2016.
[23] Ozden, F. O., Ozgonenel, O., Ozden, B., & Aydogdu, A., “Diagnosis of periodontal diseases using different classification algorithms: a preliminary study”, Nigerian journal of clinical practice, 18(3), pp.416-421, 2015.
[24] Parodi, S., Filiberti, R., Marroni, P., Libener, R., Ivaldi, G. P., Mussap, M., ... & Muselli, M., “Differential diagnosis of pleural mesothelioma using Logic Learning Machine”, BMC bioinformatics, 16(9), S3, 2015.
[25] Prilutsky, D., Rogachev, B., Marks, R. S., Lobel, L., & Last, M., “Classification of infectious diseases based on chemiluminescent signatures of phagocytes in whole blood”, Artificial intelligence in medicine, 52(3), pp.153-163, 2011.
[26] Rahulamathavan, Y., Veluru, S., Phan, R. C. W., Chambers, J. A., & Rajarajan, M., “Privacy-preserving clinical decision support system using gaussian kernel-based classification”, IEEE journal of biomedical and health informatics, 18(1), pp.56-66, 2014.
[27] Ramezankhani, A., Pournik, O., Shahrabi, J., Khalili, D., Azizi, F., & Hadaegh, F., “Applying decision tree for identification of a low risk population for type 2 diabetes. Tehran Lipid and Glucose Study”, Diabetes research and clinical practice, 105(3), pp.391-398, 2014.
[28] Ruiz-Fernandez, D., Torra, A. M., Soriano-Payá, A., Marin-Alonso, O., & Palencia, E. T., “Aid decision algorithms to estimate the risk in congenital heart surgery”, Computer methods and programs in biomedicine, 126, pp.118-127, 2016.
[29] Santos, R. S., Malheiros, S. M., Cavalheiro, S., & De Oliveira, J. P., “A data mining system for providing analytical information on brain tumors to public health decision makers”, Computer methods and programs in biomedicine, 109(3), pp.269-282, 2013.
[30] Settouti, N., Chikh, M. A., & Saidi, M., “Generating fuzzy rules for constructing interpretable classifier of diabetes disease”, Australasian physical & engineering sciences in medicine, 35(3), pp.257-270, 2012.
[31] Shahhoseini, R., Ghazvini, A., Esmaeilpour, M., Pourtaghi, G., & Tofighi, S., “Presentation of a model-based data mining to predict lung cancer”, Journal of research in health sciences, 15(3), pp.189-195, 2015.
[32] Somu, N., Raman, M. G., Kirthivasan, K., & Sriram, V. S., “Hypergraph based feature selection technique for medical diagnosis”, Journal of medical systems, 40(11), pp.239, 2016.
[33] Tang, H., Poynton, M. R., Hurdle, J. F., Baird, B. C., Koford, J. K., & Goldfarb-Rumyantzev, A. S., “Predicting three-year kidney graft survival in recipients with systemic lupus erythematosus”, ASAIO Journal, 57(4), pp.300-309, 2011.
[34] Taylor, R. A., Pare, J. R., Venkatesh, A. K., Mowafi, H., Melnick, E. R., Fleischman, W., & Hall, M. K., “Prediction of In‐hospital Mortality in Emergency Department Patients with Sepsis: A Local Big Data–Driven, Machine Learning Approach”, Academic emergency medicine, 23(3), pp.269-278, 2016.
[35] Tuti, T., Agweyu, A., Mwaniki, P., Peek, N., & English, M., “An exploration of mortality risk factors in non-severe pneumonia in children using clinical data from Kenya”, BMC medicine, 15(1), pp.201-210, 2017.
[36] Valdes, G., Solberg, T. D., Heskel, M., Ungar, L., & Simone II, C. B., “Using machine learning to predict radiation pneumonitis in patients with stage I non-small cell lung cancer treated with stereotactic body radiation therapy”, Physics in Medicine & Biology, 61(16), pp.6105-6112, 2016.
[37] Wang, Z., Wen, X., Lu, Y., Yao, Y., & Zhao, H., “Exploiting machine learning for predicting skeletal-related events in cancer patients with bone metastases”, Oncotarget, 7(11), pp.12612-12620, 2016.
[38] Weakley, A., Williams, J. A., Schmitter-Edgecombe, M., & Cook, D. J., “Neuropsychological test selection for cognitive impairment classification: A machine learning approach”, Journal of clinical and experimental neuropsychology, 37(9), pp.899-916, 2015.
[39] Wu, J. L., Tseng, H. S., Yang, L. H., Wu, H. K., Kuo, S. J., Chen, S. T., & Chen, D. R., “Prediction of axillary lymph node metastases in breast cancer patients based on pathologic information of the primary tumor”, Medical science monitor: international medical journal of experimental and clinical research, 20, pp.577-585, 2014.
[40] Zhu, K., Lou, Z., Zhou, J., Ballester, N., Kong, N., & Parikh, P., “Predicting 30-day Hospital Readmission with Publicly Available Administrative Database”, Methods of information in medicine, 54(06), pp.560-567, 2015.
[41] O.A. Mohamed Jafar, "A Study of Different Similarity Measures on the Performance of Fuzzy Clustering", International Journal of Computer Sciences and Engineering, Vol.6, Issue.4, pp.168-173, 2018.
[42] N. Radha and S. Ramya , "Performance Analysis of Machine Learning Algorithms for Predicting Chronic Kidney Disease", International Journal of Computer Sciences and Engineering, Vol.3, Issue.8, pp.72-76, 2015.
[43] Pier Luca Lanzi, “Machine Learning and Data Mining”,Technology, Education, 2007.