A Two-Stage Learning Method For Fault Detection of Machines Using Mechanical Big Data
M.S. Jamadagni1 , K.A. Nagarkar2 , V.S. Badame3 , C.R. Chindwin4 , V.M. Lomte5
- Computer Engineering, RMD Sinhgad School of Engineering, Savitribai Phule Pune University, Pune, India.
- Computer Engineering, RMD Sinhgad School of Engineering, Savitribai Phule Pune University, Pune, India.
- Computer Engineering, RMD Sinhgad School of Engineering, Savitribai Phule Pune University, Pune, India.
- Computer Engineering, RMD Sinhgad School of Engineering, Savitribai Phule Pune University, Pune, India.
- Computer Engineering, RMD Sinhgad School of Engineering, Savitribai Phule Pune Universit.
Section:Research Paper, Product Type: Journal Paper
Volume-6 ,
Issue-5 , Page no. 387-391, May-2018
CrossRef-DOI: https://doi.org/10.26438/ijcse/v6i5.387391
Online published on May 31, 2018
Copyright © M.S. Jamadagni, K.A. Nagarkar, V.S. Badame, C.R. Chindwin, V.M. Lomte . This is an open access article distributed under the Creative Commons Attribution License, which permits unrestricted use, distribution, and reproduction in any medium, provided the original work is properly cited.
View this paper at Google Scholar | DPI Digital Library
How to Cite this Paper
- IEEE Citation
- MLA Citation
- APA Citation
- BibTex Citation
- RIS Citation
IEEE Citation
IEEE Style Citation: M.S. Jamadagni, K.A. Nagarkar, V.S. Badame, C.R. Chindwin, V.M. Lomte, “A Two-Stage Learning Method For Fault Detection of Machines Using Mechanical Big Data,” International Journal of Computer Sciences and Engineering, Vol.6, Issue.5, pp.387-391, 2018.
MLA Citation
MLA Style Citation: M.S. Jamadagni, K.A. Nagarkar, V.S. Badame, C.R. Chindwin, V.M. Lomte "A Two-Stage Learning Method For Fault Detection of Machines Using Mechanical Big Data." International Journal of Computer Sciences and Engineering 6.5 (2018): 387-391.
APA Citation
APA Style Citation: M.S. Jamadagni, K.A. Nagarkar, V.S. Badame, C.R. Chindwin, V.M. Lomte, (2018). A Two-Stage Learning Method For Fault Detection of Machines Using Mechanical Big Data. International Journal of Computer Sciences and Engineering, 6(5), 387-391.
BibTex Citation
BibTex Style Citation:
@article{Jamadagni_2018,
author = {M.S. Jamadagni, K.A. Nagarkar, V.S. Badame, C.R. Chindwin, V.M. Lomte},
title = {A Two-Stage Learning Method For Fault Detection of Machines Using Mechanical Big Data},
journal = {International Journal of Computer Sciences and Engineering},
issue_date = {5 2018},
volume = {6},
Issue = {5},
month = {5},
year = {2018},
issn = {2347-2693},
pages = {387-391},
url = {https://www.ijcseonline.org/full_paper_view.php?paper_id=1991},
doi = {https://doi.org/10.26438/ijcse/v6i5.387391}
publisher = {IJCSE, Indore, INDIA},
}
RIS Citation
RIS Style Citation:
TY - JOUR
DO = {https://doi.org/10.26438/ijcse/v6i5.387391}
UR - https://www.ijcseonline.org/full_paper_view.php?paper_id=1991
TI - A Two-Stage Learning Method For Fault Detection of Machines Using Mechanical Big Data
T2 - International Journal of Computer Sciences and Engineering
AU - M.S. Jamadagni, K.A. Nagarkar, V.S. Badame, C.R. Chindwin, V.M. Lomte
PY - 2018
DA - 2018/05/31
PB - IJCSE, Indore, INDIA
SP - 387-391
IS - 5
VL - 6
SN - 2347-2693
ER -
![]() |
![]() |
![]() |
575 | 511 downloads | 318 downloads |
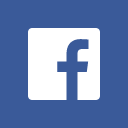
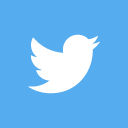
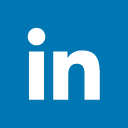
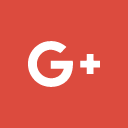
Abstract
Intelligent fault diagnosis is a promising instrument to deal with mechanical big data because of its capacity in quickly and proficiently handling gathered signals and giving exact diagnosis outcomes. Feature extraction is done manually in most of the traditional techniques which required previous knowledge along with diagnostic expertise. Such procedures take favourable position of human inventiveness is tedious and work escalated. The main possibility of unsupervised component discovering that utilization of intelligence systems to learn raw data, a two-stage learning technique is proposed for intelligent analysis of machines. In the first stage vibration signal is utilized to get a grasp on features from mechanical vibration signals. In the next stage, softmax regression is used to classify the health conditions depends on the studied features. The approach is verified by a motor bearing dataset and a locomotive bearing dataset. It can be seen that using this method high diagnosis accuracy can be obtained. Also, the proposed method reduces the need of human labour making it preferable than the existing methods.
Key-Words / Index Term
Mechanical big data, unsupervised feature learning, sparse filtering, softmax regression, intelligent fault diagnosis
References
[1] X. Wu, X. Zhu, G.-Q. Wu, and W. Ding, “Data mining with big data,” IEEE Trans. Knowl. Data Eng., vol. 26, no. 1, pp. 97-107, Jan. 2014.
[2] S. Yin, and O. Kaynak, “Big data for modern industry: challenges and trends,” Proc. IEEE, vol. 103, no. 2, pp. 143-146, Feb. 2015.
[3] W. Qiao, and D. Lu, “A survey on wind turbine condition monitoring and fault diagnosis, ” IEEE Trans. Ind. Electron., vol. 62, no. 10, pp. 6536-6545, Oct. 2015.
[4] Y. Lei, J. Lin, M. J. Zuo, and Z. He, “Condition monitoring and fault diagnosis of planetary gearboxes: A review,” Measurement, vol. 48, pp. 292-305, Feb. 2014.
[5] D. You, X. Gao, and S. Katayama, “WPD-PCA-based laser welding process monitoring and defects diagnosis by using FNN and SVM,” IEEE Trans. Ind. Electron., vol. 62, no. 1, pp. 628-638, Jan. 2015.
[6] Y. Lei, Z. He, Y. Zi, and X. Chen, “New clustering algorithm-based fault diagnosis using compensation distance evaluation technique,” Mech. Syst. Signal Process., vol. 22, no. 2, pp. 419-435, Feb. 2008.
[7] S. Yin, S. X. Ding, X. Xie, and H. Luo, “A review on basic data-driven approaches for industrial process monitoring,” IEEE Trans. Ind. Electron., vol. 61, no. 11, pp. 6418-6428, Nov. 2014.
[8] Y. Lei, Z. He, Y. Zi, and Q. Hu, “Fault diagnosis of rotating machinery based on multiple ANFIS combination with GAs,” Mech. Syst. Signal Process., vol. 21, no. 5, pp. 2280-2294, Jul. 2007.
[9] M. Kang, J. Kim, J.-M. Kim, A. C. Tan, E. Y. Kim, and B.-K. Choi, “Reliable Fault Diagnosis for Low-Speed Bearings Using Individually Trained Support Vector Machines With Kernel Discriminative Feature Analysis,” IEEE Trans. Power Electron., vol. 30, no. 5, pp. 2786-2797, May 2015.
[10] Yaguo Lei, Member, IEEE, Feng Jia, Jing Lin, Saibo Xing, and Steven X. Ding,” An Intelligent Fault Diagnosis Method Using Unsupervised Feature Learning Towards Mechanical Big Data.”, IEEE Transctions on Industrial Electronics, 2016.