Survey on Skin Lesion Analysis towards Melanoma Detection
S. Sreena1 , A. Lijiya2
- Dept. of computer Science and Engineering, National Institute of Technology Calicut, Kerala, India.
- Dept. of computer Science and Engineering, National Institute of Technology Calicut, Kerala, India.
Section:Survey Paper, Product Type: Journal Paper
Volume-6 ,
Issue-5 , Page no. 602-615, May-2018
CrossRef-DOI: https://doi.org/10.26438/ijcse/v6i5.602615
Online published on May 31, 2018
Copyright © S. Sreena, A. Lijiya . This is an open access article distributed under the Creative Commons Attribution License, which permits unrestricted use, distribution, and reproduction in any medium, provided the original work is properly cited.
View this paper at Google Scholar | DPI Digital Library
How to Cite this Paper
- IEEE Citation
- MLA Citation
- APA Citation
- BibTex Citation
- RIS Citation
IEEE Style Citation: S. Sreena, A. Lijiya, “Survey on Skin Lesion Analysis towards Melanoma Detection,” International Journal of Computer Sciences and Engineering, Vol.6, Issue.5, pp.602-615, 2018.
MLA Style Citation: S. Sreena, A. Lijiya "Survey on Skin Lesion Analysis towards Melanoma Detection." International Journal of Computer Sciences and Engineering 6.5 (2018): 602-615.
APA Style Citation: S. Sreena, A. Lijiya, (2018). Survey on Skin Lesion Analysis towards Melanoma Detection. International Journal of Computer Sciences and Engineering, 6(5), 602-615.
BibTex Style Citation:
@article{Sreena_2018,
author = {S. Sreena, A. Lijiya},
title = {Survey on Skin Lesion Analysis towards Melanoma Detection},
journal = {International Journal of Computer Sciences and Engineering},
issue_date = {5 2018},
volume = {6},
Issue = {5},
month = {5},
year = {2018},
issn = {2347-2693},
pages = {602-615},
url = {https://www.ijcseonline.org/full_paper_view.php?paper_id=2028},
doi = {https://doi.org/10.26438/ijcse/v6i5.602615}
publisher = {IJCSE, Indore, INDIA},
}
RIS Style Citation:
TY - JOUR
DO = {https://doi.org/10.26438/ijcse/v6i5.602615}
UR - https://www.ijcseonline.org/full_paper_view.php?paper_id=2028
TI - Survey on Skin Lesion Analysis towards Melanoma Detection
T2 - International Journal of Computer Sciences and Engineering
AU - S. Sreena, A. Lijiya
PY - 2018
DA - 2018/05/31
PB - IJCSE, Indore, INDIA
SP - 602-615
IS - 5
VL - 6
SN - 2347-2693
ER -
![]() |
![]() |
![]() |
890 | 315 downloads | 269 downloads |
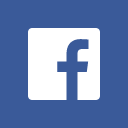
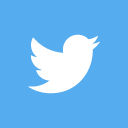
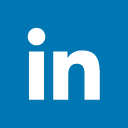
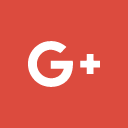
Abstract
Malignant melanoma is the deadliest form of skin cancer. Researches are attempting for the early automatic diagnosis of Melanoma, a lethal form of skin cancer, from dermoscopic images. The process includes different stages like pre-processing, lesion segmentation, dermoscopic feature detection within a lesion, feature extraction and disease classification. In this paper, we review the state-of-the-art computer aided diagnosis system for melanoma detection and examine recent practices in different steps of these systems. Statistics and results from the most important and recent implementations are analyzed and reported. We compared the performance of recent works based on different parameters like accuracy, sensitivity, specificity, machine learning techniques, dataset etc. Research challenges regarding the different parts of computer aided skin cancer diagnosis systems are also highlighted in this paper.
Key-Words / Index Term
Skin cancer, Melanoma, Dermoscopy, Preprocessing, Image segmentation, Feature extraction, Classification, Ridgelet, K-Means, GLCM, SVM
References
[1] David Gutman, Noel C. F. Codella, Emre Celebi, Brian Helba, Michael Marchetti, Nabin Mishra, and Allan Halpern. Skin lesion analysis toward melanoma detection: A challenge at the international symposium on biomedical imaging (ISBI) 2016, hosted by the international skin imaging collaboration (ISIC).CoRR, abs/1605.01397, 2016.
[2] Nurulhuda Firdaus Mohd Azmi, Haslina Md Sarkan, Yazriwati Yahya, and Suriayati Chuprat. Abcd rules segmentation on malignant tumor and benign skin lesion images. In Computer and Information Sciences (ICCOINS), 2016 3rd International Conference on, pages 66–70. IEEE, 2016.
[3] ”International Skin Imaging Collaboration: Melanoma Project Website, ” https://isic-archive. com, note = Accessed: 2010-09-30.
[4] Brian D’Alessandro, Atam P Dhawan, and Nizar Mullani. Computer aided analysis of epi-illumination and transillumination images of skin lesions for diagnosis of skin cancers. In Engineering in Medicine and Biology Society, EMBC, 2011 Annual International Conference of the IEEE, pages 3434–3438. IEEE, 2011.
[5] Wen-Yu Chang, Adam Huang, Chung-Yi Yang, Chien-Hung Lee, Yin- Chun Chen, Tian-Yau Wu, and Gwo-Shing Chen. Computer-aided diagnosis of skin lesions using conventional digital photography: a reliability and feasibility study. PloS one, 8(11):e76212, 2013.
[6] Thanh-Toan Do, Yiren Zhou, Haitian Zheng, Ngai-Man Cheung, and Dawn Koh. Early melanoma diagnosis with mobile imaging. In Engineering in Medicine and Biology Society (EMBC), 2014 36th Annual International Conference of the IEEE, pages 6752–6757. IEEE, 2014.
[7] O. Abuzaghleh, B. D. Barkana, and M. Faezipour. Noninvasive real-time automated skin lesion analysis system for melanoma early detection and prevention. IEEE Journal of Translational Engineering in Health and Medicine, 3:1–12, 2015.
[8] Maria Joa˜o M Vasconcelos, Lu´ıs Rosado, and Ma´rcia Ferreira. A new risk assessment methodology for dermoscopic skin lesion images. In Medical Measurements and Applications (MeMeA), 2015 IEEE Interna- tional Symposium on, pages 570–575. IEEE, 2015.
[9] O. Abuzaghleh, M. Faezipour, and B. D. Barkana. A comparison of feature sets for an automated skin lesion analysis system for melanoma early detection and prevention. In 2015 Long Island Systems, Applica- tions and Technology, pages 1–6, May 2015.
[10] U. Jamil, S. Khalid, and M. U. Akram. Dermoscopic feature analysis for melanoma recognition and prevention. In 2016 Sixth International Conference on Innovative Computing Technology (INTECH), pages 290–295, Aug 2016.
[11] J Premaladha and KS Ravichandran. Novel approaches for diagnosing melanoma skin lesions through supervised and deep learning algorithms. Journal of medical systems, 40(4):96, 2016.
[12] Teck Yan Tan, Li Zhang, and Ming Jiang. An intelligent decision support system for skin cancer detection from dermoscopic images. In Natural Computation, Fuzzy Systems and Knowledge Discovery (ICNC-FSKD),2016 12th International Conference on, pages 2194–2199. IEEE, 2016.
[13] Nazia Hameed, Anglia Ruskin, Kamal Abu Hassan, and MA Hossain. A comprehensive survey on image-based computer aided diagnosis systems for skin cancer. In Software, Knowledge, Information Management & Applications (SKIMA), 2016 10th International Conference on, pages 205–214. IEEE, 2016.
[14] Nguyen Hong Quang et al. Automatic skin lesion analysis towards melanoma detection. In Intelligent and Evolutionary Systems (IES), 2017 21st Asia Pacific Symposium on, pages 106–111. IEEE, 2017.
[15] L. Yu, H. Chen, Q. Dou, J. Qin, and P. A. Heng. Automated melanoma recognition in dermoscopy images via very deep residual networks. IEEE Transactions on Medical Imaging, 36(4):994–1004, April 2017.
[16] Q. Ul Ain, Bing Xue, H. Al-Sahaf, and M. Zhang. Genetic programming for skin cancer detection in dermoscopic images. In 2017 IEEE Congress on Evolutionary Computation (CEC), pages 2420–2427, June 2017.
[17] Sabrina Conoci, Francesco Rundo, Salvatore Petralta, and Sebastiano Battiato. Advanced skin lesion discrimination pipeline for early melanoma cancer diagnosis towards poc devices. In Circuit Theory and Design (ECCTD), 2017 European Conference on, pages 1–4. IEEE, 2017.
[18] Yuexiang Li and Linlin Shen. Skin lesion analysis towards melanoma detection using deep learning network. Sensors, 18(2):556, 2018.
[19] Marcelo Bertalmio, Guillermo Sapiro, Vincent Caselles, and Coloma Ballester. Image inpainting. In Proceedings of the 27th annual conference on Computer graphics and interactive techniques, pages 417–424. ACM Press/Addison-Wesley Publishing Co., 2000.
[20] Jean-Luc Starck, Emmanuel J Cande`s, and David L Donoho. The curvelet transform for image denoising. IEEE Transactions on image processing, 11(6):670–684, 2002.
[21] Tony F Chan, Jianhong Shen, and Hao-Min Zhou. Total variation wavelet inpainting. Journal of Mathematical imaging and Vision, 25(1):107–125, 2006.
[22] APV Ranganathan and RF Von Borries. Sliced ridgelet transform for image denoising. In Digital Signal Processing Workshop, 12th-Signal Processing Education Workshop, 4th, pages 209–213. IEEE, 2006.
[23] Anne Cuzol, Kim Steenstrup Pedersen, and Mads Nielsen. Field of particle filters for image inpainting. Journal of Mathematical Imaging and Vision, 31(2):147–156, 2008.
[24] M Emre Celebi, Hitoshi Iyatomi, Gerald Schaefer, and William V Stoecker. Lesion border detection in dermoscopy images. Computerized medical imaging and graphics, 33(2):148–153, 2009.
[25] Supot Sookpotharom. Border detection of skin lesion images based on fuzzy c-means thresholding. In Genetic and Evolutionary Computing, 2009. WGEC’09. 3rd International Conference on, pages 777–780. IEEE, 2009.
[26] H Noori and Saeid Saryazdi. Image inpainting using directional median filters. In Computational Intelligence and Communication Networks (CICN), 2010 International Conference on, pages 45–49. IEEE, 2010.
[27] Sachin D Ruikar and Dharmpal D Doye. Wavelet based image denoising technique. International Journal of Advanced Computer Science and Applications, 2(3):49–53, 2011.
[28] Sandeep Palakkal and KMM Prabhu. Poisson noise removal from images using the fast discrete curvelet transform. In Communications (NCC), 2011 National Conference on, pages 1–5. IEEE, 2011.
[29] Mohammad Taghi Bahreyni Toossi, Hamid Reza Pourreza, Hoda Zare, Mohamad-Hoseyn Sigari, Pouran Layegh, and Abbas Azimi. An effective hair removal algorithm for dermoscopy images. Skin Research and Technology, 19(3):230–235, 2013.
[30] Adam Huang, Shun-Yuen Kwan, Wen-Yu Chang, Min-Yin Liu, Min- Hsiu Chi, and Gwo-Shing Chen. A robust hair segmentation and removal approach for clinical images of skin lesions. In Engineering in Medicine and Biology Society (EMBC), 2013 35th Annual International Conference of the IEEE, pages 3315–3318. IEEE, 2013.
[31] Santosh Kumar Yadav, Rohit Sinha, and Prabin Kumar Bora. Image denoising using ridgelet transform in a collaborative filtering framework. In Signal Processing and Communications (SPCOM), 2014 International Conference on, pages 1–5. IEEE, 2014.
[32] Azadeh Noori Hoshyar, Adel Al-Jumaily, and Afsaneh Noori Hoshyar. The beneficial techniques in preprocessing step of skin cancer detection system comparing. Procedia Computer Science, 42:25–31, 2014.
[33] Shehzad Khalid and Uzma Jamil. Valuable pre-processing and segmen- tation techniques used in automated skin lesion detection systems. 2015.
[34] Sumit Kumar and Mantosh Biswas. New method of noise removal in images using curvelet transform. In Computing, Communication & Automation (ICCCA), 2015 International Conference on, pages 1193–1197. IEEE, 2015.
[35] Tomas Majtner, Kristına Lidayova, Sule Yildirim-Yayilgan, and Jon Yn- gve Hardeberg. Improving skin lesion segmentation in dermoscopic images by thin artefacts removal methods. In Visual Information Processing (EUVIP), 2016 6th European Workshop on, pages 1–6. IEEE, 2016.
[36] Ph Schmid and S Fischer. Colour segmentation for the analysis of pigmented skin lesions. In Image Processing and Its Applications, 1997., Sixth International Conference on, volume 2, pages 688–692. IET, 1997.
[37] Heng-Da Cheng, X H Jiang, Ying Sun, and Jingli Wang. Color image segmentation: advances and prospects. Pattern recognition, 34(12):2259–2281, 2001.
[38] Wan-Ting Lin, Chuen-Horng Lin, Tsung-Ho Wu, and Yung-Kuan Chan. Image segmentation using the k-means algorithm for texture features. World Academy of Science, Engineering and Technology, 65:612–615, 2010.
[39] Xiang Li, Ben Aldridge, Robert Fisher, and Jonathan Rees. Estimating the ground truth from multiple individual segmentations incorporating prior pattern analysis with application to skin lesion segmentation. In Biomedical Imaging: From Nano to Macro, 2011 IEEE International Symposium on, pages 1438–1441. IEEE, 2011.
[40] R Yogamangalam and B Karthikeyan. Segmentation techniques com- parison in image processing. International Journal of Engineering and Technology (IJET), 5(1):307–313, 2013.
[41] Nameirakpam Dhanachandra, Khumanthem Manglem, and Yambem Jina Chanu. Image segmentation using k-means clustering algorithm and subtractive clustering algorithm. Procedia Computer Science, 54:764–771, 2015.
[42] Fahimeh Sadat Saleh and Reza Azmi. Automatic multiple regions segmentation of dermoscopy images. In Artificial Intelligence and Signal Processing (AISP), 2015 International Symposium on, pages 24–29. IEEE, 2015.
[43] Mohammad H Jafari, Nader Karimi, Ebrahim Nasr-Esfahani, Shadrokh Samavi, S Mohamad R Soroushmehr, K Ward, and Kayvan Najarian. Skin lesion segmentation in clinical images using deep learning. In Pattern Recognition (ICPR), 2016 23rd International Conference on, pages 337–342. IEEE, 2016.
[44] Noel CF Codella, David Gutman, M Emre Celebi, Brian Helba, Michael A Marchetti, Stephen W Dusza, Aadi Kalloo, Konstantinos Liopyris, Nabin Mishra, Harald Kittler, et al. Skin lesion analysis toward melanoma detection: A challenge at the 2017 international symposium on biomedical imaging (isbi), hosted by the international skin imaging collaboration (isic). arXiv preprint arXiv:1710.05006, 2017.
[45] Chiranjeev Sagar and Lalit Mohan Saini. Color channel based segmenta- tion of skin lesion from clinical images for the detection of melanoma. In Power Electronics, Intelligent Control and Energy Systems (ICPEICES), IEEE International Conference on, pages 1–5. IEEE, 2016.
[46] Dao Nam Anh. Detection of lesion region in skin images by moment of patch. In Computing & Communication Technologies, Research, Inno- vation, and Vision for the Future (RIVF), 2016 IEEE RIVF International Conference on, pages 217–222. IEEE, 2016.
[47] Neda Zamani Tajeddin and Babak Mohammadzadeh Asl. A general algorithm for automatic lesion segmentation in dermoscopy images. In Biomedical Engineering and 2016 1st International Iranian Conference on Biomedical Engineering (ICBME), 2016 23rd Iranian Conference on, pages 134–139. IEEE, 2016.
[48] Behzad Bozorgtabar, Zongyuan Ge, Rajib Chakravorty, Mani Abedini, Sergey Demyanov, and Rahil Garnavi. Investigating deep side layers for skin lesion segmentation. In Biomedical Imaging (ISBI 2017), 2017 IEEE 14th International Symposium on, pages 256–260. IEEE, 2017.
[49] Rashika Mishra and Ovidiu Daescu. Deep learning for skin lesion segmentation. In 2017 IEEE International Conference on Bioinformatics and Biomedicine (BIBM), pages 1189–1194. IEEE, 2017.
[50] Yading Yuan, Ming Chao, and Yeh-Chi Lo. Automatic skin lesion segmentation using deep fully convolutional networks with jaccard distance. IEEE Transactions on Medical Imaging, 2017.
[51] Mohamed Attia, Mohamed Hossny, Saeid Nahavandi, and Anousha Yazdabadi. Skin melanoma segmentation using recurrent and convolu- tional neural networks. In Biomedical Imaging (ISBI 2017), 2017 IEEE 14th International Symposium on, pages 292–296. IEEE, 2017.
[52] Oludayo O Olugbara, Tunmike B Taiwo, and Delene Heukelman. Segmentation of melanoma skin lesion using perceptual color differ- ence saliency with morphological analysis. Mathematical Problems in Engineering, 2018, 2018.
[53] Miroslav Bencˇo and Robert Hudec. Novel method for color textures features extraction based on glcm. Radioengineering, 16(4):65, 2007.
[54] Giuseppe Di Leo, Gabriella Fabbrocini, Alfredo Paolillo, Orsola Rescigno, and Paolo Sommella. Towards an automatic diagnosis system for skin lesions: estimation of blue-whitish veil and regression structures. In Systems, Signals and Devices, 2009. SSD’09. 6th International Multi- Conference on, pages 1–6. IEEE, 2009.
[55] R Sumithra, Mahamad Suhil, and DS Guru. Segmentation and classifi- cation of skin lesions for disease diagnosis. Procedia Computer Science, 45:76–85, 2015.
[56] Omar Abuzaghleh, Buket D Barkana, and Miad Faezipour. Automated skin lesion analysis based on color and shape geometry feature set for melanoma early detection and prevention. In Systems, Applications and Technology Conference (LISAT), 2014 IEEE Long Island, pages 1–6. IEEE, 2014.
[57] Sinan Kockara, Mutlu Mete, Tansel Halic, Nurcan Yuruk, Muhyeddin Ercan, and Ashley Lawrence. Fractals for malignancy detection in der- moscopy images. In Healthcare Informatics (ICHI), 2015 International Conference on, pages 115–121. IEEE, 2015.
[58] M Suryapraba, G Rajanarayanee, Priyanka Kumari, and P Santhi. Analysis of skin cancer classification using glcm based on feature extraction in artificial neural network. International Journal of Emerging Technology in Computer Science & Electronics, 13.
[59] Seema Kolkur and DR Kalbande. Survey of texture based feature extraction for skin disease detection. In ICT in Business Industry & Government (ICTBIG), International Conference on, pages 1–6. IEEE, 2016.
[60] Md Khalad Abu Mahmoud, Adel Al-Jumaily, and Maen Takruri. The automatic identification of melanoma by wavelet and curvelet analysis: study based on neural network classification. In Hybrid Intelligent Systems (HIS), 2011 11th International Conference on, pages 680–685. IEEE, 2011.
[61] Bareqa Salah, Mohammad Alshraideh, Rasha Beidas, and Ferial Haya- jneh. Skin cancer recognition by using a neuro-fuzzy system. Cancer informatics, 10:CIN–S5950, 2011.
[62] Maen Takruri, Adel Al-Jumaily, and Mohamed Khaled Abu Mahmoud. Automatic recognition of melanoma using support vector machines: A study based on wavelet, curvelet and color features. In Industrial Au- tomation, Information and Communications Technology (IAICT), 2014 International Conference on, pages 70–75. IEEE, 2014.
[63] Diwakar Gautam and Mushtaq Ahmed. Melanoma detection and classi- fication using svm based decision support system. In India Conference (INDICON), 2015 Annual IEEE, pages 1–6. IEEE, 2015.
[64] RS Shiyam Sundar and M Vadivel. Performance analysis of melanoma early detection using skin lession classification system. In Circuit, Power and Computing Technologies (ICCPCT), 2016 International Conference on, pages 1–5. IEEE, 2016.
[65] Muhammad Ali Farooq, Muhammad Aatif Mobeen Azhar, and Rana Hammad Raza. Automatic lesion detection system (alds) for skin cancer classification using svm and neural classifiers. In Bioinformatics and Bioengineering (BIBE), 2016 IEEE 16th International Conference on, pages 301–308. IEEE, 2016.
[66] Wiem Abbes and Dorra Sellami. High-level features for automatic skin lesions neural network based classification. In Image Processing, Applications and Systems (IPAS), 2016 International, pages 1–7. IEEE, 2016.
[67] Adria Romero Lopez, Xavier Giro-i Nieto, Jack Burdick, and Oge Marques. Skin lesion classification from dermoscopic images using deep learning techniques. In Biomedical Engineering (BioMed), 2017 13th IASTED International Conference on, pages 49–54. IEEE, 2017.
[68] Saeid Amouzad Mahdiraji, Yasser Baleghi, and Sayed Mahmoud Sakhaei. Skin lesion images classification using new color pigmented boundary descriptors. In Pattern Recognition and Image Analysis (IPRIA), 2017 3rd International Conference on, pages 102–107. IEEE, 2017.
[69] A Rajesh. Classification of malignant melanoma and benign skin lesion by using back propagation neural network and abcd rule. In Electrical, Instrumentation and Communication Engineering (ICEICE), 2017 IEEE International Conference on, pages 1–8. IEEE, 2017.
[70] S Chatterjee, D Dey, and S Munshi. Development of a superpixel based local color feature extraction technique for the classification of skin lesions. In Calcutta Conference (CALCON), 2017 IEEE, pages 210–214. IEEE, 2017.
[71] Hiam Alquran, Isam Abu Qasmieh, Ali Mohammad Alqudah, Sajidah Alhammouri, Esraa Alawneh, Ammar Abughazaleh, and Firas Hasayen. The melanoma skin cancer detection and classification using support vector machine. In Applied Electrical Engineering and Computing Technologies (AEECT), 2017 IEEE Jordan Conference on, pages 1–5. IEEE, 2017.
[72] Youssef Filali, Assia Ennouni, My Abdelouahed Sabri, and Abdellah Aarab. Multiscale approach for skin lesion analysis and classification. In Advanced Technologies for Signal and Image Processing (ATSIP),2017 International Conference on, pages 1–6. IEEE, 2017.
[73] Bill S Lin, Kevin Michael, Shivam Kalra, and HR Tizhoosh.Skin lesion segmentation: U-nets versus clustering. arXiv preprint arXiv:1710.01248, 2017.
[74] Nay Chi Lynn and Zin Mar Kyu. Segmentation and classification of skin cancer melanoma from skin lesion images. In Parallel and Distributed Computing, Applications and Technologies (PDCAT), 2017, 18th International Conference on, pages 117–122. IEEE, 2017.
[75] MA Rahman, MT Haque, C Shahnaz, SA Fattah, WP Zhut, and MO Ahmedt. Skin lesions classification based on color plane-histogram- image quality analysis features extracted from digital images. 2017.
[76] Ivan Gonzalez Diaz. Dermaknet: Incorporating the knowledge of der- matologists to convolutional neural networks for skin lesion diagnosis. IEEE Journal of Biomedical and Health Informatics, 2018.
[77] Roberta B Oliveira, Joa˜o P Papa, Aledir S Pereira, and Joa˜o Manuel RS Tavares. Computational methods for pigmented skin lesion classification in images: review and future trends. Neural Computing and Applica- tions, 29(3):613–636, 2018.