Classification of Chronic Kidney Disease using Feature Selection Techniques
A. K. Shrivas1 , Sanat Kumar Sahu2
- Dept. of IT, Dr. C. V. Raman University, Bilaspur (C.G.), India.
- Dept. of Computer Science, Govt. Kaktiya P.G. College, Jagdalpur (C.G.), India.
Section:Research Paper, Product Type: Journal Paper
Volume-6 ,
Issue-5 , Page no. 649-653, May-2018
CrossRef-DOI: https://doi.org/10.26438/ijcse/v6i5.649653
Online published on May 31, 2018
Copyright © A. K. Shrivas, Sanat Kumar Sahu . This is an open access article distributed under the Creative Commons Attribution License, which permits unrestricted use, distribution, and reproduction in any medium, provided the original work is properly cited.
View this paper at Google Scholar | DPI Digital Library
How to Cite this Paper
- IEEE Citation
- MLA Citation
- APA Citation
- BibTex Citation
- RIS Citation
IEEE Citation
IEEE Style Citation: A. K. Shrivas, Sanat Kumar Sahu, “Classification of Chronic Kidney Disease using Feature Selection Techniques,” International Journal of Computer Sciences and Engineering, Vol.6, Issue.5, pp.649-653, 2018.
MLA Citation
MLA Style Citation: A. K. Shrivas, Sanat Kumar Sahu "Classification of Chronic Kidney Disease using Feature Selection Techniques." International Journal of Computer Sciences and Engineering 6.5 (2018): 649-653.
APA Citation
APA Style Citation: A. K. Shrivas, Sanat Kumar Sahu, (2018). Classification of Chronic Kidney Disease using Feature Selection Techniques. International Journal of Computer Sciences and Engineering, 6(5), 649-653.
BibTex Citation
BibTex Style Citation:
@article{Shrivas_2018,
author = {A. K. Shrivas, Sanat Kumar Sahu},
title = {Classification of Chronic Kidney Disease using Feature Selection Techniques},
journal = {International Journal of Computer Sciences and Engineering},
issue_date = {5 2018},
volume = {6},
Issue = {5},
month = {5},
year = {2018},
issn = {2347-2693},
pages = {649-653},
url = {https://www.ijcseonline.org/full_paper_view.php?paper_id=2036},
doi = {https://doi.org/10.26438/ijcse/v6i5.649653}
publisher = {IJCSE, Indore, INDIA},
}
RIS Citation
RIS Style Citation:
TY - JOUR
DO = {https://doi.org/10.26438/ijcse/v6i5.649653}
UR - https://www.ijcseonline.org/full_paper_view.php?paper_id=2036
TI - Classification of Chronic Kidney Disease using Feature Selection Techniques
T2 - International Journal of Computer Sciences and Engineering
AU - A. K. Shrivas, Sanat Kumar Sahu
PY - 2018
DA - 2018/05/31
PB - IJCSE, Indore, INDIA
SP - 649-653
IS - 5
VL - 6
SN - 2347-2693
ER -
![]() |
![]() |
![]() |
590 | 260 downloads | 242 downloads |
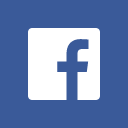
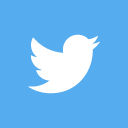
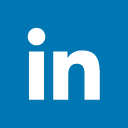
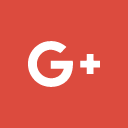
Abstract
Classification and features selection play very important role to develop robust and computationally efficient model. In this paper, we have compared different classification techniques for classification of chronic kidney disease data. Two supervised classification learning algorithms are used to develop classifiers as Multilayer Perceptron Network (MLPN) and Radial Base Function Network (RBFN). The main focus of this research work is to reduce the number of features using different feature selection technique. We have also used five different classification techniques for select the relevant feature subsets and improve the accuracy of the classification through the Feature Selection Technique (FST). The RBFN classifier achieved the highest average percentage of performance in terms of accuracy. The results shows that both classification techniques given satisfactory accuracy rate in each different selected feature subset.
Key-Words / Index Term
MLP, RBFN,CKD, Feature Selection Techniques
References
[1] M. Kumar, “Prediction of Chronic Kidney Disease Using Random Forest Machine Learning Algorithm,” Int. J. Comput. Sci. Mob. Comput., vol. 5, no. 2, pp. 24–33, 2016.
[2] J. Han, M. Kamber, and J. Pei, Data mining: concepts and techniques, Third. Elsevier, 2012.
[3] J. Novakovic, P. Strbac, and D. Bulatovic, “Toward optimal feature selection using ranking methods and classification algorithms,” Yugosl. J. Oper. Res., vol. 21, no. 1, pp. 119–135, 2011.
[4] D. N. R. S.Ramya, “Diagnosis of Chronic Kidney Disease Using Machine Learning Algorithms,” Int. J. Innov. Res. Comput. Commun. Eng., vol. 4, no. 1, pp. 812–820, 2016.
[5] M. Arora and E. A. Sharma, “Chronic Kidney Disease Detection by Analyzing Medical Datasets in Weka,” Int. J. Comput. Appl., vol. 6, no. 4, pp. 20–26, 2016.
[6] P. sinha; P. Sinha, “Comparative Study of Chronic Kidney Disease Prediction using KNN and SVM,” vol. 4, no. 12, pp. 608–612, 2015.
[7] A. Marcano-Cedeño, P. Chausa, A. García, C. Cáceres, J. M. Tormos, and E. J. Gómez, “Data mining applied to the cognitive rehabilitation of patients with acquired brain injury,” Expert Syst. Appl., vol. 40, no. 4, pp. 1054–1060, 2013.
[8] D. Oreski and T. Novosel, “Comparison of Feature Selection Techniques in Knowledge Discovery Process,” vol. 3, no. 4, pp. 285–290, 2014.
[9] S. I. Ali and W. Shahzad, “A feature subset selection method based on symmetric uncertainty and Ant Colony Optimization,” pp. 1–6, 2012.
[10] Sivanandam and Deepa, Principles of Soft Computing, Second. wiley, 2014.
[11] S. Haykin, Neural Networks and Learning Machines, vol. 3. 2008.
[12] R. Kala, H. Vazirani, N. Khanwalkar, and M. Bhattacharya, “Evolutionary radial basis function network for classificatory problems,” Int. J. Comput. Sci. Appl., vol. 7, no. 4, pp. 34–49, 2010.
[13] “UCI Machine Learning Repository of machine learning databases,” 2015. [Online]. Available: https://archive.ics.uci.edu/ml/datasets/Chronic_Kidney_Disease. [Accessed: 01-Jan-2016].