Improving Mediterm Classification in Medical Subject Headings (MeSH)
R. Aravazhi1 , M. Chidambaram2
- Department of Computer Science, A.V.V.M Sri Pushpam College (Autonomous), Poondi, Thanjavur, India.
- Department of Computer Science, Rajah Serfoji Government College (Autonomous), Thanjavur, India.
Section:Review Paper, Product Type: Journal Paper
Volume-6 ,
Issue-5 , Page no. 821-825, May-2018
CrossRef-DOI: https://doi.org/10.26438/ijcse/v6i5.821825
Online published on May 31, 2018
Copyright © R. Aravazhi, M. Chidambaram . This is an open access article distributed under the Creative Commons Attribution License, which permits unrestricted use, distribution, and reproduction in any medium, provided the original work is properly cited.
View this paper at Google Scholar | DPI Digital Library
How to Cite this Paper
- IEEE Citation
- MLA Citation
- APA Citation
- BibTex Citation
- RIS Citation
IEEE Citation
IEEE Style Citation: R. Aravazhi, M. Chidambaram, “Improving Mediterm Classification in Medical Subject Headings (MeSH),” International Journal of Computer Sciences and Engineering, Vol.6, Issue.5, pp.821-825, 2018.
MLA Citation
MLA Style Citation: R. Aravazhi, M. Chidambaram "Improving Mediterm Classification in Medical Subject Headings (MeSH)." International Journal of Computer Sciences and Engineering 6.5 (2018): 821-825.
APA Citation
APA Style Citation: R. Aravazhi, M. Chidambaram, (2018). Improving Mediterm Classification in Medical Subject Headings (MeSH). International Journal of Computer Sciences and Engineering, 6(5), 821-825.
BibTex Citation
BibTex Style Citation:
@article{Aravazhi_2018,
author = {R. Aravazhi, M. Chidambaram},
title = {Improving Mediterm Classification in Medical Subject Headings (MeSH)},
journal = {International Journal of Computer Sciences and Engineering},
issue_date = {5 2018},
volume = {6},
Issue = {5},
month = {5},
year = {2018},
issn = {2347-2693},
pages = {821-825},
url = {https://www.ijcseonline.org/full_paper_view.php?paper_id=2070},
doi = {https://doi.org/10.26438/ijcse/v6i5.821825}
publisher = {IJCSE, Indore, INDIA},
}
RIS Citation
RIS Style Citation:
TY - JOUR
DO = {https://doi.org/10.26438/ijcse/v6i5.821825}
UR - https://www.ijcseonline.org/full_paper_view.php?paper_id=2070
TI - Improving Mediterm Classification in Medical Subject Headings (MeSH)
T2 - International Journal of Computer Sciences and Engineering
AU - R. Aravazhi, M. Chidambaram
PY - 2018
DA - 2018/05/31
PB - IJCSE, Indore, INDIA
SP - 821-825
IS - 5
VL - 6
SN - 2347-2693
ER -
![]() |
![]() |
![]() |
528 | 330 downloads | 235 downloads |
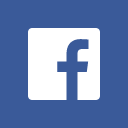
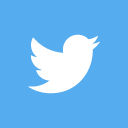
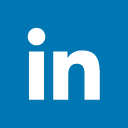
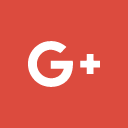
Abstract
A standout amongst the most difficult activities in data frameworks is separating data from unstructured writings, including medical archive classification. A classification calculation that arranges a medical record by examining its substance and classifying it under predefined themes from the Medical Subject Headings (MeSH). It gathered a corpus of 50 full-content diary articles (N=50) from MEDLINE, which were at that point ordered by specialists in light of MeSH. Utilizing natural language processing (NLP), the calculation orders the gathered articles under MeSH subject headings. It assessed the calculation`s result by estimating its accuracy and review of coming about subject headings from the calculation, contrasting outcomes with the real archives` subject headings. The calculation ordered the articles effectively under 45% to 60% of the genuine subject headings and got 40% to 53% of the aggregate subject headings rectify. This holds promising answers for the worldwide wellbeing field to file and arrange medical archives quickly.
Key-Words / Index Term
MeSH, Natural Language Processing, MEDLINE, Classification
References
[1] G. Fabian, T. Wachter, and M. Schroeder, “Extending ontologies by finding siblings using set expansion techniques,” Bioinformatics, vol. 28, no. 12, pp. I292–I300, 2012.
[2] O. Bodenreider, T. C. Rindflesch, and A. Burgun, “Unsupervised, corpus-based method for extending a biomedical terminology,” in Proc. ACL-02 Workshop Natural Language Process. Biomed. Domain, Phildadelphia, PA, USA, 2002, vol. 3, pp. 53–60.
[3] Illhoi Yoo, Xiaohua Hu, “Biomedical Ontology MeSH Improves Document Clustering Qualify on MEDLINE Articles: A Comparison Study”, 19th IEEE Symposium on Computer-Based Medical Systems (CBMS`06), pp. 577 – 582, 2006.
[4] A.Kogilavani, B. Dr.P.Balasubramanie, “Ontology Enhanced Clustering Based Summarization of Medical Documents”, International Journal of Recent Trends in Engineering, Vol. 1, No. 1, May 2009.
[5] H. a. N. Al-Mubaid, A., "Measuring semantic similarity between biomedical concepts within multiple ontologies," IEEE Transactions on Systems, Man, and Cybernetics, Part C: Applications and Reviews, vol. 39, pp. 389–398, 2009.
[6] Shengwen Peng, Ronghui You, Hongning Wang, Chengxiang Zhai, Hiroshi Mamitsuka, Shanfeng Zhu, DeepMeSH: deep semantic representation for improving large-scale MeSH
[7] O. Bodenreider and R. Stevens, “Bio-ontologies: Current trends and future directions,” Brief. Bioinform., vol. 7, no. 3, pp. 256–74, 2006.
[8] H. Al-Mubaid and H. A. Nguyen, “A cluster-based approach for semantic similarity in the biomedical domain,” in Conf. Proc. IEEE Eng. Med. Biol. Soc., 2006, vol. 1, pp. 2713–7.
[9] Yu-Wen Guo, Yi-Tsung Tang, Hung-Yu Kao, “Genealogical-Based Method for Multiple Ontology Self-Extension in MeSH”, IEEE Transactions on NanoBioscience, Vol. 13, No. 2, pp. 124 – 130, 2014
[10] Ahmed Al-Saadi, Rossitza Setchi, Yulia Hicks, “Semantic Reasoning in Cognitive Networks for Heterogeneous Wireless Mesh Systems”, IEEE Transactions on Cognitive Communications and Networking, Vol. 3, No. 3, pp. 374 – 389, 2017.
[11] Pandey, Hari, “Review on Web Content Mining Techniques”, International Journal of Computer Applications. Vol. 118. 33-36. 10.5120/20848-3536.
[12] H.A, "Semantic similarity measures in the MESH ontology and their application to information retrieval on medline," Diploma Thesis, Dept. of Electronic and Computer Engineering, Technical Univ. of Crete (TUC), Crete, Greece, 2005.
[13] Xin Li, José-Fernán Martínez, Gregorio Rubio, “A New Fuzzy Ontology Development Methodology (FODM) Proposal”, IEEE Access, Vol. 4, pp. 7111 – 7124, 2016.
[14] Joseph, Sethunya & Sedimo, Kutlwano & Kaniwa, Freeson & Hlomani, Hlomani & Letsholo, Keletso. (2016). Natural Language Processing: A Review. Natural Language Processing: A Review. 6. 207-210.
[15] Morota G, Beissinger TM, Peñagaricano F. MeSH-Informed Enrichment Analysis and MeSH-Guided Semantic Similarity Among Functional Terms and Gene Products in Chicken. G3: Genes|Genomes|Genetics. 2016, 6(8):2447-2453. doi:10.1534/g3.116.031096.
[16] T. C. Rindflesch, J. V. Rajan, and L. Hunter, “Extracting molecular binding relationships from biomedical text,” in Proc. 6th Appl. Natural Language Process. Conf./1st Meet. North Amer. Chapter Assoc. Comput. Linguistics, Proc. Conf. and Proc. Anlp-Naacl 2000 Student Res. Workshop, 2000, pp. 188–195.
[17] D. Sanchez and M. Batet, “Semantic similarity estimation in the biomedical domain: An ontology-based information-theoretic perspective,” J. Biomed. Inform., vol. 44, no. 5, pp. 749–759, 2011.