Hybrid Recommender Systems: Process, Challenges, Approaches and Metrics
K.Reka 1 , T.N.Ravi 2
- Department of Computer Science, Cauvery College For Women, Trichy, TamilNadu, India.
- Department of Computer Science, Periyar E.V.R College, Trichy, TamilNadu, India.
Section:Research Paper, Product Type: Journal Paper
Volume-6 ,
Issue-5 , Page no. 1024-1033, May-2018
CrossRef-DOI: https://doi.org/10.26438/ijcse/v6i5.10241033
Online published on May 31, 2018
Copyright © K.Reka, T.N.Ravi . This is an open access article distributed under the Creative Commons Attribution License, which permits unrestricted use, distribution, and reproduction in any medium, provided the original work is properly cited.
View this paper at Google Scholar | DPI Digital Library
How to Cite this Paper
- IEEE Citation
- MLA Citation
- APA Citation
- BibTex Citation
- RIS Citation
IEEE Citation
IEEE Style Citation: K.Reka, T.N.Ravi, “Hybrid Recommender Systems: Process, Challenges, Approaches and Metrics,” International Journal of Computer Sciences and Engineering, Vol.6, Issue.5, pp.1024-1033, 2018.
MLA Citation
MLA Style Citation: K.Reka, T.N.Ravi "Hybrid Recommender Systems: Process, Challenges, Approaches and Metrics." International Journal of Computer Sciences and Engineering 6.5 (2018): 1024-1033.
APA Citation
APA Style Citation: K.Reka, T.N.Ravi, (2018). Hybrid Recommender Systems: Process, Challenges, Approaches and Metrics. International Journal of Computer Sciences and Engineering, 6(5), 1024-1033.
BibTex Citation
BibTex Style Citation:
@article{_2018,
author = {K.Reka, T.N.Ravi},
title = {Hybrid Recommender Systems: Process, Challenges, Approaches and Metrics},
journal = {International Journal of Computer Sciences and Engineering},
issue_date = {5 2018},
volume = {6},
Issue = {5},
month = {5},
year = {2018},
issn = {2347-2693},
pages = {1024-1033},
url = {https://www.ijcseonline.org/full_paper_view.php?paper_id=2103},
doi = {https://doi.org/10.26438/ijcse/v6i5.10241033}
publisher = {IJCSE, Indore, INDIA},
}
RIS Citation
RIS Style Citation:
TY - JOUR
DO = {https://doi.org/10.26438/ijcse/v6i5.10241033}
UR - https://www.ijcseonline.org/full_paper_view.php?paper_id=2103
TI - Hybrid Recommender Systems: Process, Challenges, Approaches and Metrics
T2 - International Journal of Computer Sciences and Engineering
AU - K.Reka, T.N.Ravi
PY - 2018
DA - 2018/05/31
PB - IJCSE, Indore, INDIA
SP - 1024-1033
IS - 5
VL - 6
SN - 2347-2693
ER -
![]() |
![]() |
![]() |
736 | 471 downloads | 305 downloads |
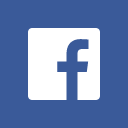
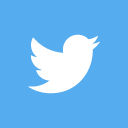
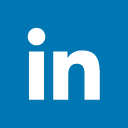
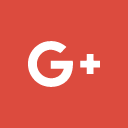
Abstract
Recommender systems plays a significant role, by providing personalized information to users over the internet. With the evolution of the internet, the recommender systems too have evolved from being based on simple demographics, user and item information, into complex hybrid models capable of providing an effective real-time recommendation on a per-user basis. This works provides an overview of traditional recommender system approaches, their taxonomy and discusses the various hybridization techniques used for creating complex models that provide hyper-personalized recommendations. A detailed discussion of the research challenges and how they impact the performance of the various recommender models have been presented as a solution to the existing issues in recommendation systems. Metrics for evaluation and the need for diversity and novelty in recommender systems have also been discussed. Future research directions concerning mobile and IoT based, context-aware recommender systems and the effectiveness of Deep Learning models and how Transfer Learning could address the major drawbacks of recommender systems have also been discussed.
Key-Words / Index Term
Recommender Systems; Hybrid Filtering; Collaborative Filtering; Content-Based Recommendation; Context-Aware Recommender; Demographic Filtering
References
[1] S.K. Lee, Y.H. Cho and S.H. Kim, “Collaborative filtering with ordinal scale-based implicit ratings for mobile music recommendations,” Information Sciences. Vol. 180 (11) ,2010, pp. 2142–2155.
[2] K. Choi, D. Yoo, G. Kim and Y. Suh, “A hybrid online-product recommendation system: combining implicit rating-based collaborative filtering and sequential pattern analysis,” Electronic Commerce Research and Applications, in press, doi: 10.1016/j.elerap.2012.02.004.
[3] E.R. Núñez-Valdéz, J.M. Cueva-Lovelle, O. Sanjuán-Martı´nez, V. Garcı ´ a-Dı´az, P. Ordoñez and C.E. Montenegro-Marı´ n, “Implicit feedback techniques on recommender systems applied to electronic books,” Computers in Human Behavior. Vol. 28 (4) ,2012, pp. 1186–1193.
[4] D. Kotkov, S. Wang and J.Veijalainen, “A survey of serendipity in recommender systems,” Knowledge-Based Systems, 111, 2016, pp.180-192.
[5] D.H. Park, H.K. Kim, I.Y. Choi and J.K. Kim, “A literature review and classification of recommender Systems research,” Expert Systems with Applications 39 ,2012, pp. 10059–10072.
[6] S.K. Lee, Y.H. Cho and S.H. Kim, “Collaborative filtering with ordinal scale-based implicit ratings for mobile music recommendations,” Information Sciences. Vol. 180 (11) ,2010, pp. 2142–2155.
[7] A. Nanolopoulus, D. Rafailidis, P. Symeonidis and Y. Manolopoulus, “Music Box: personalizad music recommendation based on cubic analysis of social tags,” IEEE Transactions on Audio, Speech and Language Processing. Vol. 18 (2) ,2010, pp. 407–412.
[8] S. Tan, J. Bu, CH. Chen and X. He, “Using rich social media information for music recommendation via hypergraph model,” ACM Transactions on Multimedia Computing, Communications, and Applications. Vol. 7 (1) 2011. Article 7.
[9] W. Carrer-Neto, M.L. Hernández-Alcaraz, R. Valencia-Garcı´ a and F. Garcı´a- Sánchez, “Social knowledge-based recommender system,” Application to the movies domain. Expert Systems with Applications. Vol. 39 (12) ,2012, pp. 10990–11000.
[10] P. Winoto and T.Y. Tang, “The role of user mood in movie recommendations,” Expert Systems with Applications. Vol. 37 (8), 2010, pp. 6086–6092.
[11] E.R. Núñez-Valdéz, J.M. Cueva-Lovelle, O. Sanjuán-Martı´nez, V. Garcı ´ a-Dı´az, P. Ordoñez and C.E. Montenegro-Marı´ n, “Implicit feedback techniques on recommender systems applied to electronic books,” Computers in Human Behavior. Vol. 28 (4) ,2012, pp. 1186–1193.
[12] R. González-Crespo, O. Sanjuán-Martı´nez, J. Manuel-Cueva, B. Cristina-Pelayo, J.E. Labra-Gayo and P. Ordoñez, “Recommendation system based on user interaction data applied to intelligent electronic books,” Computers in Human Behavior. Vol. 27 (4), 2011, pp.1445–1449.
[13] Z. Huang, D. Zeng and H. Chen, “A comparison of collaborative filtering recommendation algorithms for e-commerce,” IEEE Intelligent Systems. Vol. 22 (5), 2007, pp. 68–78.
[14] J.J. Castro-Sanchez, R. Miguel, D. Vallejo and L.M. López-López, “A highly adaptive recommender system based on fuzzy logic for B2C e-commerce portals,” Expert Systems with Applications. Vol. 38 (3),2011, pp. 2441–2454.
[15] J. Serrano-Guerrero, E. Herrera-Viedma, J.A. Olivas, A. Cerezo and F.P. Romero, “A google wave-based fuzzy recommender system to disseminate information in University Digital Libraries 2.0,” Information Sciences. vol. 181 (9), 2011, pp. 1503–1516.
[16] C. Porcel and E. Herrera-Viedma, “Dealing with incomplete information in a fuzzy linguistic recommender system to disseminate information in university digital libraries,” Knowledge-Based Systems 23 (1), 2010, pp. 32–39.
[17] K. Mcnally, M.P. O’mahony, M. Coyle, P. Briggs and B. Smyth, “A case study of collaboration and reputation in social web search,” ACM Transactions on Intelligent Systems and Technology. Vol. 3 (1) ,2011. Article 4.
[18] M. Pazzani, “A framework for collaborative, content-based, and demographic filtering,” Artificial Intelligence Review-Special Issue on Data Mining on the Internet. Vol.13 (5-6), 1999, pp. 393–408.
[19] J.S. Breese, D. Heckerman and C. Kadie, “Empirical analysis of predictive algorithms for collaborative filtering,” in: 14th Conference on Uncertainty in Artificial Intelligence, 1998, pp. 43–52.
[20] P. Resnick and H.R. Varian, “Recommender systems,” Communications of the ACM 40 ,1997, pp. 56–58.
[21] J.L. Herlocker, J.A. Konstan and J.T. Riedl, “An empirical analysis of design choices in neighborhood-based collaborative filtering algorithms,” Information Retrieval 5 , 2002, pp. 287–310.
[22] G. Adomavicius and A. Tuzhilin, “Toward the next generation of recommender systems: a survey of the state-of-the-art and possible extensions,” IEEE Transactions on Knowledge and Data Engineering 17 ,2005, pp. 734–749.
[23] J.B. Schafer, D. Frankowski, J. Herlocker and S. Sen, “Collaborative filtering recommender systems,” in: P. Brusilovsky, A. Kobsa,W. Nejdl (Eds.), The Adaptive Web, Springer, Berlin Heidelberg 2007, pp. 291–324.
[24] L. Boratto, S. Carta, G. Fenu and R. Saia, “Semantics-aware content-based recommender systems: Design and architecture guidelines,” Neurocomputing, Vol. 254, pp. 79-85, September 6, 2017.
[25] “Diversity in recommender systems – A survey Matevž Kunaver, Tomaž Požrl, Knowledge-Based Systems,” Vol. 123, pp. 154-162, 1 May 2017.
[26] D. Ben-Shimon, A. Tsikinovsky, L. Rokach, A. Meisles, G. Shani and L. Naamani, “Recommender system from personal social networks,” Advances in Intelligent Web Mastering, Springer, 2007, pp. 47–55.
[27] W. Woerndl,M. Brocco and R. Eigner, “Context-aware recommender systems inmobile scenarios,” International Journal of Information Technology andWeb Engineering 4 ,2009, pp. 67–85.
[28] K. Verbert, N. Manouselis, X. Ochoa, M. Wolpers, H. Drachsler, I. Bosnic and E. Duval, “Context-aware recommender systems for learning: a survey and future challenges,” IEEE Transactions on Learning Technologies 5 ,2012, pp. 318–335.
[29] S. Middleton, D. Roure and N. Shadbolt, “Ontology-based recommender systems,” in: S. Staab, R. Studer (Eds.), Handbook on Ontologies, Springer, Berlin Heidelberg 2009, pp. 779–796.
[30] B. Smyth, “Case-based recommendation,” in: P. Brusilovsky, A. Kobsa, W. Nejdl (Eds.), The Adaptive Web, Springer, Berlin Heidelberg 2007, pp. 342–376.
[31] X. Amatriain, A. Jaimes, N. Oliver and J. Pujol, “Data mining methods for recommender systems,” in: F. Ricci, L. Rokach, B. Shapira, P.B. Kantor (Eds.), Recommender Systems Handbook, Springer, US 2011, pp. 39–71.
[32] S. Hsu, M.-H.Wen, H.-C. Lin, C.-C. Lee and C.-H. Lee, “AIMED— a personalized TV recommendation system,” in: P. Cesar, K. Chorianopoulos, J. Jensen (Eds.), Interactive TV: a Shared Experience, Springer, Berlin Heidelberg 2007, pp. 166–174.
[33] A. Jameson, B. Smyth, “Recommendation to groups,” in: P. Brusilovsky, A. Kobsa, W. Nejdl (Eds.), The Adaptive Web, Methods and Strategies of Web Personalization, Springer, Berlin Heidelberg 2007, pp. 596–627.
[34] I. Garcia and L. Sebastia, “A negotiation framework for heterogeneous group recommendation,” Expert Systems with Applications 41 ,2014, pp. 1245–1261.
[35] P. De Meo, G. Quattrone and D. Ursino, “A decision support system for designing new services tailored to citizen profiles in a complex and distributed e-government scenario,” Data and Knowledge Engineering 67 ,2008, pp. 161–184.
[36] B. Esteban, Á. Tejeda-Lorente, C. Porcel, M. Arroyo and E. Herrera-Viedma, “TPLUFIBWEB: a fuzzy linguistic Web system to help in the treatment of low back pain problems,” Knowledge-Based Systems 2014, http://dx.doi.org/10.1016/j.knosys.2014.03.004.
[37] C. Cornelis, X. Guo, J. Lu and G. Zhang, “A fuzzy relational approach to event recommendation, Proceedings of the Second Indian International Conference on Artificial Intelligence (IICAI-05),” Pune, INDIA 2005, pp. 2231–2242.
[38] J. Lu, Q. Shambour, Y. Xu, Q. Lin and G. Zhang, “BizSeeker: a hybrid semantic recommendation system for personalized government-to-business e-services,” Internet Research 20 ,2010, pp. 342–365.
[39] T. Lee, J. Chun, J. Shim and S.-g. Lee, “An ontology-based product recommender system for B2B marketplaces,” International Journal of Electronic Commerce 11 ,2006, pp. 125–155.
[40] J.-C. Wang and C.-C. Chiu, “Recommending trusted online auction sellers using social network analysis,” Expert Systems with Applications 34, 2008, pp. 1666–1679.
[41] J. Bobadilla, F. Ortega, A. Hernando and A. Gutiérrez, “Recommender systems survey,” Knowledge-Based Systems 46 ,2013, pp. 109–132.
[42] R. Meteren and M. Someren, “Using content-based filtering for recommendation,” in: Proceedings of ECML 2000 Workshop: Maching Learning in Information Age, 2000, pp. 47–56.
[43] J. Mooney and L. Roy, “Content-based book recommending using learning for text categorization,” in: Proceedings of the Fifth ACM Conference on Digital Libraries, 2000, pp. 195–204.
[44] J.L. Herlocker, J.A. Konstan, A.L. Borchers and J.T. Riedl, “An algorithmic framework for performing collaborative filtering,” in: Proceedings of the 22nd Annual International ACM SIGIR Conference on Research and Development in Information Retrieval, 1999, pp. 230–237.
[45] J.L. Herlocker, J.A. Konstan, J.T. Riedl and L.G. Terveen, “Evaluating collaborative filtering recommender systems,” ACM Transactions on Information Systems. Vol. 22 (1) ,2004 pp. 5–53.
[46] J. Bobadilla, F. Ortega and A. Hernando, “A collaborative filtering similarity measure based on singularities,” Information Processing and Management. Vol. 48 (2) ,2012, pp. 204–217.
[47] B. Krulwich, “Lifestyle finder: intelligent user profiling using large-scale demographic data,” Artificial Intelligence Magazine. Vol. 18 (2) ,1997, pp. 37–45.
[48] C. Porcel, A. Tejeda-Lorente, M.A. Martı´ nez and E. Herrera-Viedma, “A hybrid recommender system for the selective dissemination of research resources in a technology transfer office,” Information Sciences. Vol. 184 (1) ,2012, pp. 1–19.
[49] R. Burke, “Hybrid web recommender systems,” in: P. Brusilovsky, A. Kobsa,W. Nejdl (Eds.), The Adaptive Web, Springer-Verlag, Berlin Heidelberg 2007, pp. 377–408.
[50] S. Middleton, D. Roure and N. Shadbolt, “Ontology-based recommender systems,” in: S. Staab, R. Studer (Eds.), Handbook on Ontologies, Springer, Berlin Heidelberg 2009, pp. 779–796.
[51] I. Cantador, A. Bellogín and P. Castells, “A multilayer ontology-based hybrid recommendation model,” AI Communications 21 ,2008 pp. 203–210.
[52] K. Yu, V. Tresp and S. Yu, “A nonparametric hierarchical bayesian framework for information filtering,” Proceedings of the 27th Annual International ACM SIGIR Conference on Research and Development in Information Retrieval, ACM, Sheffield, United Kingdom 2004, pp. 353–360.
[53] C. Christakou, S. Vrettos and A. Stafylopatis, “A hybrid movie recommender system based on neural networks,” International Journal on Artificial Intelligence Tools 16 ,2007 pp. 771–792.
[54] K.-j. Kim and H. Ahn, “A recommender systemusing GA K-means clustering in an online shopping market,” Expert Systems with Applications 34 ,2008, pp. 1200–1209.
[55] C.-N. Ziegler and G. Lausen, “Analyzing correlation between trust and user similarity in online communities,” Trust Management, Springer, 2004 ,pp. 251–265.
[56] G. Adomavicius and A. Tuzhilin, “Context-aware recommender systems,” in: F. Ricci, L. Rokach, B. Shapira, P.B. Kantor (Eds.), Recommender Systems Handbook, Springer, US 2011, pp. 217–253.
[57] M.Claypool, A. Gokhale, T. Miranda, P. Murnikov, D. Netes and M. Sartin, “Combining content- based and collaborative filters in an online newspaper,” In: Proceedings of ACM SIGIR workshop on recommender systems: algorithms and evaluation, Berkeley, California; 1999.
[58] D. Billsus and M.J. Pazzani, “ A hybrid user model for news story classification,” In: Kay J, editor. In: Proceedings of the seventh international conference on user modeling, Banff, Canada. Springer-Verlag, New York; 1999, pp. 99–108.
[59] R. Burke, “Hybrid recommender systems: survey and experiments,” User Model User-adapted Interact, Vol.12(4), 2002, pp.331–70.
[60] B. Smyth and P.A. Cotter, “personalized TV listings service for the digital TV age,” J Knowl-Based Syst Vol. 13(2–3), 2000, PP.53–9.
[61] C. Basu, H. Hirsh and W. Cohen, “Recommendation as classification: using social and content-based information in recommendation,” In: Proceedings of the 15th national conference on artificial intelligence, Madison, WI; 1998. pp. 714–20.
[62] R.J. Mooney and L. Roy, “Content-based book recommending using learning for text categorization,” In: Proceedings of the fifth ACM conference on digital libraries. ACM; 2000, pp. 195–204.
[63] I. Schwab, A. Kobsa and I. Koychev, “Learning user interests through positive examples using content analysis and collaborative filtering,”s Draft from Fraunhofer Institute for Applied Information Technology, Germany; 2001.