Protecting the Users Information in Personalized Recommendation
P.B.Varpe 1 , M.A.Wakchaure 2
Section:Research Paper, Product Type: Journal Paper
Volume-6 ,
Issue-6 , Page no. 285-290, Jun-2018
CrossRef-DOI: https://doi.org/10.26438/ijcse/v6i6.285290
Online published on Jun 30, 2018
Copyright © P.B.Varpe, M.A.Wakchaure . This is an open access article distributed under the Creative Commons Attribution License, which permits unrestricted use, distribution, and reproduction in any medium, provided the original work is properly cited.
View this paper at Google Scholar | DPI Digital Library
How to Cite this Paper
- IEEE Citation
- MLA Citation
- APA Citation
- BibTex Citation
- RIS Citation
IEEE Style Citation: P.B.Varpe, M.A.Wakchaure, “Protecting the Users Information in Personalized Recommendation,” International Journal of Computer Sciences and Engineering, Vol.6, Issue.6, pp.285-290, 2018.
MLA Style Citation: P.B.Varpe, M.A.Wakchaure "Protecting the Users Information in Personalized Recommendation." International Journal of Computer Sciences and Engineering 6.6 (2018): 285-290.
APA Style Citation: P.B.Varpe, M.A.Wakchaure, (2018). Protecting the Users Information in Personalized Recommendation. International Journal of Computer Sciences and Engineering, 6(6), 285-290.
BibTex Style Citation:
@article{_2018,
author = {P.B.Varpe, M.A.Wakchaure},
title = {Protecting the Users Information in Personalized Recommendation},
journal = {International Journal of Computer Sciences and Engineering},
issue_date = {6 2018},
volume = {6},
Issue = {6},
month = {6},
year = {2018},
issn = {2347-2693},
pages = {285-290},
url = {https://www.ijcseonline.org/full_paper_view.php?paper_id=2177},
doi = {https://doi.org/10.26438/ijcse/v6i6.285290}
publisher = {IJCSE, Indore, INDIA},
}
RIS Style Citation:
TY - JOUR
DO = {https://doi.org/10.26438/ijcse/v6i6.285290}
UR - https://www.ijcseonline.org/full_paper_view.php?paper_id=2177
TI - Protecting the Users Information in Personalized Recommendation
T2 - International Journal of Computer Sciences and Engineering
AU - P.B.Varpe, M.A.Wakchaure
PY - 2018
DA - 2018/06/30
PB - IJCSE, Indore, INDIA
SP - 285-290
IS - 6
VL - 6
SN - 2347-2693
ER -
![]() |
![]() |
![]() |
591 | 446 downloads | 312 downloads |
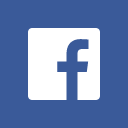
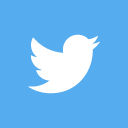
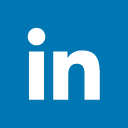
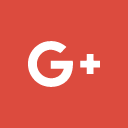
Abstract
As online purchase has growing nowadays, recommendation becomes important field for today. Due to the regard of privacy, user’s unwillingness to expose their private data has become considerable obstacle for the growth of customized recommendation system. So the motive is to safeguard the user’s private data. In this work, it is proposed to formulate the dummy preferences set to protect user’s sensitive subjects. Firstly, a client based structure for user security assurance is introduced, which does not need any modification to existing algorithms, as well as no trade off to the proposal exactness. Then a privacy protection model formulated by the prime requirements such as similarity in the feature distribution and the degree of exposure is put forth. Feature distribution measures the success of dummy preference profile to envelop actual user profile and the degree of exposure measures the favorable result of dummy preferences to envelop sensitive subject. Finally the implementation algorithm is introduced to meet the actual privacy goal. Proposed system also aims to provide the sentiment analysis of the reviews for the products in order to help the people to identify the good products among the huge number of products available.
Key-Words / Index Term
Personalized Recommendation, Individual Privacy, sensitive subjects, Feature Distribution, Dummy Preferences
References
[1] Jieming Zhu, Pinjia He, Zibin Zheng, Michael R. Lyu, “A Privacy-Preserving QoS Prediction Framework for Web Service Recommendation”, 2015 IEEE International Conference on Web Services.
[2] Hwee Hwa PANG, Xuhua DING, Xiaokui XIAO, “Embellishing Text Search Queries to Protect User Privacy”, Proceedings of the VLDB Endowment: 36th International Conference on Very Large Data Bases: Singapore, 13-17 September 2010.
[3] Hwee Hwa PANG, Xiaokui XIAO, Jialie SHEN, “Obfuscating the Topical Intention in Enterprise Text Search”, ICDE 2012: IEEE 28th International Conference on Data Engineering, Arlington Virginia, 1-5 April 2012: Proceedings. 1168-1179.
[4] Feng Zhang, Victor E. Lee, and Ruoming Jin, “k-CoRating: Filling Up Data to Obtain Privacy and Utility”, Proceedings of the Twenty-Eighth AAAI Conference on Artificial Intelligence 2014.
[5] Yilin Shen and Hongxia Jin, “Privacy-Preserving Personalized Recommendation: An Instance-based Approach via Differential Privacy”, 2014 IEEE International Conference on Data Mining.
[6] Zhifeng Luo, Shuhong Chen , Yutian Li, “A Distributed Anonymization Scheme for Privacy-preserving Recommendation Systems”, Supported by University Innovation Research and Training Program of Guangdong Province(1056111033) 2013 IEEE.
[7] Zongda Wu, Guiling Li, et al, ”Covering the sensitive subjects to protect personal privacy in personalized recommendation”, IEEE transaction on serviced computing 2016.
[8] Guandong Xu, ZongdaWu, Guiling Li et al. “Improving contextual advertising matching by using wikipedia thesaurus knowledge”, Knowledge and Information Systems, 2015, 43 (3): 599–631.
[9] Dipasree Pal, Mandar Mitra, Kalyankumar Datta. “Improving query expansion using WordNet”. Journal of the Association for Information Science and Technology, 2014, 65 (12): 2469–2478
[10] S. Zhang, J. Ford and Fillia Makedon, "A privacy-preserving collaborative filtering scheme with two-way communication", Proc. the 7th ACM Conference on Electroinic Commerce, pp. 316-323, 2006.
[11] Liang Hu, Guohang Song, Zhenzhen Xie, and Kuo Zhao, “Personalized Recommendation Algorithm Based on
Preference Features”, Tsinghua science and Technology, Vol. 19, No. 3, 11llpp293-299, June 2014
[12] Yande M, Wakchaure M, Student ME. “Cross-Site Cold-Start Product Recommendation for Social Media and E-Commerce Websites.” International Journal of Engineering Science. 2017 Jul;13751.
[13] Khalid O, Khan M U S, Khan S U et al. ”OmniSuggest: A ubiquitous cloud-based context-aware recommendation system for mobile social networks”. IEEE Transactions on Services Computing, 2014, 7 (3):401414.
[14] Varpe P. “A Preserving Personal Privacy in Personalized Recommendation by protecting the Sensitive Subjects. ”ASIAN JOURNAL FOR CONVERGENCE IN TECHNOLOGY (AJCT)-UGC LISTED. 2018 Apr 15; 4(I).
[15] Shitole MA, Wakchaure MA. “Patient-Centric and Privacy Preserving Clinical Decision Support System Using Naive Bayesian Classification.” 2016, pp. 999-1003
[16] Guandong Xu, ZongdaWu, Guiling Li et al. “Improving contextual advertising matching by using wikipedia thesaurus knowledge”.Knowledge and Information Systems, 2015, 43 (3): 599–631
[17] Wakchaure MM., Survey on Discrimination Prevention in Data-Mining.
[18] Mankar A, Patil H, Arage C, Gaikwad M. “A Survey on Sentiment Computing for the Opinions Based on the Twitter.” International Journal of Scientific Research in computer Science and, Engineering and information Technology, ISSN : 2456-3307, Volume 3 Issue 1, pp.361-364 , 2018
[19] N.Rajganesh, S.Seetha Devi, J. Keerthana, R.Poovizhi, “A Personalized Job Recommender System Using Hybrid Collaborative Filtering Algorithm”, International Journal of Scientific Research in computer Science and, Engineering and information Technology, ISSN : 2456-3307, Volume 3 Issue 3, pp.192-196 , 2018