Implementation of Neuro-Fuzzy and Statistical Technique for Flood Forecasting in Cauvery Basin, India
P. Misra1 , S. Shukla2
Section:Research Paper, Product Type: Journal Paper
Volume-6 ,
Issue-6 , Page no. 291-298, Jun-2018
CrossRef-DOI: https://doi.org/10.26438/ijcse/v6i6.291298
Online published on Jun 30, 2018
Copyright © P. Misra, S. Shukla . This is an open access article distributed under the Creative Commons Attribution License, which permits unrestricted use, distribution, and reproduction in any medium, provided the original work is properly cited.
View this paper at Google Scholar | DPI Digital Library
How to Cite this Paper
- IEEE Citation
- MLA Citation
- APA Citation
- BibTex Citation
- RIS Citation
IEEE Style Citation: P. Misra, S. Shukla, “Implementation of Neuro-Fuzzy and Statistical Technique for Flood Forecasting in Cauvery Basin, India,” International Journal of Computer Sciences and Engineering, Vol.6, Issue.6, pp.291-298, 2018.
MLA Style Citation: P. Misra, S. Shukla "Implementation of Neuro-Fuzzy and Statistical Technique for Flood Forecasting in Cauvery Basin, India." International Journal of Computer Sciences and Engineering 6.6 (2018): 291-298.
APA Style Citation: P. Misra, S. Shukla, (2018). Implementation of Neuro-Fuzzy and Statistical Technique for Flood Forecasting in Cauvery Basin, India. International Journal of Computer Sciences and Engineering, 6(6), 291-298.
BibTex Style Citation:
@article{Misra_2018,
author = { P. Misra, S. Shukla},
title = {Implementation of Neuro-Fuzzy and Statistical Technique for Flood Forecasting in Cauvery Basin, India},
journal = {International Journal of Computer Sciences and Engineering},
issue_date = {6 2018},
volume = {6},
Issue = {6},
month = {6},
year = {2018},
issn = {2347-2693},
pages = {291-298},
url = {https://www.ijcseonline.org/full_paper_view.php?paper_id=2178},
doi = {https://doi.org/10.26438/ijcse/v6i6.291298}
publisher = {IJCSE, Indore, INDIA},
}
RIS Style Citation:
TY - JOUR
DO = {https://doi.org/10.26438/ijcse/v6i6.291298}
UR - https://www.ijcseonline.org/full_paper_view.php?paper_id=2178
TI - Implementation of Neuro-Fuzzy and Statistical Technique for Flood Forecasting in Cauvery Basin, India
T2 - International Journal of Computer Sciences and Engineering
AU - P. Misra, S. Shukla
PY - 2018
DA - 2018/06/30
PB - IJCSE, Indore, INDIA
SP - 291-298
IS - 6
VL - 6
SN - 2347-2693
ER -
![]() |
![]() |
![]() |
541 | 384 downloads | 241 downloads |
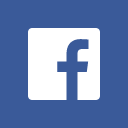
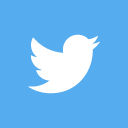
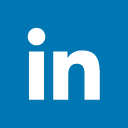
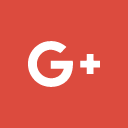
Abstract
Prediction and forecasting has been significant area of study in computer science since last decades. Out of various approaches, soft computing data driven models are very effective for forecasting. Soft Computing Models are usefully applicable when the relationship between the parameters are very complex to understand. India a disaster prone country which requires such major soft computing based data driven models to handle disasters like flood, drought, landslide etc. Flood has a major impact in many parts of India out of which Cauvery, Godavari and Ganges river basins are the mostly affected regions. The paper attempts to forecast floods by modeling river flow in the area of Cauvery river basin of India which has a complicated topography. In this study, the potential of two data driven techniques namely, Adaptive Neuro Fuzzy Inference System (ANFIS) and Gaussian Process Regression (GPR) were used for forecasting floods by predicting river flow in Cauvery river sub-basin of southern India. The techniques were applied on various models constructed from combinations of various antecedent river flow values from two gauging stations and the results were compared for the best fit models of each technique. To get more accurate assessment of results of the models, three standard statistical quantitative performance assessment parameters, the Mean Squared Error (MSE), the coefficient of correlation (R) and the Nash-Sutcliffe coefficient (NS) were used to analyze the performances of the models developed. A complete comparison of the overall performance indices demonstrated that the ANFIS models performed better than GPR models in flood prediction.
Key-Words / Index Term
Adaptive Neuro Fuzzy Inference System (ANFIS), Gaussian Process Regression (GPR), Mean Squared Error (MSE), the coefficient of correlation (R), Nash-Sutcliffe coefficient (NS)
References
[1] M. Aqil, I. Kita, A. Yano, S. Nishiyama, Journal of hydrology 337(1-2), 22, 2007.
[2] Nayak, P. C., K. P. Sudheer, D. M. Rangan, and K. S. Ramasastri. "Short‐term flood forecasting with a Neurofuzzy model." Water Resources Research 41, no. 4, 2005.
[3] Keong, Khoo Chun, Mahfuzah Mustafa, Ahmad Johari Mohammad, Mohd Herwan Sulaiman, and Nor Rul Hasma Abdullah. "Artificial neural network flood prediction for sungai isap residence." In Automatic Control and Intelligent Systems (I2CACIS), IEEE International Conference on, pp. 236-241. IEEE, 2016.
[4] Oh, Hyun-Joo, and Biswajeet Pradhan. "Application of a neuro-fuzzy model to landslide-susceptibility mapping for shallow landslides in a tropical hilly area." Computers & Geosciences 37, no. 9, pp. 1264-1276, 2011.
[5] S.L. Kant, Amal, Pranmohan K. Suman, Brijesh K. Giri, Mukesh K. Tiwari, Chandranath Chatterjee, Purna C. Nayak, and Sawan Kumar. "Comparison of multi-objective evolutionary neural network, adaptive neuro-fuzzy inference system and bootstrap-based neural network for flood forecasting." Neural Computing and Applications 23, no. 1, pp. 231-246, 2013.
[6] Bacanli, Ulker Guner, Mahmut Firat, and Fatih Dikbas. "Adaptive neuro-fuzzy inference system for drought forecasting." Stochastic Environmental Research and Risk Assessment 23, no. 8, pp. 1143-1154, 2009.
[7] Pramanik, Niranjan, and Rabindra Kumar Panda. "Application of neural network and adaptive neuro-fuzzy inference systems for river flow prediction." Hydrological Sciences Journal 54, no. 2, pp. 247-260, 2009.
[8] Firat, Mahmut, and Mahmud Güngör. "Hydrological time‐series modelling using an adaptive neuro‐fuzzy inference system." Hydrological processes 22, no. 13, pp. 2122-2132, 2008.
[9] Shaikh, L, and Sawlani, K. “A Rainfall Prediction Model Using Articial Neural Network” International Journal of Scientific Research in Network Security and Communication Vol. 5, Issue 1, pp. 24 – 28, 2017.
[10] Jaswant Meena, Ashish Mandloi , “Classification of Data Mining Techniques for Weather Prediction”, International Journal of Scientific Research in Computer Science and Engineering, Vol.4, Issue.1, pp.21-24, 2016.
[11] Grbić, Ratko, Dino Kurtagić, and Dražen Slišković. "Stream water temperature prediction based on Gaussian process regression." Expert systems with applications 40, no. 18, pp. 7407-7414, 2013.
[12] Sun, Alexander Y., Dingbao Wang, and Xianli Xu. "Monthly streamflow forecasting using Gaussian process regression." Journal of Hydrology 511, pp. 72-81, 2014.
[13] Pal, Sankar K., and Ashish Ghosh. "Soft computing data mining." pp. 1-3, 2004.
[14] Jang, Jyh-Shing Roger, Chuen-Tsai Sun, and Eiji Mizutani. "Neuro-fuzzy and soft computing; a computational approach to learning and machine intelligence.", 1997.
[15] Rasmussen, Carl Edward, and Christopher KI Williams. "Gaussian processes for machine learning. Number ISBN 0-262-18253-X." 2006.
[16] Bhawan, Sewa, and R. K. Puram. "WATERSHED ATLAS OF INDIA.", 2014.
[17] India-WRIS WebGIS Water Resource Information System of India. Retrieved from http://www.india-wris.nrsc.gov.in/
[18] Kişi, Özgür. "Daily pan evaporation modelling using a neuro-fuzzy computing technique." Journal of hydrology 329, no. 3-4, pp. 636-646, 2006.
[19] Shanker, Murali, Michael Y. Hu, and Ming S. Hung. "Effect of data standardization on neural network training." Omega 24, no. 4, pp. 385-397, 1996.
[20] Shu, C., and T. B. M. J. Ouarda. "Regional flood frequency analysis at ungauged sites using the adaptive neuro-fuzzy inference system." Journal of Hydrology 349, no. 1-2, pp. 31-43, 2008.