An Ingenious Segmentation Application for Brain Lesion Detection in Multimodal MR Images
V. Murugesh1 , V. Sivakumar2 , P. Janarthanan3
Section:Research Paper, Product Type: Journal Paper
Volume-6 ,
Issue-6 , Page no. 367-374, Jun-2018
CrossRef-DOI: https://doi.org/10.26438/ijcse/v6i6.367374
Online published on Jun 30, 2018
Copyright © V. Murugesh, V. Sivakumar, P. Janarthanan . This is an open access article distributed under the Creative Commons Attribution License, which permits unrestricted use, distribution, and reproduction in any medium, provided the original work is properly cited.
View this paper at Google Scholar | DPI Digital Library
How to Cite this Paper
- IEEE Citation
- MLA Citation
- APA Citation
- BibTex Citation
- RIS Citation
IEEE Style Citation: V. Murugesh, V. Sivakumar, P. Janarthanan, “An Ingenious Segmentation Application for Brain Lesion Detection in Multimodal MR Images,” International Journal of Computer Sciences and Engineering, Vol.6, Issue.6, pp.367-374, 2018.
MLA Style Citation: V. Murugesh, V. Sivakumar, P. Janarthanan "An Ingenious Segmentation Application for Brain Lesion Detection in Multimodal MR Images." International Journal of Computer Sciences and Engineering 6.6 (2018): 367-374.
APA Style Citation: V. Murugesh, V. Sivakumar, P. Janarthanan, (2018). An Ingenious Segmentation Application for Brain Lesion Detection in Multimodal MR Images. International Journal of Computer Sciences and Engineering, 6(6), 367-374.
BibTex Style Citation:
@article{Murugesh_2018,
author = {V. Murugesh, V. Sivakumar, P. Janarthanan},
title = {An Ingenious Segmentation Application for Brain Lesion Detection in Multimodal MR Images},
journal = {International Journal of Computer Sciences and Engineering},
issue_date = {6 2018},
volume = {6},
Issue = {6},
month = {6},
year = {2018},
issn = {2347-2693},
pages = {367-374},
url = {https://www.ijcseonline.org/full_paper_view.php?paper_id=2189},
doi = {https://doi.org/10.26438/ijcse/v6i6.367374}
publisher = {IJCSE, Indore, INDIA},
}
RIS Style Citation:
TY - JOUR
DO = {https://doi.org/10.26438/ijcse/v6i6.367374}
UR - https://www.ijcseonline.org/full_paper_view.php?paper_id=2189
TI - An Ingenious Segmentation Application for Brain Lesion Detection in Multimodal MR Images
T2 - International Journal of Computer Sciences and Engineering
AU - V. Murugesh, V. Sivakumar, P. Janarthanan
PY - 2018
DA - 2018/06/30
PB - IJCSE, Indore, INDIA
SP - 367-374
IS - 6
VL - 6
SN - 2347-2693
ER -
![]() |
![]() |
![]() |
651 | 381 downloads | 244 downloads |
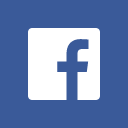
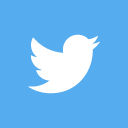
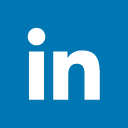
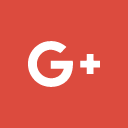
Abstract
Automatic segmentation and detection of brain disease is a disreputably complicated issue in Magnetic Resonance Image (MRI). The similar state-of-art segmentation methods and techniques are limited for the detection of brain disease in multimodal brain MRI. Thus this research work deals with the accurate segmentation and detection of brain diseases in multimodal brain MRI and this research work is focused on improve automatic segmentation results. This work analyses the segmentation performance of existing state-of-art method improved Fuzzy C-Means Clustering (FCMC) method and marker controlled Watershed method .In our research work the proposed method is to compound segmentation results of improved Fuzzy C-Means Clustering (FCMC) method and marker controlled Watershed method to carry out accurate brain tumor detection and improved the segmentation results. The performance of proposed method is evaluated with the assorted performance metric, viz., Segmentation accuracy, Sensitivity and Specificity. The comparative performance of our Proposed Method, FCMC Method and Watershed method is demonstrated on real and benchmark multimodal brain MRI datasets, viz. FLAIR (Fluid Attenuated Inversion Recovery) MRI, T1 MRI, MRI and T2 MRI and the experimental results of the proposed method exhibits better results for segmentation and detection of brain diseases in multimodal brain MR images.
Key-Words / Index Term
Brain diseases, FCMC Method, Watershed Method, Proposed Method, Bilateral Filter, Brain MRI, Multimodal
References
[1] N Van . Porz, "Multi-modalodal glioblastoma segmentation: Man versus machine", PLOS ONE, Vol. 9, pp. e96873, 2014.
[2]J.L. Marroquin, B.C. Vemuri, S. Botello and F. Calderon, ―An accurate and efficient Bayesian method for automatic segmentation of brain MRI,‖ Proceedings of the 7th European Conference on Computer Vision, London, UK, August 2002.
[3] M.G DiBono and M. Zorzi, ―Decoding cognitive states from fMRI data using support vector regression,‖ Psychology Journal, 2008.
[4] S. Bauer, R. Wiest, L.-P. Nolte and M. Reyes, "A survey of MRI-based medical image analysis for brain tumor studies", Phys. Med. Biol., Vol. 58, No. 13, Pp. R97-R129, 2013.
[5] Z. Shi, L. He, T.N.K Suzuki, and H. Itoh, ―Survey on Neural Networks used for Medical Image Processing,‖ International Journal of Computational Science, 2009.
[6] V.B Padole and D.S. Chaudhari, ―Detection of Brain Tumor in MRI Images Using Mean Shift Algorithm and Normalized Cut Method,‖ International Journal of Engineering and Advanced Technology, June 2012.
[7] L. Weizman, "Automatic segmentation, internal classification, and follow-up of optic pathway gliomas in MRI", Med. Image Anal., vol. 16, no. 1, pp. 177-188, 2012.
[8].Meenakshi, R & Anandhakumar, P 2012, ‘Brain Tumor identification in MRI with BPN Classifier and Orthonormal Operators’, European Journal of Scientific Research, vol.85, no.4, pp.559-569.
[9] S. Ahmed, K. M. Iftekharuddin and A. Vossough, "Efficacy of texture, shape, and intensity feature fusion for posterior-fossa tumor segmentation in MRI", IEEE Trans. Inf. Technol. Biomed., Vol. 15, No. 2, Pp. 206-213, 2011.
[10] Jin Liu, Min Li, Jianxin Wang, Fangxiang Wu, Tianming Liu, and Yi Pan, “A Survey of MRI-Based Brain Tumor Segmentation Methods”, TSINGHUA SCIENCE AND TECHNOLOGY,Volume 19, Number 6, December 2014.
[11] J. B. T. M. Roerdink and A. Meijster, “The watershed transform: Definitions, Algorithms and parallelization strategies,” Fundamenta Informaticae, Vol. 41, Pp. 187–228, 2000.
[12] Gang Li , “Improved watershed segmentation with optimal scale based on ordered dither halftone and mutual information”,Page(s)296-300, Computer Science and Information Technology (ICCSIT), 2010, 3rd IEEE International Conference, 9-11 July 2011.
[13] Benson. C. C, Deepa V, Lajish V. L and Kumar Rajamani, "Brain Tumor Segmentation from MR Brain Images using Improved Fuzzy c-Means Clustering and Watershed Algorithm", Intl. Conference on Advances in Computing, Communications and Informatics (ICACCI), Sept. 21-24, 2016, Jaipur, India.
[14] L´aszl´o Szil´agyi,L´aszl´o Lefkovits and Bal´azs Beny´o, "Automatic Brain Tumor
Segmentation in Multispectral MRI Volumes Using a Fuzzy c-Means Cascade Algorithm",12th International Conference on Fuzzy Systems and Knowledge Discovery (FSKD),2015.
[15] G.-C. Lin, W.-J. Wang, C.-C. Kang and C.-M. Wang, “Multispectral MR images segmentation based on fuzzy knowledge and modified seeded region growing”, Magnetic Resonance Imaging(MRI), Vol. 30, No. 2, Pp. 230-246, 2012.
[16]NageswaraReddy P, C.P.V.N.J.Mohan Rao, Ch.Satyanarayana, “Optimal Segmentation Framework for Detection of Brain Anomalies”, I.J. Engineering and Manufacturing, 2016, 6, 26-37, November 2016 in MECS.
[17] Devanand Bhonsle, Vivek Chandra , G.R. Sinha3 , “Medical Image Denoising Using Bilateral Filter”, I.J. Image, Graphics and Signal Processing, 2012, 6, 36-43. July 2012 in MECS.
[18] Sanjay Kumar, Santosh Kumar Ray, Peeyush Tewari,, A “Hybrid Approach for Image Segmentation Using Fuzzy Clustering and Level Set Method”, I.J. Image, Graphics and Signal Processing, 2012, 6, 1-7, July 2012 in MECS.
[19] Kalaiselvi T and Nagaraja P, “An Automatic Segmentation of Brain Tumor from MRI Scans through Wavelet Transformations”, I.J. Image, Graphics and Signal Processing, 2016, 11, 59- 65, November 2016 in MECS.
[20] Anam Mustaqeem, Ali Javed, Tehseen Fatima, “An Efficient Brain Tumor Detection Algorithm Using Watershed & Thresholding Based Segmentation”, I.J. Image, Graphics and Signal Processing, 2012, 10, 34-39, September 2012 in MECS.
[21] B.Jyothia, Y.MadhaveeLathab, P.G.Krishna Mohanc ,V.S.K.Reddy, ”Integrated Multiple Features for Tumor Image Retrieval Using Classifier and Feedback Methods”, International Conference on Computational Modeling and Security (CMS 2016), Science direct, 2016, PP141-148.
[22] L.A. Khoo, P. Taylor, and R.M. Given-Wilson, “Computer- Aided Detection in the United Kingdom National Breast Screening Programme: Prospective Study,” Radiology, vol. 237, pp. 444-449, 2005.
[23] Pritam Dungarwal and Prof. Dinesh Patil, “Literature Survey on Detection of lumps in brain” International Journal of Modern Trends in Engineering and Research (IJMTER) Volume 03, Issue 12, December – 2016,pp 312-316.
[24] Krit Somkantha, Nipon Theera-Umpon, “Boundary Detection in Medical Images Using Edge Following Algorithm Based on Intensity Gradient and Texture Gradient Features,” in Proc.IEEE transactions on biomedical engineering, Vol. 58, No. 3, March 2011,Pp. 567–573.
[25] B.Jyothi, Y.MadhaveeLatha, P.G.Krishna Mohan,” Multidimentional Feature Vector Space for an Effective Content Based Medical Image Retrieval”, 5th IEEE International Advance Computing Conference(IACC- 2015),BMS College of Engineering Bangalore, June 12 to 13
,2015.
[26] Nitin Jain & Dr. S. S. Salankar,” Color & Texture Feature Extraction for Content Based Image Retrieval”, IOSR Journal of Electrical and Electronics Engineering (IOSR-JEEE) e-ISSN: 2278-1676, p-ISSN: 2320-3331 PP 53-58.
[27] J. Selvakumar, A. Lakshmi, T. Arivoli, “Brain tumor segmentation and its Area calculation in Brain MR images using K-Mean clustering and Fuzzy C-Mean Algorithm”, IEEE- International conference on advances in engineering science and management, March-2012.
[28] Cs Pillai, “A Survey of Shape Descriptors for Digital Image Processing”, IRACST – International Journal of Computer Science and Information Technology & Security (IJCSITS), ISSN: 2249-9555 Vol. 3, No.1, February 2013.
[29] Prashant Aher and Umesh Lilhore, “An improved CBMIR architecture, based on modified classifiers & feedback method for tumor image retrieval from MRI images” International Journal of Modern Trends in Engineering and Research (IJMTER) Volume 03, Issue 12, Dec – 2016,pp-157- 160.