Study on Migration from conventional File System to advancement of Bigdata Technologies in the real world
P.N. Priyanka1 , S.V. Phaneendra2
Section:Survey Paper, Product Type: Journal Paper
Volume-2 ,
Issue-8 , Page no. 11-20, Aug-2014
Online published on Aug 31, 2014
Copyright © P.N. Priyanka, S.V. Phaneendra . This is an open access article distributed under the Creative Commons Attribution License, which permits unrestricted use, distribution, and reproduction in any medium, provided the original work is properly cited.
View this paper at Google Scholar | DPI Digital Library
How to Cite this Paper
- IEEE Citation
- MLA Citation
- APA Citation
- BibTex Citation
- RIS Citation
IEEE Style Citation: P.N. Priyanka, S.V. Phaneendra, “Study on Migration from conventional File System to advancement of Bigdata Technologies in the real world,” International Journal of Computer Sciences and Engineering, Vol.2, Issue.8, pp.11-20, 2014.
MLA Style Citation: P.N. Priyanka, S.V. Phaneendra "Study on Migration from conventional File System to advancement of Bigdata Technologies in the real world." International Journal of Computer Sciences and Engineering 2.8 (2014): 11-20.
APA Style Citation: P.N. Priyanka, S.V. Phaneendra, (2014). Study on Migration from conventional File System to advancement of Bigdata Technologies in the real world. International Journal of Computer Sciences and Engineering, 2(8), 11-20.
BibTex Style Citation:
@article{Priyanka_2014,
author = {P.N. Priyanka, S.V. Phaneendra},
title = {Study on Migration from conventional File System to advancement of Bigdata Technologies in the real world},
journal = {International Journal of Computer Sciences and Engineering},
issue_date = {8 2014},
volume = {2},
Issue = {8},
month = {8},
year = {2014},
issn = {2347-2693},
pages = {11-20},
url = {https://www.ijcseonline.org/full_paper_view.php?paper_id=219},
publisher = {IJCSE, Indore, INDIA},
}
RIS Style Citation:
TY - JOUR
UR - https://www.ijcseonline.org/full_paper_view.php?paper_id=219
TI - Study on Migration from conventional File System to advancement of Bigdata Technologies in the real world
T2 - International Journal of Computer Sciences and Engineering
AU - P.N. Priyanka, S.V. Phaneendra
PY - 2014
DA - 2014/08/31
PB - IJCSE, Indore, INDIA
SP - 11-20
IS - 8
VL - 2
SN - 2347-2693
ER -
![]() |
![]() |
![]() |
3686 | 3680 downloads | 3671 downloads |
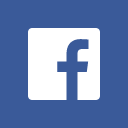
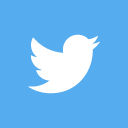
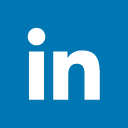
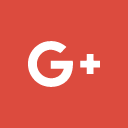
Abstract
Now a day the internet was generating an explosion in growth of data in the form of data sets called Big Data that are complex to store, manage and analyze using conventional RDBMS that is used for Online Transaction Processing (OLTP) only. This new data is not only unstructured, voluminous but even more difficult to control, the cost of hardware and software infrastructure required to crunch it using conventional RDBMS. To exploit on the Big Data trend, a new type of Bigdata technologies (like Hadoop, HBase, PIG, HIVE, SQOOP,OOZIE, APACHE FLUME, MAHOUT) have developed by many companies that leverages new paralleled processing, commodity machines, open source framework to capture and analyze these new data sets. Bigdata presents a performance better than the existing Database or Data Warehouse or Business Intelligence systems. In this study we shall know how the emergent Bigdata is controlled for managing huge volume of data. This paper also lays out the ecosystem of big data technology that has been evolving rapidly.
Key-Words / Index Term
Bigdata, Flume, DBMS, HBase, HIVE, Mahout, OOZIE, PIG, RDBMS, SQOOP
References
[1]. Brad Brown, Michael Chui, and James Manyika, Are you ready for the era of �big data�?, McKinseyQuaterly, Mckinsey Global Institute, October 2011.
[2]. Bhandarkar M: MapReduce programming with apache Hadoop in the proceedings of IEEE 24th International Symposium on Parallel & Distributed Processing (IPDPS� 10) 2010.
[3]. Cisco Internet Business Solutions Group (IBSG): The Internet of Things: How the Next Evolution of the Internet is Changing Everything 2011.
[4]. Digital Universe Study (on behalf of EMC Corporation): Big Data, Bigger Digital Shadows, and Biggest Growth in the Far East 2012.
[5]. DunrenChe, MejdlSafran, and ZhiyongPeng, From Big Data to Big Data Mining: Challenges, Issues, and Opportunities, DASFAA Workshops 2013, LNCS 7827, pp. 1�15, 2013.
[6]. Dean J, Ghemawat S: �MapReduce: Simplified Data Processing on Large Clusters� San Francisco, CA: OSDI` 04 Proceedings of the 6th symposium on Operating System Design and Implementation; 2004.
[7]. Ekpe Okorafor1 and Mensah Kwabena Patrick : Availability of jobtracker machine in Hadoop/MapReduce Zookeeper coordinated clusters in Advanced Computing: An International Journal ( ACIJ ), Vol.3, No.3, May 2012
[8]. Esteves RM, Rong C: Using Mahout for Clustering Wikipedia�s Latest Articles: A Comparison between K-means and Fuzzy C-means in the Cloud in the proceedings of IEEE Third International Conference on Cloud Computing Technology and Science (Cloud Com �11) 2011 IEEE pp 565�569.
[9]. Esteves RM, Pais R, Rong C: K-means Clustering in the Cloud � A Mahout Test. IEEE International Conference on Advanced Information Networking and Applications (WAINA �11) 2011.
[10]. GrzegorzMalewicz, Matthew H. Austern, Aart J. C. Bik, James C.Dehnert, Ilan Horn, NatyLeiser, and GrzegorzCzajkowski,Pregel: A System for Large-Scale Graph Processing, SIGMOD‟10, June 6�11, 2010, pp 135-145.
[11]. Garhan Attebury. "Hadoop distributed file system for the Grid", 2009 IEEE Nuclear Science Symposium Conference Record (NSS/MIC), 10/2009
[12]. J. Dean and S. Ghemawat, �MapReduce: Simplified data processing on large clusters,� in USENIX Symposium on Operating Systems Design and Implementation, San Francisco, CA, Dec. 2004, pp. 137�150.
[13]. Jefry Dean and Sanjay Ghemwat, MapReduce: A Flexible Data Processing Tool, Communications of the ACM, Volume 53, Issuse.1,January 2010, pp 72-77.
[14]. Karmasphere: Deriving Intelligence from Big Data in Hadoop: A Big Data Analytics Primer 2011.
[15]. Kyuseok Shim, MapReduce Algorithms for Big Data Analysis, DNIS 2013, LNCS 7813, pp. 44�48, 2013.
[16]. McKinsey Global Institute: Big data: The next frontier for innovation, competition, and productivity 2011.
[17]. OnurSavas, YalinSagduyu, Julia Deng, and Jason Li,Tactical Big Data Analytics: Challenges, Use Cases and Solutions, Big Data Analytics Workshop in conjunction with ACM Sigmetrics 2013,June 21, 2013.
[18]. Owen S, Anil R, Dunning T, Friedman E, Manning Publications: Mahout in action. Chapter 8. Shelter Island, N.Y; 2012:130-144.
[19]. Poornima Sharma, Varun Garg , Prof. Randeep Kaur , Prof. Satendra Sonare , "Big Data in Cloud Environment", Int. Journal of Computer Sciences and Engineering, Volume01, Issue-03, Page No (15-17), Nov 2013.
[20]. Ren, Shengbing, and Dieudonne Muheto. "A Reactive Scheduling Strategy Applied On MapReduce OLAM Operators System", Journal of Software, 2012.
[21]. Sanjay Ghemawat and Jeffrey Dean, �MapReduce: Simplified data processing on large clusters,� in OSDI`04: Proceedings of the 6th Symposium on Operating Systems Design and Implementation. USENIX Association, 2004.
[22]. S. Ghemawat, H. Gobioff, and S. Leung, �The Google File System.� in ACM Symposium on Operating Systems Principles, Lake George, NY, Oct 2003, pp. 29 � 43.
[23]. Shvachko K, Kuang H, Radia S, Chansler R: The Hadoop Distributed File System in the proceedings of IEEE 26th Symposium on Mass Storage Systems and Technologies (MSST� 10) 2010.
[24]. Shen, Qingni, Yahui Yang, Zhonghai Wu, Dandan Wang, and Min Long. "Securing dataservices: a security architecture design for private storage cloud based on HDFS", International Journal of Grid and Utility Computing, 2013.
[25]. S. Vikram Phaneendra, E. Madhusudhana Reddy,� Big Data - Solutions for RDBMS Problems - A Survey�, International Journal of Advanced Research in Computer and Communication Engineering, ISSN (Print): 2319-5940, ISSN (Online) : 2278-1021, Volume 2, Issue 9, September 2013, pp 3686-3691.
[26]. Tyson Condie, Neil Conway, Peter Alvaro, Joseph M. Hellerstein, JohnGerth, Justin Talbot, Khaled Elmeleegy, Russell Sears, Online Aggregation and Continuous Query support in MapReduce, SIGMOD 10, June 6�11, 2010, Indianapolis, Indiana, USA.
[27]. VinayakBorkar, Michael J. Carey, Chen Li, Inside �Big Data Management�:Ogres, Onions, or Parfaits?, EDBT/ICDT 2012 Joint Conference Berlin, Germany,2012 ACM 2012, pp 3-14.
[28]. Venner. "The Basics of a MapReduce Job", Pro Hadoop, 2009
[29]. Wang, Lizhe, Jie Tao, Rajiv Ranjan, Holger Marten, Achim Streit, Jingying Chen, and DanChen. "G-Hadoop:MapReduce across distributed data centers for data-intensive computing", Future Generation Computer Systems, 2013.
[30]. Apache: Apache Hadoop, http://hadoop.apache.org
[31]. Apache Hbase 2012, http://hbase.apache.org
[32]. Apache PIG, http://pig.apache.org/
[33]. Apache Hive, 2012 http://hive.apache.org/
[34]. HADOOP-3759: Provide ability to run memory intensive jobs without affecting other running tasks on the nodes. https://issues.apache.org/jira/browse/HADOOP-3759
[35]. Karmasphere: Understanding the Elements of Big Data: More than Hadoop Distribution 2011.
[36]. MarcinJedyk, MAKING BIG DATA, SMALL, Using distributed systems for processing, analysing and managing large huge data sets, Software Professionals Network, Cheshire Data systems Ltd.
[37]. Mahout, http://lucene.apache.org/mahout/
[38]. T. White, Hadoop: The Definitive Guide. O�Reilly, 2009. pp. 33-36, 68.
[39]. The Age of Big Data. Steve Lohr. New York Times, Feb 11, 2012. http://www.nytimes.com/2012/02/12/sunday-review/bigdatas-impact-in-the-world.html
[40]. http://www.wikidifference.com