Cancer Classification from Gene Expression data using Fuzzy-Rough techniques: An Empirical Study
Ansuman Kumar1 , Anindya Halder2
Section:Research Paper, Product Type: Journal Paper
Volume-6 ,
Issue-6 , Page no. 415-420, Jun-2018
CrossRef-DOI: https://doi.org/10.26438/ijcse/v6i6.415420
Online published on Jun 30, 2018
Copyright © Ansuman Kumar, Anindya Halder . This is an open access article distributed under the Creative Commons Attribution License, which permits unrestricted use, distribution, and reproduction in any medium, provided the original work is properly cited.
View this paper at Google Scholar | DPI Digital Library
How to Cite this Paper
- IEEE Citation
- MLA Citation
- APA Citation
- BibTex Citation
- RIS Citation
IEEE Style Citation: Ansuman Kumar, Anindya Halder, “Cancer Classification from Gene Expression data using Fuzzy-Rough techniques: An Empirical Study,” International Journal of Computer Sciences and Engineering, Vol.6, Issue.6, pp.415-420, 2018.
MLA Style Citation: Ansuman Kumar, Anindya Halder "Cancer Classification from Gene Expression data using Fuzzy-Rough techniques: An Empirical Study." International Journal of Computer Sciences and Engineering 6.6 (2018): 415-420.
APA Style Citation: Ansuman Kumar, Anindya Halder, (2018). Cancer Classification from Gene Expression data using Fuzzy-Rough techniques: An Empirical Study. International Journal of Computer Sciences and Engineering, 6(6), 415-420.
BibTex Style Citation:
@article{Kumar_2018,
author = {Ansuman Kumar, Anindya Halder},
title = {Cancer Classification from Gene Expression data using Fuzzy-Rough techniques: An Empirical Study},
journal = {International Journal of Computer Sciences and Engineering},
issue_date = {6 2018},
volume = {6},
Issue = {6},
month = {6},
year = {2018},
issn = {2347-2693},
pages = {415-420},
url = {https://www.ijcseonline.org/full_paper_view.php?paper_id=2197},
doi = {https://doi.org/10.26438/ijcse/v6i6.415420}
publisher = {IJCSE, Indore, INDIA},
}
RIS Style Citation:
TY - JOUR
DO = {https://doi.org/10.26438/ijcse/v6i6.415420}
UR - https://www.ijcseonline.org/full_paper_view.php?paper_id=2197
TI - Cancer Classification from Gene Expression data using Fuzzy-Rough techniques: An Empirical Study
T2 - International Journal of Computer Sciences and Engineering
AU - Ansuman Kumar, Anindya Halder
PY - 2018
DA - 2018/06/30
PB - IJCSE, Indore, INDIA
SP - 415-420
IS - 6
VL - 6
SN - 2347-2693
ER -
![]() |
![]() |
![]() |
449 | 369 downloads | 133 downloads |
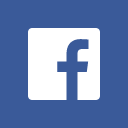
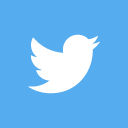
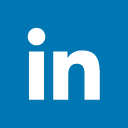
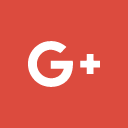
Abstract
Cancer classification from gene expression data is one of the most challenging research areas in the field of computation biology, bioinformatics and machine learning as the number of clinically labeled samples are very few compared to number of genes present. Also the cancer subtype classes are often highly overlapping, imprecise, and indiscernible in nature. Various machine techniques have been developed and applied on gene expression data for cancer sample classification. Here in this article, an empirical study of cancer classification from microarray gene expression data is performed using fuzzy-rough nearest neighbour techniques where performance of four different types of classifiers viz., Fuzzy nearest neighbour, Fuzzy-rough nearest neighbour, Vaguely quantified fuzzy-rough nearest neighbour and Ordered weighted average based fuzzy-rough nearest neighbor are investigated. The experiments are carried out on eight publicly available real life microarray gene expression cancer datasets. To assess the results of the classifiers percentage accuracy, precision, recall, macro averaged F1 measure, micro averaged F1 measure and kappa are used. The comparative study of the investigated methods is also done using paired t-test. Fuzzy-rough nearest neighbour method is found to be better for most of the data sets for cancer classification.
Key-Words / Index Term
Cancer Classification, Fuzzy-Rough set, Vaguely Quantified, Ordered Weighted Average, Microarray Gene Expression data
References
[1] D. Stekel, “Microarray Bioinformatics”, 1st ed., Cambridge, Cambridge University Press, UK, 2003.
[2] M. Dettling and P. Buhlmann, “Boosting for tumor classification with gene expression data”, Bioinformatics, Vol. 19, Issue. 9, pp.1061–1069, 2003.
[3] D. Jiang, C. Tang and A. Zhang, “Cluster analysis for gene expression data: A survey”, IEEE Transactions on Knowledge and Data Engineering, Vol.16, Issue.11,pp.1370–1386, 2004.
[4] R. Priscilla and S. Swamynathan, “A semi-supervised hierarchical approach: two-dimensional clustering of microarray gene expression data”, Frontiers of Computer Science, Vol.7, Issue.2, pp. 204–213, 2013.
[5] A. Halder and S. Misra, “Semi-supervised fuzzy k-NN for cancer classification from microarray gene expression data”, in Proceedings of the 1st International Conference on Automation, Control, Energy and Systems (ACES 2014) (IEEE Computer Society Press) pp.1–5,2014.
[6] D. Du, K. Li, X. Li, and M. Fei, “A novel forward gene selection algorithm for microarray data,” Neurocomputing, vol. 133, pp. 446–458, 2014.
[7] L. Zadeh, “Fuzzy sets”, Information and Control, Vol.8, Issue.3, pp.338–353, 1965.
[8] Z. Pawlak, “Rough sets”, International Journal of Computer and Information Science, Vol.11, Issue.5, pp.341–356, 1982.
[9] A.M. Radzikowska and E.E. Kerre, “A comparative study of fuzzy rough sets”, Fuzzy Sets and Systems, Vol.126, pp.137–156, 2002.
[10] J.M. Keller, M.R. Gray and J.A. Givens, “A fuzzy K -nearest neighbor algorithm”, IEEE Transactions on Systems, Man and Cybernetics, Vol.15, Issue.4, pp. 580–585, 1985.
[11] R. Jensen and C. Cornelis, “A new approach to fuzzy-rough nearest neighbour classification”, in: Proceedings of the 6th International Conference on Rough Sets and Current Trends in Computing, pp. 310–319, 2008.
[12] R. R. Yager, “On ordered weighted averaging aggregation operators in multicriteria decision making”, IEEE Transaction on Systems, Man and Cybernetics, Vol.18, pp.183-190, 1988.
[13] M. Dettling, “Bagboosting for tumor classification with gene expression data”, Bioinformatics, Vol.20, Issue.18, pp.583–593, 2004.
[14] Technology Agency for Science and Research. Kent ridge bio-medical dataset repository.
[15] A. Halder, S. Ghosh, and A. Ghosh. “Aggregation pheromone metaphor for semi-supervised classification”, Pattern Recognition, Vol.46, Issue.8, pp.2239–2248, 2013.
[16] J. Cohen, “A coefficient of agreement for nominal scales”, Educational and Psychological Measurement, Vol.20, Issue.1 pp. 37–46, 1960.
[17] E. Kreyszig, “Introductory Mathematical Statistics”, 1st ed,
John Wily, 1970.