Fitting the Best Model for ACEs Using Interrupted Time Series Data
A. Srinivasulu1 , B. Sarojamma2
Section:Research Paper, Product Type: Journal Paper
Volume-6 ,
Issue-6 , Page no. 753-760, Jun-2018
CrossRef-DOI: https://doi.org/10.26438/ijcse/v6i6.753760
Online published on Jun 30, 2018
Copyright © A. Srinivasulu, B. Sarojamma . This is an open access article distributed under the Creative Commons Attribution License, which permits unrestricted use, distribution, and reproduction in any medium, provided the original work is properly cited.
View this paper at Google Scholar | DPI Digital Library
How to Cite this Paper
- IEEE Citation
- MLA Citation
- APA Citation
- BibTex Citation
- RIS Citation
IEEE Style Citation: A. Srinivasulu, B. Sarojamma, “Fitting the Best Model for ACEs Using Interrupted Time Series Data,” International Journal of Computer Sciences and Engineering, Vol.6, Issue.6, pp.753-760, 2018.
MLA Style Citation: A. Srinivasulu, B. Sarojamma "Fitting the Best Model for ACEs Using Interrupted Time Series Data." International Journal of Computer Sciences and Engineering 6.6 (2018): 753-760.
APA Style Citation: A. Srinivasulu, B. Sarojamma, (2018). Fitting the Best Model for ACEs Using Interrupted Time Series Data. International Journal of Computer Sciences and Engineering, 6(6), 753-760.
BibTex Style Citation:
@article{Srinivasulu_2018,
author = {A. Srinivasulu, B. Sarojamma},
title = {Fitting the Best Model for ACEs Using Interrupted Time Series Data},
journal = {International Journal of Computer Sciences and Engineering},
issue_date = {6 2018},
volume = {6},
Issue = {6},
month = {6},
year = {2018},
issn = {2347-2693},
pages = {753-760},
url = {https://www.ijcseonline.org/full_paper_view.php?paper_id=2250},
doi = {https://doi.org/10.26438/ijcse/v6i6.753760}
publisher = {IJCSE, Indore, INDIA},
}
RIS Style Citation:
TY - JOUR
DO = {https://doi.org/10.26438/ijcse/v6i6.753760}
UR - https://www.ijcseonline.org/full_paper_view.php?paper_id=2250
TI - Fitting the Best Model for ACEs Using Interrupted Time Series Data
T2 - International Journal of Computer Sciences and Engineering
AU - A. Srinivasulu, B. Sarojamma
PY - 2018
DA - 2018/06/30
PB - IJCSE, Indore, INDIA
SP - 753-760
IS - 6
VL - 6
SN - 2347-2693
ER -
![]() |
![]() |
![]() |
438 | 288 downloads | 132 downloads |
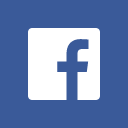
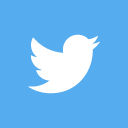
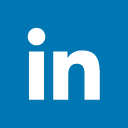
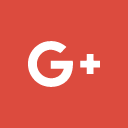
Abstract
The interrupted time series data plays a very important role in the evaluation of public health interventions and also to improve hospital antibiotic prescribing. We take the data from a study on the effects of the Italian smoking ban in public places on hospital admissions for Acute Coronary Events (ACEs). In January 2005, Italy introduced regulations to ban smoking in all indoor public places, which the aim of limiting adverse health affects of second hand smoke. The data used here are ACEs in the Sicily region between 2002 and 2006 among those aged 0-69 years. In this paper, we use three models for interrupted time series data. Root Mean Square Error (RMSE) measure is used for selecting the best model. Three models are empirically tested using interrupted time series smoke ban data in the Sicily region, Italy.
Key-Words / Index Term
ARIMA models, Before Intervention, After Intervention, Adaptive smoothing model, R2, RMSE.
References
[1] James Lopez Bernal, Steven Cummins, Antonio Gasparrini, “Interrupted Time Series Regression for The Evaluation of Public Health Interventions: a tutorial”, International Journal of Epidemiology, 348-355, 2017.
[2] Ariel Linden, “Conducting Interrupted Time Series Analysis for Single and Multiple Group Comparisions”, The Stata Journal 15, (2), 480-500, 2015.
[3] Evangelos Kontopantelis, Tim Doran, David A Springate, Lain Buchan, David Reeves,“Regression Based Quasi-Experimental Approach When Randomisation is not an option: Interrupted Time Series Analysis”, The BMJ; 350:h2750, 2015.
[4] Monica Taljaard, Joanne E McKenzie, Craig R Ramsay, Jeremy M Grimshaw, “ The Use of Segmented Regression in Analysing Interrupted Time Series Studies: An Exmple in Pre-Hospital Ambulance care”, Implementation Science, 9:77, 2014.
[5] Faranak Ansari, Kiesteen Gray, Dilip Nathwani, Gabby Philips, Simon Ogston, Craig Ramsay, Peter Davely “Outcomes of an Intervention to Improve Hospital Antibiotic Prescribing: Interrupted Time Series with Segmented Regression Analysis”, Journal of Antimicrobial Chemotherapy, 52, 842-848, 2003.
[6] A.K. Wagner, S,B. Soumerai, F.Zhang, D.Ross-Degnan “Segmented Regression Analysis of Interrupted Time Series Studies in Medication Use Research” Journal of Clinical Pharmacy and Therapeutics, 27, 299-309, 2002.
[7] Gene V Glass,”Interrupted Time Series Quasi-Experiments”, American Educational Research Association., 2nd edition 589-608, 1997.
[8] Donald P.Hartmann, John M. Gottman, Richard R. Jones, William Gardner, Alan E. Kazdin, Russell S. Vaught “Interrupted Time Series Analysis and Its Application to Behavioral Data”,Journal of Applied Behavior Analysis,13, 543-559, 1980.
[9] http://ije.oxfordjournals.org/lookup/suppl/doi:10.1093/ije/dyw098/-/DC1.