Divergence Based Generalized Fuzzy Rough Sets
Sheeja T.K.1 , Sunny Kuriakose A.2
Section:Research Paper, Product Type: Journal Paper
Volume-6 ,
Issue-6 , Page no. 809-815, Jun-2018
CrossRef-DOI: https://doi.org/10.26438/ijcse/v6i6.809815
Online published on Jun 30, 2018
Copyright © Sheeja T.K., Sunny Kuriakose A. . This is an open access article distributed under the Creative Commons Attribution License, which permits unrestricted use, distribution, and reproduction in any medium, provided the original work is properly cited.
View this paper at Google Scholar | DPI Digital Library
How to Cite this Paper
- IEEE Citation
- MLA Citation
- APA Citation
- BibTex Citation
- RIS Citation
IEEE Style Citation: Sheeja T.K., Sunny Kuriakose A., “Divergence Based Generalized Fuzzy Rough Sets,” International Journal of Computer Sciences and Engineering, Vol.6, Issue.6, pp.809-815, 2018.
MLA Style Citation: Sheeja T.K., Sunny Kuriakose A. "Divergence Based Generalized Fuzzy Rough Sets." International Journal of Computer Sciences and Engineering 6.6 (2018): 809-815.
APA Style Citation: Sheeja T.K., Sunny Kuriakose A., (2018). Divergence Based Generalized Fuzzy Rough Sets. International Journal of Computer Sciences and Engineering, 6(6), 809-815.
BibTex Style Citation:
@article{T.K._2018,
author = {Sheeja T.K., Sunny Kuriakose A.},
title = {Divergence Based Generalized Fuzzy Rough Sets},
journal = {International Journal of Computer Sciences and Engineering},
issue_date = {6 2018},
volume = {6},
Issue = {6},
month = {6},
year = {2018},
issn = {2347-2693},
pages = {809-815},
url = {https://www.ijcseonline.org/full_paper_view.php?paper_id=2260},
doi = {https://doi.org/10.26438/ijcse/v6i6.809815}
publisher = {IJCSE, Indore, INDIA},
}
RIS Style Citation:
TY - JOUR
DO = {https://doi.org/10.26438/ijcse/v6i6.809815}
UR - https://www.ijcseonline.org/full_paper_view.php?paper_id=2260
TI - Divergence Based Generalized Fuzzy Rough Sets
T2 - International Journal of Computer Sciences and Engineering
AU - Sheeja T.K., Sunny Kuriakose A.
PY - 2018
DA - 2018/06/30
PB - IJCSE, Indore, INDIA
SP - 809-815
IS - 6
VL - 6
SN - 2347-2693
ER -
![]() |
![]() |
![]() |
348 | 248 downloads | 218 downloads |
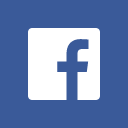
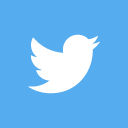
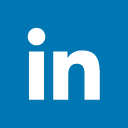
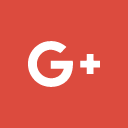
Abstract
Fuzzy set theory and rough set theory are two formal mathematical tools to handle vagueness, imperfection or incompleteness in data. Fuzzy rough set theory is an embodiment of the prime features of both the theories. This hybrid theory has been proved to be an effective tool for data mining, particularly for feature selection. In this paper, generalized fuzzy rough approximations based on divergence measure of fuzzy sets in an information system is defined using a fuzzy implicator and a fuzzy t-norm. Also, the properties of the fuzzy rough approximations are investigated. Further, an algorithm for feature selection using the fuzzy boundary region of the proposed approximations is presented and experimented with twelve real data sets.
Key-Words / Index Term
Information System, Approximations, Divergence Measure, Fuzzy rough set, Feature selection
References
[1] S. Sumathi and S.N. Sivanandam, "Introduction to Data Mining and its Applications", Springer, Berlin, 2006.
[2] Sonali Suskar, S. D. Babar , "Survey on Feature Selection for Text Categorization", International Journal of Scientific Research in Computer Science, Engineering and Information Technology, Vol.3, Issue.4, pp.261-266, 2018.
[3] P. Rutravigneshwaran , "A Study of Intrusion Detection System using Efficient Data Mining Techniques", International Journal of Scientific Research in Network Security and Communication, Vol.5, Issue.6, pp.5-8, 2017.
[4] L. A. Zadeh, "Fuzzy Sets", Information and Control, Vol.8, Issue.3, pp.338-353, 1965.
[5] Z. Pawlak, "Rough Sets", International Journal of Computer and Information Sciences, Vol.11, Issue.5, pp.341 - 356, 1982.
[6] S. Vluymans, L. D`eer, Y. Saeys, C. Cornelis, "Applications of Fuzzy Rough Set Theory in Machine Learning: a Survey", Fundamenta Informaticae, pp.1-34, 2015.
[7] D. Dubois, H. Prade, "Rough Fuzzy Sets and Fuzzy Rough Sets", International Journal of General Systems, Vol.17, pp.191-209, 1990.
[8] J. S. Mi, Y. Leung and T. Feng, "Generalized Fuzzy Rough Sets Determined by a Triangular Norm", Information Sciences, Vol.178, pp.3203-3213, 2008.
[9] N. M. Parthalain, R. Jensen and Q. Shen, "Fuzzy entropy assisted fuzzy-rough feature selection", in Proceedings of 2006 IEEE International Conference on Fuzzy Systems, pp.1499-1506, 2006.
[10] A. Radzikowska and E. E. Kerre, "A comparative Study of Fuzzy Rough Sets", Fuzzy Sets and Systems, Vol.126, pp.137-155, 2002.
[11] W. Z. Wu, J. S. Mi and W. X. Zhang, "Generalized Fuzzy Rough Sets", Information Sciences, Vol.151, pp.263-282, 2003.
[12] Sheeja T. K. and A. Sunny Kuriakose, "A novel feature selection method using fuzzy rough sets", Computers in Industry, Vol.97, pp.111 - 116, 2018.
[13] J. Klir and B. Yuan, "Fuzzy Sets and Fuzzy Logic", Prentice Hall, New Jersey,1995.
[14] Z. Pawlak, "Rough Sets - Theoretical Aspect of Reasoning About Data", Kluwer Academic Publishers, The Netherlands, 1991.
[15] S. Montes, I. Couso, P. Gil and C. Bertoluzza, "Divergence Measure Between Fuzzy Sets", International Journal of Approximate Reasoning, Vol.30, pp.91-105, 2002.
[16] S. An, Q. Hu and D. Yu, "A Robust Rough Set Model Based on Minimum Enclosing Ball", In Rough Sets and Knowledge Discovery, LNAI 6401 eds J. Yu et al., pp.102-109, Springer - Verlag, Berlin 2010.
[17] C. Chornelis, N. Verdiest and R. Jensen, "Ordered Weighted Average Based Fuzzy Rough Sets", In Rough Sets and Knowledge Discovery, LNAI 6401, eds J. Yu et al., pp.78-85, (Springer - Verlag, Berlin 2010.
[18] Q. Hu, D. Chen, D. Yu and W. Pedrycz, "Kernelized Fuzzy Rough Sets", In Rough Sets and Knowledge Discovery, LNCS 5589, eds P. Wen et al. pp.304-311, 2009 (Springer-Verlag, Berlin).
[19] L. Deer, N. Verbiest, C. Cornelis and L. Godo, "A comprehensive study of implicator-conjunctor-based and noise-tolerant fuzzy rough sets: Definitions, properties and robustness analysis", Fuzzy Sets and Systems, Vol.275, pp.1-38, 2015.
[20] R. Jensen and Q. Shen, "Fuzzy-rough attribute reduction with application to web categorization", Fuzzy Sets and Systems, Vol.141, issue.3, pp.469-485, 2004.
[21] R. Jensen and Q. Shen, "Fuzzy-rough data reduction with ant colony optimization", Fuzzy Sets and Systems, Vol.149, Issue.1, pp.5- 20, 2005.
[22] R. Jensen and Q. Shen, "New approaches to fuzzy-rough feature selection", IEEE Transactions on Fuzzy Systems, Vol.17, Issue.4, pp.824-838, 2009.
[23] R. Jensen and Q. Shen, "Fuzzy-rough sets assisted feature selection", IEEE Transactions on Fuzzy Systems, Vol.15, Issue.1, pp.73 - 89, 2007.
[24] C. C. Eric, D. Chen, D.S.Yeung, X. Z. Wang and W.T. John, "Attributes reduction using fuzzy rough sets", IEEE Transactions on Fuzzy Systems, Vol.16, Issue.5, pp.1130-1141, 2008.
[25] N. M.Parthalain, Q. Shen and R. Jensen, "A distance measure approach to exploring the rough set boundary region for attribute reduction", IEEE Transactions on Knowledge and Data Engineering, Vol.22, Issue.3, pp.305-317, 2010.
[26] M. Lichman, UCI Machine Learning Repository [http://archive.ics.uci.edu/ml]. Irvine, CA: University of California, School of Information and Computer Science 2013.
[27] C. Armanino, R. Leardi, S. Lanteri and G. Modi, "Chemometrics and Intelligent Laboratory Systems" Vol.5, pp.343-354, 1989.
[28] H. T. Kahraman, S. Sagiroglu and I.Colak, "Developing intuitive knowledge classifier and modeling of users` domain dependent data in web", Knowledge Based Systems, Vol.37, pp.283-295, 2013.
[29] A. Tsanas and A. Xifara, "Accurate quantitative estimation of energy performance of residential buildings using statistical machine learning tools", Energy and Buildings, Vol.49, pp.560-567, 2012.
[30] C. Mallah, J. Cope and J. Orwell, "Plant Leaf Classification Using Probabilistic Integration of Shape, Texture and Margin Features", Signal Processing, Pattern Recognition and Applications, 2013.
[31] P. Cortez, A. Cerdeira, F. Almeida, T. Matos and J. Reis, "Modeling wine preferences by data mining from physicochemical properties", Decision Support Systems, Elsevier, Vol.47, Issue.4, pp.547-553, 2009.