A hybrid filter-wrapper feature selection method for stress detection and monitoring among employees at workspaces
Monika 1 , Anita Sahoo2
Section:Research Paper, Product Type: Journal Paper
Volume-6 ,
Issue-6 , Page no. 831-836, Jun-2018
CrossRef-DOI: https://doi.org/10.26438/ijcse/v6i6.831836
Online published on Jun 30, 2018
Copyright © Monika, Anita Sahoo . This is an open access article distributed under the Creative Commons Attribution License, which permits unrestricted use, distribution, and reproduction in any medium, provided the original work is properly cited.
View this paper at Google Scholar | DPI Digital Library
How to Cite this Paper
- IEEE Citation
- MLA Citation
- APA Citation
- BibTex Citation
- RIS Citation
IEEE Citation
IEEE Style Citation: Monika, Anita Sahoo, “A hybrid filter-wrapper feature selection method for stress detection and monitoring among employees at workspaces,” International Journal of Computer Sciences and Engineering, Vol.6, Issue.6, pp.831-836, 2018.
MLA Citation
MLA Style Citation: Monika, Anita Sahoo "A hybrid filter-wrapper feature selection method for stress detection and monitoring among employees at workspaces." International Journal of Computer Sciences and Engineering 6.6 (2018): 831-836.
APA Citation
APA Style Citation: Monika, Anita Sahoo, (2018). A hybrid filter-wrapper feature selection method for stress detection and monitoring among employees at workspaces. International Journal of Computer Sciences and Engineering, 6(6), 831-836.
BibTex Citation
BibTex Style Citation:
@article{Sahoo_2018,
author = {Monika, Anita Sahoo},
title = {A hybrid filter-wrapper feature selection method for stress detection and monitoring among employees at workspaces},
journal = {International Journal of Computer Sciences and Engineering},
issue_date = {6 2018},
volume = {6},
Issue = {6},
month = {6},
year = {2018},
issn = {2347-2693},
pages = {831-836},
url = {https://www.ijcseonline.org/full_paper_view.php?paper_id=2264},
doi = {https://doi.org/10.26438/ijcse/v6i6.831836}
publisher = {IJCSE, Indore, INDIA},
}
RIS Citation
RIS Style Citation:
TY - JOUR
DO = {https://doi.org/10.26438/ijcse/v6i6.831836}
UR - https://www.ijcseonline.org/full_paper_view.php?paper_id=2264
TI - A hybrid filter-wrapper feature selection method for stress detection and monitoring among employees at workspaces
T2 - International Journal of Computer Sciences and Engineering
AU - Monika, Anita Sahoo
PY - 2018
DA - 2018/06/30
PB - IJCSE, Indore, INDIA
SP - 831-836
IS - 6
VL - 6
SN - 2347-2693
ER -
![]() |
![]() |
![]() |
771 | 371 downloads | 175 downloads |
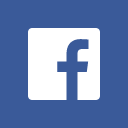
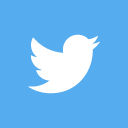
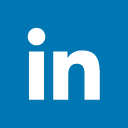
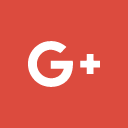
Abstract
In this competitive world, employees often experience stress at work. Stress for a prolonged period of time is converted to chronic stress. This may lead to high blood pressure, damage to muscle tissue, inhibition of growth, suppression of the immune system and damage to mental health. Generally, stress management is subjective to the realization of the person. For a better mental health management, continuous monitoring and objective evaluation of stress is a need. Nowadays, various sensors are used for the same. This paper investigates how new context-aware pervasive systems can support knowledge workers to diminish stress. The focus is on developing an automatic classifier to infer working conditions and stress-related mental states from a multimodal set of sensor data (computer logging, facial expressions, posture, and physiology). Instead of using all the sensor data (149 features), the further focus is on selecting a subset of features, which are most effective in detecting stress using a hybrid filter-wrapper approach for feature selection. As a final note, implementing such a stress detection system in real-world settings brings additional challenges. Not only sensors have to be installed to collect data in the workplace, but also the signals need to be processed, features extracted and analyzed in real time yielding meaningful results. But selecting a set of features makes the task a lot easier and results in higher accuracy and fast processing. Different filter and wrapper methods and their hybrids were analyzed for the problem at hand. Finally, the hybrid of information gain and best first method resulted in a significant reduction in the number of features in the original feature set and an increase in accuracy.
Key-Words / Index Term
Machine learning, Stress, Feature selection, Hybrid method, Facial expression, Postures, Computer loggings, Physiological, Filter approach, Wrapper approach
References
[1] Atlee Fernandes, Rakesh Helawar, R. Lokesh, Tushar Tari and Ashwini V. Shahapurkar, “Determination of Stress using Blood Pressure and Galvanic Skin Response”, International Conference on Communication and Network Technologies (ICCNT), pp. 165-168 ,2014
[2] G. Prashanti, Mujafar Abdul Ghani, "An Efficient Analysis of Psychological Stress Prediction Technique Using Social Interaction of Social Networks ", International Journal of Scientific Research in Computer Science, Engineering and Information Technology (IJSRCSEIT), ISSN : 2456-3307, Volume 4, Issue 2, pp.87-94, March-April.2018.
[3] P. Arumugam , P. Jose, "Efficient Feature Selection and Classification Technique For Large Data", International Journal of Scientific Research in Computer Science, Engineering and Information Technology (IJSRCSEIT), ISSN : 2456-3307, Volume 2, Issue 2, pp.1041-1047, March-April.2017.
[4] S. Koldijk, M. Sappelli, S. Verberne, Mark A. Neerincx and W. Kraaij, “The SWELL Knowledge Work Dataset for Stress and User Modeling Research”, 16th International Conference on Multimodal Interaction, pp. 291-298, Nov 2014.
[5] Lisa M.Vizer, Lina Zhou and Andrew Sears “Automated stress detection using keystroke and linguistic features: An exploratory study” International Journal of Human-Computer Studies Volume 67, Issue 10, pp. 870-886, October 2009
[6] Javier Hernandez, Pablo Paredes, Asta Roseway and Mary Czerwinski “Under pressure: sensing stress of computer users” SIGCHI Conference on Human Factors in Computing Systems, pp. 51-60, May 2014
[7] Hua Gao, Anil Yüce and Jean-Philippe Thiran “Detecting emotional stress from facial expressions for driving safety” IEEE International Conference on Image Processing (ICIP), pp. 5961-5965, Oct 2014
[8] Dinges DF, Rider RL, Dorrian J, McGlinchey EL, Rogers NL, Cizman Z, Goldenstein SK, Vogler C, Venkataraman S, Metaxas DN. “Optical Computer Recognition of Facial Expressions Associated with Stress Induced by Performance Demands” Aviat Space Environ Med., pp. 172-82, Jun2005.
[9] Feng-Tso SunCynthia KuoHeng-Tze ChengSenaka Buthpitiya Patricia Collins and Martin Griss . “Activity-Aware Mental Stress Detection Using Physiological Sensors”, Part of the Lecture Notes of the Institute for Computer Sciences, Social Informatics and Telecommunications Engineering book series (LNICST, volume 76), pp.211-230.
[10] Dimitris Giakoumis, Anastasios Drosou, Pietro Cipresso, Dimitrios Tzovaras, George Hassapis, Andrea Gaggioli, Giuseppe Riva. "Using Activity-Related Behavioural Features towards More Effective Automatic Stress Detection”, PLoS ONE 7(9): e43571.,September 2012.
[11] Setz, C., Arnrich, B., Schumm, J., La Marca, R., Troster, G., & Ehlert, U. “Discriminating stress from cognitive load using a wearable eda device.”, Information Technology in Biomedicine, IEEE Transactions on, pp. 410-417,2010
[12] R. LiKamWa, Y. Liu, N. D. Lane, and L. Zhong, “MoodScope: Building a Mood Sensor from Smartphone Usage Patterns,” in ACM MobiSys, pp. 389–402, 2013.
[13] Nicolaj SÃÿndberg-madsen, Casper Thomsen, and Jose M. PeÃsa. Unsupervised feature subset selection. In In Proceedings of the Workshop on Probabilistic Graphical Models for Classification, pp. 71–82, 2003.
[14] George H John, Ron Kohavi, and Karl P fleger,“Irrelevant features and the subset selection problem” ICML volume 129, pp. 121–129, 1994.