An Investigation of Occupational stress Classification by using Machine Learning Techniques
S.K. Yadav1 , Arshad Hashmi2
Section:Research Paper, Product Type: Journal Paper
Volume-6 ,
Issue-6 , Page no. 842-850, Jun-2018
CrossRef-DOI: https://doi.org/10.26438/ijcse/v6i6.842850
Online published on Jun 30, 2018
Copyright © S.K. Yadav, Arshad Hashmi . This is an open access article distributed under the Creative Commons Attribution License, which permits unrestricted use, distribution, and reproduction in any medium, provided the original work is properly cited.
View this paper at Google Scholar | DPI Digital Library
How to Cite this Paper
- IEEE Citation
- MLA Citation
- APA Citation
- BibTex Citation
- RIS Citation
IEEE Style Citation: S.K. Yadav, Arshad Hashmi, “An Investigation of Occupational stress Classification by using Machine Learning Techniques,” International Journal of Computer Sciences and Engineering, Vol.6, Issue.6, pp.842-850, 2018.
MLA Style Citation: S.K. Yadav, Arshad Hashmi "An Investigation of Occupational stress Classification by using Machine Learning Techniques." International Journal of Computer Sciences and Engineering 6.6 (2018): 842-850.
APA Style Citation: S.K. Yadav, Arshad Hashmi, (2018). An Investigation of Occupational stress Classification by using Machine Learning Techniques. International Journal of Computer Sciences and Engineering, 6(6), 842-850.
BibTex Style Citation:
@article{Yadav_2018,
author = {S.K. Yadav, Arshad Hashmi},
title = {An Investigation of Occupational stress Classification by using Machine Learning Techniques},
journal = {International Journal of Computer Sciences and Engineering},
issue_date = {6 2018},
volume = {6},
Issue = {6},
month = {6},
year = {2018},
issn = {2347-2693},
pages = {842-850},
url = {https://www.ijcseonline.org/full_paper_view.php?paper_id=2266},
doi = {https://doi.org/10.26438/ijcse/v6i6.842850}
publisher = {IJCSE, Indore, INDIA},
}
RIS Style Citation:
TY - JOUR
DO = {https://doi.org/10.26438/ijcse/v6i6.842850}
UR - https://www.ijcseonline.org/full_paper_view.php?paper_id=2266
TI - An Investigation of Occupational stress Classification by using Machine Learning Techniques
T2 - International Journal of Computer Sciences and Engineering
AU - S.K. Yadav, Arshad Hashmi
PY - 2018
DA - 2018/06/30
PB - IJCSE, Indore, INDIA
SP - 842-850
IS - 6
VL - 6
SN - 2347-2693
ER -
![]() |
![]() |
![]() |
593 | 294 downloads | 127 downloads |
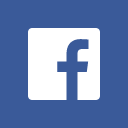
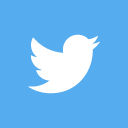
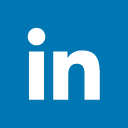
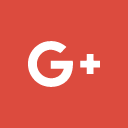
Abstract
Occupational stress can impact our lives in several aspects. This affects employee’s health, causes absenteeism and overall performance of an organization affected. World Health Organization (WHO) identifies it as epidemics for the modern life. The insurance sector employees have direct customer interaction. The policies and the services introduced to the new customers, convincing the ideas and satisfying the divergent customer needs causes more pressure on the employees which leads to higher level of stress. Occupational stress data mining is an emerging stream which helps in mining stressed data for solving various types of problem. One of the problems is to know the impact of role overload and role ambiguity on occupational stress. In this paper, we have tried to implement a model using machine learning classification techniques for the prediction of Occupational stress related to insurance sector personnel. In this paper, we have applied support vector machine (SVM), Neural network (NN), decision tree (DT) and random forest (RF). The training and testing are done through a stratified tenfold cross-validation. The proposed model obtained an accuracy of 60%, a sensitivity of 80%, and specificity 60%. The evaluation of occupational stress is critically connected to job performance in the organization. So it is essential to identify the causes of occupational stress and can be reduced to the possible extent with the help of proper management techniques.
Key-Words / Index Term
Occupational Stress, Distress, Predictive model, Classification techniques, SVM, NN, DT, RF
References
[1] R. PJ. “The health effects of job stress”. Bus Health. PubMed, Vol.1Issue.6, 198, pp.5–8,1984
[2] European Commission, “Guidance on Work-related Stress”, Health and safety at work, Luxembourg, Directorate-General for
Employment and Social Affairs , Unit D.6, 1999
[3] WHO, “Raising awareness of stress at work in developing countries, a modern hazard in a traditional working environment,
Protecting workers”,Health series; no. 6. 2007
[4] Allison G., “Perceived and Reported Occupational stressors and Coping Strategies of Selected Community College Business Faculty Members in Texas”, University of Texas, United States; 2004 PhD dissertation.
[5] Bevilacqua, M., Ciarapica, F.E., Giacchetta, G, “Industrial and Occupational Ergonomics in The Petrochemical Process
Industry: A Regression Trees Approach”, Accid. Anal. Prev. Vol.40, pp.1468-1479, 2008.
[6] Parhizi, S., Shahrabi, J., Pariazar, M., “A New Accident Investigation Approach Based On Data Mining Techniques”, J. Appl. Science, Vol.9, pp. 731-737, 2009,
[7] Persona, A., Battini, D., Faccio, M., Bevilacqua, M., Ciarapica, F.E., “Classification Of Occupational Injury Cases Using The Regression Tree Approach”. Int. J. Reliab. Qual. Saf. Eng.Vol.13, pp.171-191, 2006.
[8] Giudici, P., “Applied Data Mining”: Statistical Methods for Business and Industry. Wiley, New York, 2003
[9] Han, J., Kamber, M. Data Mining: “Concepts and Techniques”, Morgan Kaufmann, San Francisco. 2001
[10] Hand, D., Mannila, H., Smyth, P., “Principles of Data Mining”. MIT Press, Cambridge (Mass.). 2001
[11] Karasek, R., Gordon, G., Pietrokovsky, C., Rrese, M., Pieper, C., Schwartz, J., Fry, L., Schirer, D., “Job Content Questionnaire: Questionnaire and User’s Guide”, University of Massachusetts, Lowell, 1985
[12] Krishna OB, Jhareswar Maiti, Pradip K. Ray, Biswajit Samanta, Saptarshi Mandal, Sobhan Sarkar, “Measurement and Modeling of Job Stress of Electric Overhead Traveling Crane Operators”, Science Direct, http://dx.doi.org/10.1016/j.shaw.2015.06.005, 2015
[13] Arezou Khaleghi, Maryam Omidi Najafabadi, Frahad Lashgarara, “Effective Factors on Job Stress from Experts’ Perception; a Case Study in Iranian Agriculture Engineering Organization”, International Journal of Review in Life Sciences, Vol.5,Issue1, pp.94-99, 2015.
[14] Aytac Serpil, The Sources of Stress, The Symptoms of Stress and Anger Styles as a Psychosocial Risk at Occupational Health
[15] and Safety: A Case Study on Turkish Police Officers. 6th International Conference on Applied Human Factors and Ergonomics (AHFE 2015) and the Affiliated Conferences. Procedia Manufacturing Journal, 3, 6421 – 6428, 2015
[16] Mohd Zuri Ghani, Aznan Che Ahmad, Suzana Ibrahim ,Stress among Special Education Teachers in Malaysia, Science Direct, Procedia - Social and Behavioral Sciences 114, 2014 , 4 – 13
[17] Che Noriah Othman, Roz Azinur Che Lamin, Nursyuhadah Othman, Occupational Stress Index of Malaysian University Workplace, Procedia - Social and Behavioral Sciences 153 ,2014 , 700 – 710
[18] Shaghayegh Parhizi, Linsey M. Steege, Kalyan S. Pasupathy, Mining the relationships between psychosocial factors and fatigue dimensions among registered nurses, International Journal of Industrial Ergonomics 43, 2013, 82-90
[19] Maryam Khodabakhshi, Predicting occupational stress for women working in the bank with assessment of their organizational commitment and personality type, Procedia -Social and Behavioral Sciences,84,2013,1859–1863
[20] Susana Garcia-Herrero, M.A.Mariscal, J.M.Gutiérrez, DaleO.Ritzel, Using Bayesian networks to analyze occupational stress caused by work demands- Preventing stress through social support, Science Direct, Accident Analysis and Prevention 57, 2013, 114– 123
[21] Yong Deng, D. frank hsu, Zhonghai wu, Chaohsien chu , Combining multiple sensor features for stress detection using
[22] combinatorial fusion, journal of interconnection networks, 1250008, 2012,17 pages, doi: 10.1142/s0219265912500089
[23] Shahina Begum, Mobyen Uddin Ahmed, Peter Funk, Ning Xiong, Bo von Schéele, A Case-Based Decision Support System for Individual Stress Diagnosis Using Fuzzy Similarity Matching, Computational Intelligence, vol 25,nr 3, 2009,p180-195
[24] Arie Shirom, Simona Shechter Gilboa, Yitzhak Fried and Cary L. Cooper, Gender, age and tenure as moderators of work-related stressors’ relationships with job performance: A meta-analysis, SAGE Publications, Human Relations, 61(10), 2008, 1371–1398, DOI: 10.1177/0018726708095708
[25] Eric G. Lambert, Nancy L. Hogan, Marie L. Griffin, The impact of distributive and procedural justice on correctional staff job stress, job satisfaction, and organizational commitment, Science Direct, Journal of Criminal Justice,35, 2007,,644–656
[26] Jing Zhai, C. Chin, Chao Li, Realization of stress detection using psychophysiological signals for improvement of human-computer interactions, IEEE Xplore Conference, 2005, DOI:10.1109/SECON.2005.1423280
[27] Vedat Isikhan, Turhan Comez, M. Zafer Danis, Job stress and coping strategies in health care professionals working with cancer patients European Journal of Oncology Nursing Society,8, 2004, 234–244
[28] Noora Nenonen, Analysing factors related to slipping, stumbling, and falling accidents at work: Application of data mining methods to Finnish occupational accidents and diseases statistics database, Applied Ergonomics, 44, 2013, 215-224
[29] ] J. Bakker, L. Holenderski, R. Kocielnik, M. Pechenizkiy, N. Sidorova ,TU Eindhoven, Stess@work: From Measuring Stress to its Understanding, Prediction and Handling with Personalized Coaching, ACM, IHI12, 2012, 28–30
[30] Ming Jiang, Zhelong Wang, A Method for Stress Detection Based on FCM Algorithm, IEEE,DOI: 10.1109/CISP.2009.5304150,2009
[31] Paul Bowen, Peter Edwards, Helen Lingard, Keith Cattell, Occupational stress and job demand, control and support factors among construction project consultants, International Journal of Project Management, 32, 2014, 1273–1284
[32] Frawley, W., Piatetsky, G.S., Matheus, C., Knowledge discovery in databases: an overview. AI Magazine Fall, 213-228, 1992]
[33] Quinlan J.R., Induction of decision trees. Machine learning, Vol.1, 81-106, 1986
[34] Quinlan, 1992 Quinlan J.R, Learning with continuous classes, Proc. of Australian Joint Conf. on AI, 343-348, World Scientific, Singapore. . 1992
[35] Chapman, P., Clinton, J., Kerber, R., Khabaza, T., Reinartz, T., Shearer, C., Crisp-DM 1.0: Step-by-step data mining guide. http://www.crisp-dm.org/ Retrieved November, 2009.
[36] Ammo,T. & Friedman, H. H, Overall Evaluation Rating Scale: An Assessment, International Journal of Marketing Research,43(3), 301-310, 2002
[37] Cronbach, L. J, Coefficient alpha and Internal Structure of Tests, Psychometrika, 16 (3), 297-333, 1951
[38] Travis C.Tubrea, Judith M.Collins, Jackson and schuler(1985), Revisited-a meta-analysis of the relationships between role ambiguity, role conflict, and job performance, Journal of Management,26(1),155-169,2000, https://doi.org/10.1016/S0149-2063(99)00035
[39] Gray Balau, Testing the Longitudinal Impact of Work variables and Performance Appraisal Satisfaction on Subsequent Overall job Satisfaction, Human relation, 52(8), 2009
[40] Carly S.Bruck,Tammy D.Allen,and Paul E.Spector,The Relation between Work–Family Conflict and Job Satisfaction-A Finer-Grained Analysis,Journal of vocational Behavior 60, 336–353, 2002,doi:10.1006/jvbe.2001.1836,available online at http://www.idealibrary.com
[41] J. Han, M. Kamber, and J. Pei, Data Mining. Concepts and techniques, Third. Morgan Kaufmann, 2012
[42] M. A. Hall, Correlation-based Feature Selection for Machine Learning, The University of Waikato, 1999
[43] ] R. Kohavi and G. H. John, Wrappers for feature subset selection, Artif. Intell. 97(1, 2), 273–324, 1997
[44] I. H. Witten, E. Frank, and M. Hall, Data Mining: Practical Machine Learning Tools and Techniques, 40(6), Third Edit., 2001
[45] N. V Chawla, K. W. Bowyer, L. O. Hall, and W. P. Kegelmeyer, SMOTE: Synthetic Minority
[46] Over-sampling Technique, J. Artif. Intell. Res., 16, 321–357, 2002
[47] A. More, Survey of resampling techniques for improving classification performance in unbalanced
datasets, 10000, 1–7, 2016, https://arxiv.org/pdf/1608.06048.pdf
[48] Hall M.A., Frank E., Holmes G., Pfahringer B., Reutemann, P. and Witten, I.H, The WEKA Data Mining Software: An Update, SIGKDD Explorations. 11(1), 10-18.2009
[49] O. Loyola, M. A. Medina, and M. García, Inducing Decision Trees based on a Cluster Quality Index, IEEE Lat. Am. Trans.,13(4), 1141–1147, 2015
[50] P. Univaso, J. M. Ale, and J. A. Gurlekian, Data mining applied to forensic speaker identification, IEEE Lat. Am. Trans., 13(4), 1098–1111, 2015.
[51] G. H. John and P. Langley, Estimating continuous distributions in Bayesian classifiers. UAI’95 Proc. Elev. Conf. Uncertain. Artif. Intell. , 338–345, 1995
[52] J. C. Platt, Fast training of support vector machines using sequential minimal optimization, in Advances in Kernel Methods: Support Vector Learning, C. J. C. Burges, and A. J.Smola, Eds. MIT Press, 185–208, 1999.
[53] B. Schölkopf and A. J. Smola, Learning with kernels: support vector machines, regularization, optimization, MIT Press, 2002.
[54] D. W. Aha, D. Kibler, and M. K. Albert, Instance-based learning algorithms, Mach. Learn., 6(1), 37–66,1991.
[55] I. H. Witten, E. Frank, and M. Hall, Data Mining: Practical Machine Learning Tools and Techniques, Third Edit., 40(6), 2001.
[56] Dickinson T, Wrigiit M. Stress and burnout in forensic mental nursing: A literature review. Br J Nurs. PubMed 17, 82–7., 2008
[57] Habrani P, Behdad F, Mobtaker M. Investigation of stressful factors in nurses., J Psychiatric Health Principles.3:231–7. 2008
[58] Kolagari SH, Sanagou A, Mirkarimi F, Behnampour N. The level of stress among gorgan university of medical sciences hospital operating rooms personals and its relation to some related factors. J Gorgan Univ Med Sci.10:54–9. 2002