Predictive Modelling for Credit Risk Detection using Ensemble Method
Anand Motwani1 , Goldi Bajaj2 , Sushila Mohane3
Section:Research Paper, Product Type: Journal Paper
Volume-6 ,
Issue-6 , Page no. 863-867, Jun-2018
CrossRef-DOI: https://doi.org/10.26438/ijcse/v6i6.863867
Online published on Jun 30, 2018
Copyright © Anand Motwani, Goldi Bajaj, Sushila Mohane . This is an open access article distributed under the Creative Commons Attribution License, which permits unrestricted use, distribution, and reproduction in any medium, provided the original work is properly cited.
View this paper at Google Scholar | DPI Digital Library
How to Cite this Paper
- IEEE Citation
- MLA Citation
- APA Citation
- BibTex Citation
- RIS Citation
IEEE Style Citation: Anand Motwani, Goldi Bajaj, Sushila Mohane, “Predictive Modelling for Credit Risk Detection using Ensemble Method,” International Journal of Computer Sciences and Engineering, Vol.6, Issue.6, pp.863-867, 2018.
MLA Style Citation: Anand Motwani, Goldi Bajaj, Sushila Mohane "Predictive Modelling for Credit Risk Detection using Ensemble Method." International Journal of Computer Sciences and Engineering 6.6 (2018): 863-867.
APA Style Citation: Anand Motwani, Goldi Bajaj, Sushila Mohane, (2018). Predictive Modelling for Credit Risk Detection using Ensemble Method. International Journal of Computer Sciences and Engineering, 6(6), 863-867.
BibTex Style Citation:
@article{Motwani_2018,
author = {Anand Motwani, Goldi Bajaj, Sushila Mohane},
title = {Predictive Modelling for Credit Risk Detection using Ensemble Method},
journal = {International Journal of Computer Sciences and Engineering},
issue_date = {6 2018},
volume = {6},
Issue = {6},
month = {6},
year = {2018},
issn = {2347-2693},
pages = {863-867},
url = {https://www.ijcseonline.org/full_paper_view.php?paper_id=2269},
doi = {https://doi.org/10.26438/ijcse/v6i6.863867}
publisher = {IJCSE, Indore, INDIA},
}
RIS Style Citation:
TY - JOUR
DO = {https://doi.org/10.26438/ijcse/v6i6.863867}
UR - https://www.ijcseonline.org/full_paper_view.php?paper_id=2269
TI - Predictive Modelling for Credit Risk Detection using Ensemble Method
T2 - International Journal of Computer Sciences and Engineering
AU - Anand Motwani, Goldi Bajaj, Sushila Mohane
PY - 2018
DA - 2018/06/30
PB - IJCSE, Indore, INDIA
SP - 863-867
IS - 6
VL - 6
SN - 2347-2693
ER -
![]() |
![]() |
![]() |
601 | 330 downloads | 210 downloads |
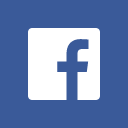
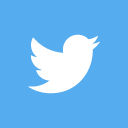
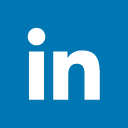
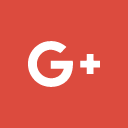
Abstract
With the expansion of credit business, the prediction models for taking decision of credit permissions with least risk are becoming more and more admired by banking sectors. The use of Machine Learning (ML) based models has confirmed to be of practical value in resolving a range of banking risk prediction problems. The model for Credit risk prediction seeks to predict feature factors, whether an individual is bad or good applicant for loan or not. Such problems can be better solved using ML. Also, Ensemble classifiers in ML play a key role in prediction problems. The use of Ensemble Methods (EMs) for classification is among the recent areas of research in ML. Many recent researches specify that EMs lead to a major improvement in classification performance by choosing suitable class. For this work, several ML techniques are explored and evaluated on real credit card datasets. Most ML methods have achieved an accuracy of less than 80 percent. Predictive model for Credit Risk Detection based on ensemble technique is proposed. The proposed model is evaluated on basis of various performance metrics and comparison is done with base classifier (learner) resulted in 81 percent prediction accuracy and better correlation coefficient.
Key-Words / Index Term
Predictive Modelling, Machine Ensemble Method, Credit Risk, Data Mining
References
[1] Polikar, R. (2006). Ensemble based systems in decision making. IEEE Circuits and Systems Magazine, 6(3), 21–45.
[2] Zhou, Z. H. (2009). Ensemble. In L. Liu & T. Özsu (Eds.), Encyclopedia of database systems. Berlin: Springer.
[3] Loris Nanni , Alessandra Lumini, “An experimental comparison of ensemble of classifiers for bankruptcy prediction and credit scoring”, Elsevier, Expert Systems with Applications 36 (2009) 3028–3033.
[4] David Opitz and Richard Maclin, “Popular Ensemble Methods: An Empirical Study”, Journal of artificial intelligence research 169-198, 1999.
[5] Freund, Y., & Schapire, R. (1996). Experiments with a new boosting algorithm. In Proceedings of the thirteenth international conference on machine learning, Bari, Italy (pp. 148–156).
[6] Wolpert, D. H. (1992). Stacked generalization. Neural Networks, 5(2), 241–259.
[7] Regina Esi Turkson, Edward Yeallakuor Baagyere, Gideon Evans Wenya, “A Machine Learning Approach for Predicting Bank Credit Worthiness”, ISBN: 978-1-4673-9187-0, IEEE 2016
[8] [8] U Bhuvaneswari, P. James Daniel Paul, Siddhant Sahu, “Financial Risk Modelling in Vehicle Credit Portfolio”, 978-1-4799-4674-7/14/$31.00, IEEE 2014
[9] C.R.Durga devi, Dr.R.Manicka chezian, “A Relative Evaluation of the Performance of Ensemble Learning in Credit Scoring”, IEEE International Conference on Advances in Computer Applications (ICACA), 978-1-5090-3770-4/16, 2016
[10] Gang Wang, Jinxing Hao, Jian Mab, Hongbing Jiang, “A comparative assessment of ensemble learning for credit scoring”, Expert Systems with Applications 38 (2011) 223–230
[11] Breiman, L. (1996). Bagging predictors. Machine Learning, 123–140.