Review of Traffic Forecasting Approaches in Software Defined Mobile Networks
Anupriya 1 , Anita Singhrova2
Section:Review Paper, Product Type: Journal Paper
Volume-6 ,
Issue-6 , Page no. 1002-1009, Jun-2018
CrossRef-DOI: https://doi.org/10.26438/ijcse/v6i6.10021009
Online published on Jun 30, 2018
Copyright © Anupriya, Anita Singhrova . This is an open access article distributed under the Creative Commons Attribution License, which permits unrestricted use, distribution, and reproduction in any medium, provided the original work is properly cited.
View this paper at Google Scholar | DPI Digital Library
How to Cite this Paper
- IEEE Citation
- MLA Citation
- APA Citation
- BibTex Citation
- RIS Citation
IEEE Citation
IEEE Style Citation: Anupriya, Anita Singhrova, “Review of Traffic Forecasting Approaches in Software Defined Mobile Networks,” International Journal of Computer Sciences and Engineering, Vol.6, Issue.6, pp.1002-1009, 2018.
MLA Citation
MLA Style Citation: Anupriya, Anita Singhrova "Review of Traffic Forecasting Approaches in Software Defined Mobile Networks." International Journal of Computer Sciences and Engineering 6.6 (2018): 1002-1009.
APA Citation
APA Style Citation: Anupriya, Anita Singhrova, (2018). Review of Traffic Forecasting Approaches in Software Defined Mobile Networks. International Journal of Computer Sciences and Engineering, 6(6), 1002-1009.
BibTex Citation
BibTex Style Citation:
@article{Singhrova_2018,
author = {Anupriya, Anita Singhrova},
title = {Review of Traffic Forecasting Approaches in Software Defined Mobile Networks},
journal = {International Journal of Computer Sciences and Engineering},
issue_date = {6 2018},
volume = {6},
Issue = {6},
month = {6},
year = {2018},
issn = {2347-2693},
pages = {1002-1009},
url = {https://www.ijcseonline.org/full_paper_view.php?paper_id=2289},
doi = {https://doi.org/10.26438/ijcse/v6i6.10021009}
publisher = {IJCSE, Indore, INDIA},
}
RIS Citation
RIS Style Citation:
TY - JOUR
DO = {https://doi.org/10.26438/ijcse/v6i6.10021009}
UR - https://www.ijcseonline.org/full_paper_view.php?paper_id=2289
TI - Review of Traffic Forecasting Approaches in Software Defined Mobile Networks
T2 - International Journal of Computer Sciences and Engineering
AU - Anupriya, Anita Singhrova
PY - 2018
DA - 2018/06/30
PB - IJCSE, Indore, INDIA
SP - 1002-1009
IS - 6
VL - 6
SN - 2347-2693
ER -
![]() |
![]() |
![]() |
517 | 280 downloads | 153 downloads |
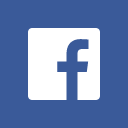
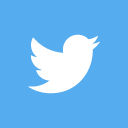
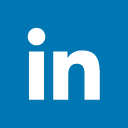
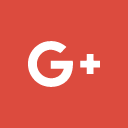
Abstract
The use of mobile phones is increasing rapidly with varying traffic demand. This drastic increase in mobile traffic is due to video streaming, mobile TV, video conferencing etc. 4G network is unable to full fill this increasing demand of bandwidth, broader coverage and lower latency. Due to this ever increasing demand, next generation of mobile networks which uses SDN, NFV, cloud approach in mobile networks came into picture. This new generation could become more efficient if various forecasting approaches are used at the centralized controller. Various algorithms for forecasting 4G data already exists using predictable behavior of mobile data traffic. Work has already been done to deploy 5G which uses SDMN paradigm. Thereafter comes the need for some forecasting technique at SDMN controller, which could predict traffic and make decision according to that prediction at centralized controller. This paper presents existing work on traffic forecast, SDMN, traffic forecasting without SDMN and with SDMN. It also discusses data analysis for dataset collection on which traffic forecasting algorithms could be tested.
Key-Words / Index Term
Software Defined Network, Network Function Virtualization, Software Defined Mobile Network, Traffic Forecasting, Real time data
References
[1] T. Cisco, Cisco Visual Networking Index: Global Mobile Data Traffic Forecast Update 2014–2019 White Paper, vol. 2011, no. 4. 2011.
[2] T. Chen, V. T. T. Technical, and N. Nikaein, “Towards Software Defined 5G Radio Access Networks,” no. March 2016, pp. 1–7, 2018.
[3] D. Karvounas, “5G on the Horizon,” no. sEptEmbEr, pp. 1–8, 2013.
[4] Jie Yang, Weicheng Li, Yuanyuan Qiao and Zubair Md. Fadlullah, Nei Kato, “Characterizing and Modeling of Large-Scale Traffic in Mobile Network,” in 2015 IEEE Wireless Communications and Networking Conference (WCNC), LA USA, New Orleans, 2015, pp. 801-806.
[5] Z. Liang and Y. Wakahara, “City traffic prediction based on real-time traffic information for intelligent transport systems,” in ITS Telecommunications (ITST), 2013 13th International Conference on IEEE, 2013, pp. 378–383.
[6] Cisco Visual Networking Index, “Global Mobile Data Traffic Forecast Update 2014-2019 White Paper”,2014.
[7] Jun Zhao, “Research on Prediction of Traffic Congestion State,” MATEC Web of Conferences, 2015,vol. 22.
[8] Shiliang Sun, Changshui Zhang, and Guoqiang Yu. A bayesian network approach to traffic flow forecasting.Intelligent Transportation Systems, IEEE Transactions on, 7(1):124 –132, march 2006.
[9] R. Chrobok, J. Wahle, and M. Schreckenberg. Traffic forecast using simulations of large scale networks. In Intelligent Transportation Systems, 2001. Proceedings. 2001 IEEE, pages 434 –439, 2001.
[10] Hussein Dia. An object-oriented neural network approach to short-term traffic forecasting. European Journal of Operational Research, 131(2):253 – 261, 2001.
[11] Feng Jin and Shiliang Sun. Neural network multitask learning for traffic flow forecasting. In Neural Networks,2008. IJCNN 2008. (IEEE World Congress on Computational Intelligence). IEEE International Joint Conference on, pages 1897 –1901, june 2008.
[12] Daniel B. Fambro Sangsoo Lee. Application of subset autoregressive integrated moving average model for short-term freeway traffic volume forecasting. Transportation Research Record: Journal of the Transportation Research Board, pages 179–188, 2007.
[13] Goodwin, P. 2010. The Holt-Winters Approach to Exponential Smoothing: 50 Years Old and Going Strong. Foresight 19:30-33
[14] S.C. Chang, R.S. Kim, S.J. Kim, and M.H. Ahn. Traffic-flow forecasting using a 3-stage model. In Intelligent Vehicles Symposium, 2000. IV 2000. Proceedings of the IEEE, pages 451 –456, 2000.
[15] M. Joshi and T. H. Hadi, “A Review of Network Traffic Analysis and Prediction Techniques,” p. 23, 2015.
[16] Ali Yadavar Nikravesh Samuel A. Ajila Chung-Horng Lung, Wayne Ding, “Mobile Network Traffic Prediction Using MLP, MLPWD, and SVM,” in 2016 IEEE International Congress on Big Data, 2016, pp. 402-409.
[17] R. Alvizu, S. Troia, G. Maier, and A. Pattavina, “Matheuristic With Machine-Learning-Based Prediction for Software-Defined Mobile Metro-Core Networks,” J. Opt. Commun. Netw., vol. 9, no. 9, p. D19, 2017.
[18] Z. Cao, S. Member, S. S. Panwar, M. Kodialam, and T. V Lakshman, “Networking and Cloud Computing,” vol. 25, no. 3, pp. 1–14, 2017.
[19] R. Alvizu, X. Zhao, G. Maier, Y. Xu, and A. Pattavina, “Energy aware optimization of mobile metro-core network under predictable aggregated traffic patterns,” 2016 IEEE Int. Conf. Commun. ICC 2016, 2016.
[20] R. Alvizu, X. Zhao, G. Maier, Y. Xu, and A. Pattavina, “Energy efficient dynamic optical routing for mobile metro-core networks under tidal traffic patterns,” J. Light. Technol., vol. 35, no. 2, pp. 325–333, 2017.
[21] I. Workshop, A. I. Multi, A. Intelligence, F. Chen, X. Zheng, C. Science, N. Computing, I. I. Processing, F. Online, D. Part, L. Notes, and C. Science, “Machine ¬ Learning Based Routing Pre ¬ plan for SDN,” pp. 1–17, 2018.
[22] Z. Zhong, N. Hua, H. Liu, Y. Li, and X. Zheng, “Considerations of effective tidal traffic dispatching in software-defined metro IP over optical networks,” 2015 Opto-Electronics Commun. Conf. OECC 2015, 2015.