Attribute Selection for the Early Diagnosis of Alzheimer`s Disease from Magnetic Resonance Images
C.S. Sandeep1 , A. Sukesh Kumar2
Section:Research Paper, Product Type: Journal Paper
Volume-6 ,
Issue-6 , Page no. 1321-1326, Jun-2018
CrossRef-DOI: https://doi.org/10.26438/ijcse/v6i6.13211326
Online published on Jun 30, 2018
Copyright © C.S. Sandeep, A. Sukesh Kumar . This is an open access article distributed under the Creative Commons Attribution License, which permits unrestricted use, distribution, and reproduction in any medium, provided the original work is properly cited.
View this paper at Google Scholar | DPI Digital Library
How to Cite this Paper
- IEEE Citation
- MLA Citation
- APA Citation
- BibTex Citation
- RIS Citation
IEEE Style Citation: C.S. Sandeep, A. Sukesh Kumar, “Attribute Selection for the Early Diagnosis of Alzheimer`s Disease from Magnetic Resonance Images,” International Journal of Computer Sciences and Engineering, Vol.6, Issue.6, pp.1321-1326, 2018.
MLA Style Citation: C.S. Sandeep, A. Sukesh Kumar "Attribute Selection for the Early Diagnosis of Alzheimer`s Disease from Magnetic Resonance Images." International Journal of Computer Sciences and Engineering 6.6 (2018): 1321-1326.
APA Style Citation: C.S. Sandeep, A. Sukesh Kumar, (2018). Attribute Selection for the Early Diagnosis of Alzheimer`s Disease from Magnetic Resonance Images. International Journal of Computer Sciences and Engineering, 6(6), 1321-1326.
BibTex Style Citation:
@article{Sandeep_2018,
author = {C.S. Sandeep, A. Sukesh Kumar},
title = {Attribute Selection for the Early Diagnosis of Alzheimer`s Disease from Magnetic Resonance Images},
journal = {International Journal of Computer Sciences and Engineering},
issue_date = {6 2018},
volume = {6},
Issue = {6},
month = {6},
year = {2018},
issn = {2347-2693},
pages = {1321-1326},
url = {https://www.ijcseonline.org/full_paper_view.php?paper_id=2347},
doi = {https://doi.org/10.26438/ijcse/v6i6.13211326}
publisher = {IJCSE, Indore, INDIA},
}
RIS Style Citation:
TY - JOUR
DO = {https://doi.org/10.26438/ijcse/v6i6.13211326}
UR - https://www.ijcseonline.org/full_paper_view.php?paper_id=2347
TI - Attribute Selection for the Early Diagnosis of Alzheimer`s Disease from Magnetic Resonance Images
T2 - International Journal of Computer Sciences and Engineering
AU - C.S. Sandeep, A. Sukesh Kumar
PY - 2018
DA - 2018/06/30
PB - IJCSE, Indore, INDIA
SP - 1321-1326
IS - 6
VL - 6
SN - 2347-2693
ER -
![]() |
![]() |
![]() |
556 | 353 downloads | 283 downloads |
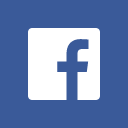
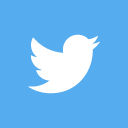
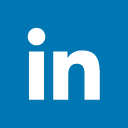
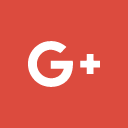
Abstract
Alzheimer`s disease (AD), also known as Senile Dementia of the Alzheimer Type (SDAT) or simply Alzheimer’s is the most common form of dementia. The AD is a slowly progressive disease of the brain that is characterized by impairment of memory and eventually by disturbances in reasoning, planning, language, and perception. Many scientists believe that Alzheimer`s disease results from an increase in the production or accumulation of a specific protein called beta-amyloid protein in the brain that leads to nerve cell death. Conventional clinical decision-making systems are more manual in nature and ultimate conclusion in terms of exact diagnosis is remote. In this case, the employment of advanced Biomedical Engineering Technology will definitely helpful for making a diagnosis. Profiling of human body parameter using computers can be utilized for the early diagnosis of Alzheimer’s disease. There are a lot of tests and imaging modalities to be performed for an effective diagnosis of the disease. In this paper, we have focused on MRI imaging for making an expert system for the diagnosis of the AD. For this purpose, we have used Discrete Wavelet Transform for the segmentation of MRI images. After segmentation, some of the attributes extracted using histogram, gradient, SURF, and Gabor has been done. Finally, we have selected some attributes based on the criteria of early diagnosis through MRI brain images.
Key-Words / Index Term
Alzheimer’s Disease, MRI, Discrete Wavelet Transform, histogram, gradient, SURF, Gabor
References
[1] Sandeep C S, Sukesh Kumar A, “A Review on the Early Prediction of Alzheimer’s Disease (AD) through Different Tests, Techniques and Databases”, AMSE JOURNALS Series: Modelling C, Vol. 76, Issue.1; pp.1-22, 2015.
[2] Harvey, R.A., P.C. Champe, B.D. Fisher, Lippincott’s Illustrated Reviews: Microbiology. 2nd Edn., Lippincott Williams and Wilkins, pp.432, 2006.
[3] Sandeep C S, Sukesh Kumar A, Susanth M J, “The Online Datasets Used to Classify the Different Stages for the Early Prediction of Alzheimer’s Disease (AD) “,International Journal of Engineering and Advanced Technology, Vol.6, Issue.4, pp.38-45, 2017.
[4] Cummings, J.L., H.V. Vinters, G.M. Cole and Z.S. Khachaturian, “Alzheimer’s disease: etiologies, pathophysiology, cognitive reserve and treatment opportunities”, Neurology, Vol.51. pp.2-17, 1998.
[5] Sandeep C S, Sukesh Kumar A, Susanth M J "The Early Diagnosis of Alzheimer Disease (AD) Using CAMD, TREAD and NAAC Databases" International Journal for Science and Advance Research In Technology, Vol.3, Issue.3, pp.366-371, 2017.
[6] Yaari, R. and J. Corey-Bloom, “Alzheimer’s disease: Pathology and pathophysiology”, Semin Neurol. Vol.27, pp.32-41, 2007.
[7] Larson EB, Wang L, Bowen JD, et al. “Exercise is associated with reduced risk for incident dementia among persons 65 years of age and older”, Ann Intern Med., Vol.144, pp.73-81, 2006.
[8] Mayeux R. “Epidemiology of neurodegeneration”, Annu Rev Neurosci., Vol.26, pp.81-104, 2003.
[9] Sandeep C S, Sukesh Kumar A ,”A Psychometric Assessment Method for the Early Prediction of Alzheimer’s disease”, International Journal of Scientific & Engineering Research, Vol.8, Issue.3, 2017
[10] Harvey RJ, Skelton-Robinson M, Rossor MN. “The prevalence and causes of dementia in people under the age of 65 years”, J Neurol Neurosurg Psychiatry, Vol.74, pp.1206-9, 2003.
[11] Chu LW, Tam S, Wong RL, et al. “Bioavailable testosterone predicts a lower risk of Alzheimer’s disease in older men”, J Alzheimers Dis; 21:1335-45, 2010.
[12] Sandeep C S, Sukesh Kumar A, Susanth M J,” Cognitive Examination for the Early Diagnosis of Alzheimer’s Disease”, IEEE International Conference on Trends in Electronics and Informatics, 2017
[13] K.-S. Cheng, J.-S. Lin, and C.-W. Mao, “Techniques and comparative analysis of neural network systems and fuzzy systems in medical image segmentation,” Fuzzy Theor. Syst. Tech. Appl., Vol.3, pp.973–1008, 1999.
[14] J. Jiang, P. Trundle, and J. Ren, “Medical image analysis with artificial neural networks,” Comput.Med. Imag. Graph., Vol. 34, Issue.8, pp. 617–631, 2010.
[15] Sandeep C S, Sukesh Kumar A, K Mahadevan and Manoj P, “Dimensionality Reduction of Optical Coherence Tomography Images for the Early Diagnosis of Alzheimer’s Disease”, American Journal of Electrical and Electronic Engineering, Vol.5, Issue.2, pp.58-63, 2017.
[16] Sandeep CS, Kumar SA, Mahadevan K, Manoj P, “Analysis of MRI and OCT Images for the Early Diagnosis of Alzheimer’s Disease Using Wavelet Networks”, AMSE journal on Lectures on Modelling and Simulation, pp.31-40, 2018
[17] Sandeep C.S, Sukesh Kumar.A, K. Mahadevan, Manoj P,” Classification of OCT Images for the Early Diagnosis of Alzheimer’s Disease”, IEEE International Conference on Intelligent Computing and Control (I2C2), 2017.
[18] Q. Zhang and A. Benveniste, “Wavelet networks,” IEEE Trans. Neural Netw., Vol.3, Issue.6, pp. 889–898, 1992.
[19] R. M. Balabin,R. Z. Safieva, and E. I. Lomakina,“Wavelet neural network (WNN) approach for calibration model building based on gasoline near infrared (NIR) spectra,” J. Chemometr. Intell. Lab. Syst., Vol.93, Issue.1, pp.58–62, 2008.
[20] Sandeep C.S, Sukesh Kumar.A, K. Mahadevan, Manoj P,” Early Prognosis of Alzheimer’s Disease Using Images from Fundus Camera”, IEEE International Conference on Electrical, Instrumentation and Communication Engineering (ICEICE), 2017.
[21] Y. C. Pati and P. S. Krishnaprasad, “Analysis and synthesis of feedforward neural networks using discrete affine wavelet tranformations,” IEEE Trans. Neur. Netw., Vol.4, Issue.1, pp.73–85, 1992.
[22] H. H. Szu, B. A. Telfer, and S. L. Kadambe, “Neural network adaptive wavelets for signal representation and classification,” Opt. Eng., Vol.31, Issue.9, pp.1907–1916, 1992.
[23] H. Zhang, B. Zhang, W. Huang, and Q. Tian, “Gabor wavelet associative memory for face recognition,” IEEE Trans. Neural Netw., Vol.16, Issue.1, pp. 275–278, 2005.
[24] O. Jemai, M. Zaied, C. B. Amar, and M. A. Alimi, “Pyramidal hybrid approach: Wavelet network with OLS algorithm-based image classification,” Int. J. Wavel. Multir. Inf. Process., Vol.9, Issue.1, pp.111–130, 2011.
[25] R. Galvao, V. M. Becerra, and M. F. Calado, “Linear–wavelet networks,” Int. J. Appl. Math. Comput. Sci., Vol.14, Issue.2, pp. 221–232, 2004.
[26] S. A. Billings and H. L. Wei, “A new class of wavelet networks for nonlinear system identification,” IEEE Trans. Neural Netw., Vol.16, Issue.4, pp. 862–874, 2005.
[27] Y. Oussar and G. Dreyfus, “Initialization by selection for wavelet network training,” Neurocomputing, Vol.34, Issue.1, pp.131–143, 2000.
[28] R. Baron and B. Girau, “Parameterized normalization: Application to wavelet networks,” in Proc. IEEE Int. Conf. Neural Netw., Vol.2, pp.1433–1437, 1998.
[29] Michael Freeman, “The Digital SLR Handbook. Ilex.” ,2005.
[30] Korn, Theresa M.; Korn, Granino Arthur, “Mathematical Handbook for Scientists and Engineers: Definitions, Theorems, and Formulas for Reference and Review”, Dover Publications. pp. 157–160, 2000.
[31] Herbert Bay, Andreas Ess, Tinne Tuytelaars, Luc Van Gool "SURF: Speeded Up Robust Features", Computer Vision and Image Understanding (CVIU), Vol. 110, Issue.3, pp.346–359, 2008.
[32] Jones, J.P.; Palmer, L.A., "An evaluation of the two-dimensional gabor filter model of simple receptive fields in cat striate cortex", J. Neurophysiol. Vol.58, Issue.6, pp.1233–1258, 1987.