ST Segment Analysis for Early Detection of Myocardial Infarction
Nang Anija Manlong1 , Jagdeep Rahul2 , Marpe Sora3
Section:Research Paper, Product Type: Journal Paper
Volume-6 ,
Issue-6 , Page no. 1500-1504, Jun-2018
CrossRef-DOI: https://doi.org/10.26438/ijcse/v6i6.15001504
Online published on Jun 30, 2018
Copyright © Nang Anija Manlong, Jagdeep Rahul, Marpe Sora . This is an open access article distributed under the Creative Commons Attribution License, which permits unrestricted use, distribution, and reproduction in any medium, provided the original work is properly cited.
View this paper at Google Scholar | DPI Digital Library
How to Cite this Paper
- IEEE Citation
- MLA Citation
- APA Citation
- BibTex Citation
- RIS Citation
IEEE Style Citation: Nang Anija Manlong, Jagdeep Rahul, Marpe Sora, “ST Segment Analysis for Early Detection of Myocardial Infarction,” International Journal of Computer Sciences and Engineering, Vol.6, Issue.6, pp.1500-1504, 2018.
MLA Style Citation: Nang Anija Manlong, Jagdeep Rahul, Marpe Sora "ST Segment Analysis for Early Detection of Myocardial Infarction." International Journal of Computer Sciences and Engineering 6.6 (2018): 1500-1504.
APA Style Citation: Nang Anija Manlong, Jagdeep Rahul, Marpe Sora, (2018). ST Segment Analysis for Early Detection of Myocardial Infarction. International Journal of Computer Sciences and Engineering, 6(6), 1500-1504.
BibTex Style Citation:
@article{Manlong_2018,
author = { Nang Anija Manlong, Jagdeep Rahul, Marpe Sora},
title = {ST Segment Analysis for Early Detection of Myocardial Infarction},
journal = {International Journal of Computer Sciences and Engineering},
issue_date = {6 2018},
volume = {6},
Issue = {6},
month = {6},
year = {2018},
issn = {2347-2693},
pages = {1500-1504},
url = {https://www.ijcseonline.org/full_paper_view.php?paper_id=2374},
doi = {https://doi.org/10.26438/ijcse/v6i6.15001504}
publisher = {IJCSE, Indore, INDIA},
}
RIS Style Citation:
TY - JOUR
DO = {https://doi.org/10.26438/ijcse/v6i6.15001504}
UR - https://www.ijcseonline.org/full_paper_view.php?paper_id=2374
TI - ST Segment Analysis for Early Detection of Myocardial Infarction
T2 - International Journal of Computer Sciences and Engineering
AU - Nang Anija Manlong, Jagdeep Rahul, Marpe Sora
PY - 2018
DA - 2018/06/30
PB - IJCSE, Indore, INDIA
SP - 1500-1504
IS - 6
VL - 6
SN - 2347-2693
ER -
![]() |
![]() |
![]() |
961 | 425 downloads | 277 downloads |
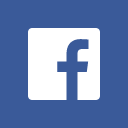
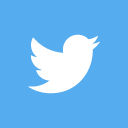
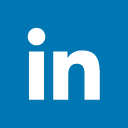
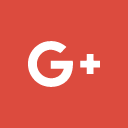
Abstract
Myocardial infarction is one of the most serious and prevailing heart disease faced in today’s world, occurs when blood supply stops to a certain artery. Early and accurate detection of myocardial infarction reduces the mortality rate of heart attack. In this paper, we proposed an algorithm for early detection of myocardial infarction based on analysis of ST segment in electrocardiogram (ECG). This algorithm consists of following steps: loading of a database from physionet, preprocessing of a signal, detection of QRS complex, P, T wave, ST segment and other related parameters. European ST-T database was used for evaluation of an algorithm for detection of ST segment.
Key-Words / Index Term
Electrocardiogram (ECG), myocardial infarction (MI), ST segment, QRS complex, European ST-T database
References
[1] Reddy, K.S., Yusuf, S.: Emerging epidemic of cardiovascular diseases in developing countries. Circulation 97(6), pp. 596-601, 1998.
[2] W.H.O.: World health statistics 2015: part ii: global health indicators. Technical report, World Health Organization (2015).
[3] Kumari Nirmala, R.M.Singh, Silpi Gupta,”Analysis of Heart related Issues using Comprehensive Approaches: A Review”, International Journal of Computer Science & Engineering,Vol3(3), pp.184-187,March 2015
[4] Duck Hee Lee, Jun Woo Park, Jeasoon Choi, Ahmed Rabbi and Reza Fazel-Rezai, “Automatic Detection of Electrocardiogram ST Segment: application in Ischemic Disease Diagnosis”, International Journal of Advanced Computer Science and Applications, Vol.4, No.2, 2014.
[5] Jayachandran, E.S., et al.: Analysis of myocardial infarction using discrete wavelet transform. J. Med. Syst. 34(6), pp. 985–992, 2010
[6] Banerjee, S., Mitra, M.: Application of cross wavelet transform for ECG pattern analysis and classification. IEEE Trans. Instrum. Meas. 63(2), pp. 326–333,2014
[7] Acharya, U.R., Fujita, H., Sudarshan, V.K., Oh, S.L., Adam, M., Koh, J.E., Tan, J.H., Ghista, D.N., Martis, R.J., Chua, C.K., et al.: Automated detection and localization of myocardial infarction using electrocardiogram: A comparative study of different leads. Knowl. Based Syst. 99, pp. 146–156, 2016
[8] Arif, M., Malagore, I.A., Afsar, F.A.: Detection and localization of myocardial infarction using k-nearest neighbor classifier. J. Med. Syst. 36(1), pp.279–289 2012
[9] Mitra, S., Mitra, M., Chaudhuri, B.B.: A rough-set-based inference engine for ECG classification. IEEE Trans. Instrum. Meas. 55(6), pp. 2198–2206, 2006
[10] Papaloukas, C., Fotiadis, D.I., Likas, A., Michalis, L.K.: An ischemia detection method based on artificial neural networks. Artif. Intell. Med. 24(2), pp. 167–178 2002.
[11] Safdarian, N., Dabanloo, N.J., Attarodi, G.: A new pattern recognition method for detection and localization of myocardial infarction using t-wave integral and total integral as extracted features from one cycle of ECG signal. J. Biomed. Sci. Eng. 7(10), pp.818–824,2014
[12] Zheng, H., Wang, H., Nugent, C., Finlay, D.: Supervised classification models to detect the presence of old myocardial infarction in body surface potential maps. In: Computers in Cardiology, pp. 265–268. IEEE, Valencia, Spain 2006
[13] “The European ST-T Database”, online at http://www.physionet.org/physiobank/database/edb/.
[14] J. Tompkins, A real-time QRS detection algorithm, IEEE Trans. Biomed. Eng. BME-32 (3), pp.230–236,1985
[15] Rangayyan R M. Biomedical Signal Analysis, 2nd edition.NewYork Wiley-IEEE Press;2015
[16] Rachid Haddadi, Elhassane Abdelmounim, Mustapha Elhanine, Abdelaziz Belaguid, “ST Segment Analysis Using Wavelet Transform”, International Journal of Computer Science and Network Security, Vol.17 No.9,2017