Improved cancer detection in mammogram images using automated Deep Learning Technique
P.Kaur 1 , G. Singh2 , P. Kaur3
Section:Research Paper, Product Type: Journal Paper
Volume-6 ,
Issue-6 , Page no. 1528-1539, Jun-2018
CrossRef-DOI: https://doi.org/10.26438/ijcse/v6i6.15281539
Online published on Jun 30, 2018
Copyright © P.Kaur, G. Singh, P. Kaur . This is an open access article distributed under the Creative Commons Attribution License, which permits unrestricted use, distribution, and reproduction in any medium, provided the original work is properly cited.
View this paper at Google Scholar | DPI Digital Library
How to Cite this Paper
- IEEE Citation
- MLA Citation
- APA Citation
- BibTex Citation
- RIS Citation
IEEE Style Citation: P.Kaur, G. Singh, P. Kaur, “Improved cancer detection in mammogram images using automated Deep Learning Technique,” International Journal of Computer Sciences and Engineering, Vol.6, Issue.6, pp.1528-1539, 2018.
MLA Style Citation: P.Kaur, G. Singh, P. Kaur "Improved cancer detection in mammogram images using automated Deep Learning Technique." International Journal of Computer Sciences and Engineering 6.6 (2018): 1528-1539.
APA Style Citation: P.Kaur, G. Singh, P. Kaur, (2018). Improved cancer detection in mammogram images using automated Deep Learning Technique. International Journal of Computer Sciences and Engineering, 6(6), 1528-1539.
BibTex Style Citation:
@article{Singh_2018,
author = {P.Kaur, G. Singh, P. Kaur},
title = {Improved cancer detection in mammogram images using automated Deep Learning Technique},
journal = {International Journal of Computer Sciences and Engineering},
issue_date = {6 2018},
volume = {6},
Issue = {6},
month = {6},
year = {2018},
issn = {2347-2693},
pages = {1528-1539},
url = {https://www.ijcseonline.org/full_paper_view.php?paper_id=2379},
doi = {https://doi.org/10.26438/ijcse/v6i6.15281539}
publisher = {IJCSE, Indore, INDIA},
}
RIS Style Citation:
TY - JOUR
DO = {https://doi.org/10.26438/ijcse/v6i6.15281539}
UR - https://www.ijcseonline.org/full_paper_view.php?paper_id=2379
TI - Improved cancer detection in mammogram images using automated Deep Learning Technique
T2 - International Journal of Computer Sciences and Engineering
AU - P.Kaur, G. Singh, P. Kaur
PY - 2018
DA - 2018/06/30
PB - IJCSE, Indore, INDIA
SP - 1528-1539
IS - 6
VL - 6
SN - 2347-2693
ER -
![]() |
![]() |
![]() |
588 | 323 downloads | 225 downloads |
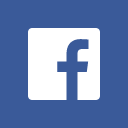
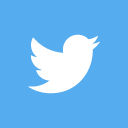
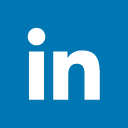
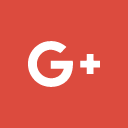
Abstract
Mammography is an exceptionally normal screening apparatus for diagnosing breast growth at beginning time as compare to other screening techniques to reduce female death rate. The techniques and algorithms that were extensively used are convolution neural networks, artificial neural networks, support vector machines, and so on. A comparison pertaining to the supremacy of deep learning techniques over existing machine learning techniques is also stated in terms of data requirements, learning, etc which is the need of the hour as the medical database is ever increasing phenomena demanding faster results. Though these studies are vast, this spectrum of research requires more rigorous investigation in terms of classification with minimal errors. In this paper, a proposed Deep Learning (DL) system is connected on large dataset to assess the prediction on the breast disease mammogram images as compare to state-of-art classification strategy. Despite the fact that this automation is known for its robustness still its execution relies on two key focuses that are: Clustering and Classification. The DL system result shows better qualitative result as compared to Multilayer Perceptron (MLP) method. The best precision of 86% for the given dataset is accomplished through proposed method when compared with different classifiers in terms of accuracy.
Key-Words / Index Term
Breast Cancer, Ultrasound, Mammography, Computer Aided Diagnosis(CAD) , Convolution Neural Network (CNN), Multi-Layer Perceptron(MLP), Machine learning techniques, Accuracy
References
[1]. Jemal, R. Siegel , E. Ward , Y. Hao, J. Xu , T. Murray , M. J. Thun, “Cancer statistics”, CA Cancer Journal Clinics, Vol. 58, Issue. 2, pp.71–96, 2008.
[2]. Secretan, C. Scoccianti, D. Loomis, L. Benbrahim, V. Bouvard, F. Bianchini, K. Straif, “Breast-cancer screening–view point of the IARC working group”, New England Journal Medical, Vol. 372, Issue 24, pp. 2353–2358, 2015.
[3]. M. Giger and A. Pritzker, “Medical imaging and computers in the diagnosis of breast cancer”, International Society for Optics and Photonics, pp. 918-908, 2014.
[4]. G. Carneiro, Y. Zheng, F. Xing and L. Yang, “Review of Deep Learning Methods in Mammography, Cardiovascular, and Microscopy Image Analysis”, Springer Advances in Computer Vision and Pattern Recognition, pp. 11-32, DOI 10.1007/978-3-319-42999-1_2, 2017.
[5]. www.radiologyinfo.org/en/info.cfm?pg=mammo.
[6]. E. Kozegar, M. Soryani, H. Behnam, M. Salamati and T. Tan , “Breast cancer detection in automated 3D breast ultrasound using iso-contours and cascaded RUSBoosts”, Ultrasonics, Vol. 79,pp. 68–80, 2017.
[7]. Q. Huang, Y. Luo and Q. Zhang, “Breast ultrasound image segmentation: a survey”, International Journal of CARS, DOI 10.1007/s11548-016-1513-1, 2017.
[8]. M. Sahar, H. Nugroho and I. Ardiyanto, “Automated Detection of Breast Cancer Lesions Using Adaptive Thresholding and Morphological Operation”, IEEE International Conference on Information Technology Systems and Innovation, 2016.
[9]. “www.peipa.essex.ac.uk/pix/mias”.
[10]. “www.archive.ics.uci.edu/ml/machine-learning- databases/breast-cancer-wisconsin”.
[11]. J. Shan, S. K. Alam, B. Garra, Y. Zhang, and T. Ahmed, “Computer-Aided Diagnosis for Breast Ultrasound Using Computerized BI-RADS Features and Machine Learning Methods”, Ultrasound in medicine & biology, Vol. 42, Issue 4, pp. 980-988, 2016.
[12]. N. Abdelwahed, M. Eltoukhy and M. Wahed, “Computer Aided System for Breast Cancer Diagnosis in Ultrasound Images”, 2015.
[13]. S. K. Alam, E. J. Feleppa, M. Rondeau, A. Kalisz, and B. S. Garra, “Computer-aided diagnosis of solid breast lesions using an ultrasonic multi-feature analysis procedure”, Bangladesh Journal of Medical Physics, Vol. 4, Issue 1, pp.1-10, 2013.
[14]. Amin, M. Khalid, A. Shahin and Y. Guo, "A novel breast tumor classification algorithm using neutrosophic score features", Measurement, Vol. 81,pp. 210-220, 2016.
[15]. Breast Cancer Facts & Figures 2015-2016.
[16]. “Breast Imaging Reporting and Data System (BI-RADS)”, Ultrasound, American College of Radiology, 2003.
[17]. H.D. Cheng, J. Shan, W. Ju, Y. Guo and L. Zhang, “Automated breast cancer detection and classification using ultrasound images: A survey”, Pattern Recognition, Vol. 43, Issue 1, pp.299-317, 2010.
[18]. G. Walter, A. Pereira, A. Fernando and C. Infantosi, "Analysis of co-occurrence texture statistics as a function of gray-level quantization for classifying breast ultrasound", IEEE transactions on medical imaging, Vol. 31, Issue 10, pp. 1889-1899, 2012.
[19]. Liu, H. D. Cheng, J. Huang, J. Tian, X. Tang, and J. Liu, “Fully automatic and segmentation-robust classification of breast tumors based on local texture analysis of ultrasound images”, Pattern Recognition, Vol. 43, Issue 1, pp.280-298, 2010.
[20]. Y. Liu, H.D. Cheng, J.H. Huang, Y.T. Zhang, X.L. Tang, J.W. Tian and Y. Wang, “Computer aided diagnosis system for breast cancer based on color Doppler flow imaging” , Journal of medical systems, Vol. 36, Issue 6, pp.3975-3982, 2012.
[21]. W. Moon, C. Lo, N. Cho, M. Chang, S. Huang , H. Chen and F. Chang, “Computer-Aided Diagnosis of breast masses using quantified BI-RADS findings”, Computer methods and programs in biomedicine, Vol. 111, Issue 1, pp.84-92, 2013.
[22]. S. Muthuselvan and K.S. Sundaram, “Prediction of breast cancer using classification rule mining techniques in blood test datasets”, IEEE International Conference on Information Communication and Embedded Systems (ICICES), pp. 1-5, 2016.
[23]. C.M. Sehgal, T.W. Cary, A. Cwanger, B.J. Levenback, and S.S. Venkatesh, “Combined Naive Bayes and logistic regression for quantitative breast sonography”, IEEE International Ultrasonics Symposium, pp. 1686-1689, 2012.
[24]. L. Sellami, O.B. Sassi and A.B. Hamida, “Breast Cancer Ultrasound Images Sequence Exploration Using BI-RADS Features Extraction: Towards an Advanced Clinical Aided Tool for Precise Lesion Characterization”, IEEE transactions on nano bioscience, Vol. 14, Issue 7, pp.740-745, 2015.
[25]. J. Shan, S.K. Alam, B. Garra, Y. Zhang, and T. Ahmed, “Computer-Aided Diagnosis for Breast Ultrasound Using Computerized BI-RADS Features and Machine Learning Methods”, Ultrasound in medicine & biology, Vol. 42, Issue 4, pp.980-988, 2016.
[26]. X. Shi, H.D. Cheng, L. Hu, W. Ju and J. Tian, “Detection and classification of masses in breast ultrasound images”, Digital Signal Processing, Vol. 20, Issue 3, pp.824-836, 2010.
[27]. E.A. Sickles, C.J. Dorsi, L.W. Bassett, C.M. Appleton, W.A. Berg, and E.S. Burnside, “ACR BI-RADS (Atlas Breast Imaging Reporting and Data System)”, American College of Radiology, 2013.
[28]. S.S. Venkatesh, B.J. Levenback, L.R. Sultan, G. Bouzghar and C.M. Sehgal, “Going beyond a First Reader: A Machine Learning Methodology for Optimizing Cost and Performance in Breast Ultrasound Diagnosis”, Ultrasound in medicine & biology, Vol. 41, Issue 12, pp.3148-3162, 2015.
[29]. P. Wahdan, A. Saad and A. Shoukry, “Automated Breast Tumour Detection in Ultrasound Images Using Support Vector Machine and Ensemble Classification”, Journal of TBA, Vol. 3, 2016.
[30]. F.S. Zakeri, H. Behnam and N. Ahmadinejad, “Classification of benign and malignant breast masses based on shape and texture features in sonography images”, Journal of medical systems, Vol. 36, Issue 3, pp.1621-1627, 2012.
[31]. J.C.M Zelst, T. Tan, B. Platel, M. Jong, A. Steenbakkers, M. Mourits, A. Grivegnee, C. Borelli, N. Karssemeije and R.M. Mann, “Improved cancer detection in automated breast ultrasound by radiologists using Computer Aided Detection”, European Journal of Radiology, Vol. 89,pp. 54–59, 2017.
[32]. Z. Liu, J. Zhang and L. Liu, “Upright orientation of 3D shapes with Convolutional Networks”, Graphical Models, Vol. 0, pp. 1–8, 2016.
[33]. Sreekumari, S. Shriram and V. Vaidya, “Breast Lesion Detection and Characterization with 3D features”, Global Research, Bengaluru, India.
[34]. J. Zhou, Z. Yang, Z. Weiwei, Z. Jingwen, H. Na, D. Yijie and Y. WANG , “Breast Lesions Evaluated by Color-Coded Acoustic Radiation Force Impulse (ARFI) Imaging”, Ultrasound in Medical & Biology, pp. 1–9, 2016.
[35]. K. Xu, G. Kim, Q. Huang and E. Kalogerakis, “Data-Driven Shape Analysis and Processing”, Computer Graphics forum, Vol. 36, 2017.
[36]. VIDYA AND S. MATHEW, “AN ACCURATE METHOD OF BREAST CANCER DETECTION FROM ULTRA SOUND IMAGES USING PROBABILISTIC FUZZY CLUSTERING ALGORITHM”, IEEE INTERNATIONAL CONFERENCE ON COMMUNICATION SYSTEMS AND NETWORKS, 2016.
[37]. J. Krzysztof, S. Wolfson, Y. Shen, G. Kim, L. Moy, Cho, “High-Resolution Breast Cancer Screening with Multi-View Deep Convolutional Neural Networks”, IEEE.
[38]. T. SenthilSelvi1 and R. Parimala, “Improving Clustering Accuracy using Feature Extraction Method”, International Journal of Scientific Research in Computer Science and Engineering, Vol. 6, Issue. 2, pp.15-19, 2018.
[39]. Sherlin and D. Murugan, “A Case Study on Brain Tumor Segmentation Using Content based Imaging”, International Journal of Scientific Research in Network Security and Communication, Vol. 6, Issue. 3, pp. 1-5, 2018.