A Robust Hybrid Human Activity Recognition System using Lbptop and Body Joint Features with Majority Voting for High Accuracy
Halakundi Chidananda1 , T Hanumantha Reddy2
Section:Research Paper, Product Type: Journal Paper
Volume-6 ,
Issue-7 , Page no. 367-374, Jul-2018
CrossRef-DOI: https://doi.org/10.26438/ijcse/v6i7.367374
Online published on Jul 31, 2018
Copyright © Halakundi Chidananda, T Hanumantha Reddy . This is an open access article distributed under the Creative Commons Attribution License, which permits unrestricted use, distribution, and reproduction in any medium, provided the original work is properly cited.
View this paper at Google Scholar | DPI Digital Library
How to Cite this Paper
- IEEE Citation
- MLA Citation
- APA Citation
- BibTex Citation
- RIS Citation
IEEE Citation
IEEE Style Citation: Halakundi Chidananda, T Hanumantha Reddy, “A Robust Hybrid Human Activity Recognition System using Lbptop and Body Joint Features with Majority Voting for High Accuracy,” International Journal of Computer Sciences and Engineering, Vol.6, Issue.7, pp.367-374, 2018.
MLA Citation
MLA Style Citation: Halakundi Chidananda, T Hanumantha Reddy "A Robust Hybrid Human Activity Recognition System using Lbptop and Body Joint Features with Majority Voting for High Accuracy." International Journal of Computer Sciences and Engineering 6.7 (2018): 367-374.
APA Citation
APA Style Citation: Halakundi Chidananda, T Hanumantha Reddy, (2018). A Robust Hybrid Human Activity Recognition System using Lbptop and Body Joint Features with Majority Voting for High Accuracy. International Journal of Computer Sciences and Engineering, 6(7), 367-374.
BibTex Citation
BibTex Style Citation:
@article{Chidananda_2018,
author = {Halakundi Chidananda, T Hanumantha Reddy},
title = {A Robust Hybrid Human Activity Recognition System using Lbptop and Body Joint Features with Majority Voting for High Accuracy},
journal = {International Journal of Computer Sciences and Engineering},
issue_date = {7 2018},
volume = {6},
Issue = {7},
month = {7},
year = {2018},
issn = {2347-2693},
pages = {367-374},
url = {https://www.ijcseonline.org/full_paper_view.php?paper_id=2443},
doi = {https://doi.org/10.26438/ijcse/v6i7.367374}
publisher = {IJCSE, Indore, INDIA},
}
RIS Citation
RIS Style Citation:
TY - JOUR
DO = {https://doi.org/10.26438/ijcse/v6i7.367374}
UR - https://www.ijcseonline.org/full_paper_view.php?paper_id=2443
TI - A Robust Hybrid Human Activity Recognition System using Lbptop and Body Joint Features with Majority Voting for High Accuracy
T2 - International Journal of Computer Sciences and Engineering
AU - Halakundi Chidananda, T Hanumantha Reddy
PY - 2018
DA - 2018/07/31
PB - IJCSE, Indore, INDIA
SP - 367-374
IS - 7
VL - 6
SN - 2347-2693
ER -
![]() |
![]() |
![]() |
461 | 418 downloads | 286 downloads |
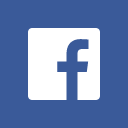
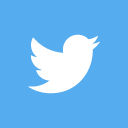
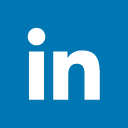
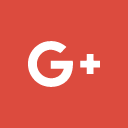
Abstract
Human activity recognition has seen an enormous success in the last decade performing a dominant part in the field of ubiquitous computing. This rising demand can be attributed to several real life applications fundamentally dealing with human-centric applications like healthcare and eldercare systems. Many research experiments with data mining and machine learning procedures have been experiencing precisely to recognize human activities for healthcare systems. This work proposes a hybrid method to recognize the patient actions under care using a simple camera instead of multiple expensive sensors using machine learning with LBPTOP algorithm and body joint features with majority voting framework for real time monitoring applications with greater efficiency of recognition. This work uses different classifiers to achieve the experimental results approximately above 90% which clearly shows a remarkable recognition achievement compared to the other activity recognition techniques.
Key-Words / Index Term
Machine Learning, Human activity, Body joint features, Real time, LBP-TOP, Classifiers
References
[1]. Katz S, Ford AB, Moskowitz RW, Jackson BA, Jaffe MW: Studies of illness in the aged. The index of ADL: A Standardized Measure of Biological and Psychological Function: JAMA 1963; 185:914–919.
[2]. Chan M, Esteve D, Escriba C, Campo E: A review of smart homes-Present state and future challenges: Comput Methods Programs Biomed 2008;91:51–81.
[3]. Tarricone R, Tsouros AD: The solid facts: home care in Europe: World Health Organization (WHO), Geneva, 2008.
[4]. Lai JHK, Yik FWH: Perceived importance of the quality of the indoor environment in commercial buildings: Indoor Built Environ 2007;16(4):311–321.
[5]. Aylward C, Harris RS, Harrison PTC: Analysis of topics and trends in indoor environment research in Europe: Studies in Indoor Monitoring: Indoor Built Environ 2005;14(3-4):197–200.
[6]. A. Yilmaz and M. Shah, “Actions sketch: a novel action representation,” in Proc. Comput. Vis. Pattern Recognit., 2005, vol. 1, pp. 984–989.
[7]. L. Gorelick, M. Blank, E. Shechtman, M. Irani, and R. Basri, “Actions as space-time shapes,” IEEE Trans. Pattern Anal. Mach. Intell., vol. 29, no. 12, pp. 2247–2253, Dec. 2007.
[8]. D.-Y. Chen, H.-Y. M. Liao, and S.-W. Shih, “Humnn action recognition using 2-D spatio-temporal templates,” in Proc. Int. Conf. MultimediaExpo, 2007, pp. 667–670.
[9]. A. Bobick and J. Davis, “The recognition of human movement using temporal templates,” IEEE Trans. Pattern Anal. Mach. Intell., vol. 23, no. 3, pp. 257–267, Mar. 2001.
[10]. M. Hu, “Visual pattern recognition by moment invariants,” IRE Trans. Inf. Theory, vol. 8, no. 2, pp. 179–187, Feb. 1962.
[11]. H. Meng, N. Pears, and C. Bailey, “A human action recognition system for embedded computer vision application,” in Proc. Comput. Vis. Pattern Recognit., 2007, pp. 1–8.
[12]. H. Fujiyoshi and A. Lipton, “Real-time human motion analysis by image skeletonization,” in Proc. 4th IEEE Workshop Appl. Comput. Vis., 1998, pp. 15–21.
[13]. M. Blank, L. Gorelick, E. Shechtman, M. Irani, and R. Basri, “Actions as space-time shapes,” in Proc. Int. Conf. Comput. Vis., 2005, pp. 1395–1402.
[14]. L.Wang and D. Suter, “Learning and matching of dynamic shape manifolds for human action recognition,” IEEE Trans. Image Process., vol. 16, no. 6, pp. 1646–1661, Jun. 2007.
[15]. Vapnik, V. Statistical learning theory (Adaptive and Learning Systems for Signal Processing, Communications and Control Series). John Wiley & Sons, New York (1998).
[16]. Larose, D. T. KNN. In: Discovering Knowledge in Data: An Introduction to Data Mining, 1st Ed., pp. 90-106. New Jersey; Wiley Interscience (2004).
[17]. Han, J., Kamber, M. Data Mining: Concepts and Techniques. Morgan Kaufmann (2006).
[18]. Larose, D. T. Decision Trees. In: Discovering Knowledge in Data: An Introduction to Data Mining, 1st Ed., pp. 108-126. New Jersey; Wiley Interscience (2004).
[19]. Lepetit V, Fua P (2006) Keypoint recognition using randomized trees. IEEE Trans Pattern Anal Mach Intell28:1465–1479
[20]. Baum E, Eagon J (1967) An inequality with applications to statistical estimation for probabilistic functions of Markov processes and to a model for ecology. Bull Am Math Soc 73:360–363
[21]. Lawrence R, Rabiner A (1989) Tutorial on hidden Markov models and selected applications in speech recognition. Proc IEEE 77(2):257–286
[22]. Nishimura Hand TsutsumiM (2001) Off-line hand-written character recognition using integrated1DHMMsbased on feature extraction filters. Sixth International Conference on Document Analysis and Recognition, p 417–421
[23]. Uddin, M.Z. (2017) Human activity recognition using segmented body part and body joint features with hidden Markov models. Multimed Tools Appl 76:13585–13614 DOI 10.1007/s11042-016-3742-2.