Automatic Human Age Estimation System for Face Images
Mittala Thulasi1 , Chandra Mohan Reddy Sivappagari2
Section:Research Paper, Product Type: Journal Paper
Volume-6 ,
Issue-7 , Page no. 550-555, Jul-2018
CrossRef-DOI: https://doi.org/10.26438/ijcse/v6i7.550555
Online published on Jul 31, 2018
Copyright © Mittala Thulasi, Chandra Mohan Reddy Sivappagari . This is an open access article distributed under the Creative Commons Attribution License, which permits unrestricted use, distribution, and reproduction in any medium, provided the original work is properly cited.
View this paper at Google Scholar | DPI Digital Library
How to Cite this Paper
- IEEE Citation
- MLA Citation
- APA Citation
- BibTex Citation
- RIS Citation
IEEE Citation
IEEE Style Citation: Mittala Thulasi, Chandra Mohan Reddy Sivappagari, “Automatic Human Age Estimation System for Face Images,” International Journal of Computer Sciences and Engineering, Vol.6, Issue.7, pp.550-555, 2018.
MLA Citation
MLA Style Citation: Mittala Thulasi, Chandra Mohan Reddy Sivappagari "Automatic Human Age Estimation System for Face Images." International Journal of Computer Sciences and Engineering 6.7 (2018): 550-555.
APA Citation
APA Style Citation: Mittala Thulasi, Chandra Mohan Reddy Sivappagari, (2018). Automatic Human Age Estimation System for Face Images. International Journal of Computer Sciences and Engineering, 6(7), 550-555.
BibTex Citation
BibTex Style Citation:
@article{Thulasi_2018,
author = {Mittala Thulasi, Chandra Mohan Reddy Sivappagari},
title = {Automatic Human Age Estimation System for Face Images},
journal = {International Journal of Computer Sciences and Engineering},
issue_date = {7 2018},
volume = {6},
Issue = {7},
month = {7},
year = {2018},
issn = {2347-2693},
pages = {550-555},
url = {https://www.ijcseonline.org/full_paper_view.php?paper_id=2472},
doi = {https://doi.org/10.26438/ijcse/v6i7.550555}
publisher = {IJCSE, Indore, INDIA},
}
RIS Citation
RIS Style Citation:
TY - JOUR
DO = {https://doi.org/10.26438/ijcse/v6i7.550555}
UR - https://www.ijcseonline.org/full_paper_view.php?paper_id=2472
TI - Automatic Human Age Estimation System for Face Images
T2 - International Journal of Computer Sciences and Engineering
AU - Mittala Thulasi, Chandra Mohan Reddy Sivappagari
PY - 2018
DA - 2018/07/31
PB - IJCSE, Indore, INDIA
SP - 550-555
IS - 7
VL - 6
SN - 2347-2693
ER -
![]() |
![]() |
![]() |
856 | 278 downloads | 223 downloads |
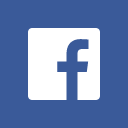
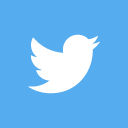
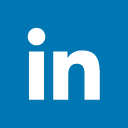
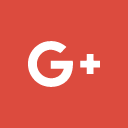
Abstract
recognition of patterns. For a facial image in order to identify the accurate age huge face data is supposed to be attached to the age labels in order to make the algorithms more effective. On the utilization of training data which is labelled weakly or is either unlabelled this imposes a constraint. For example, in the social networks huge number of human photos is there. No age label is offered by these images but the age difference can easily be derived for the pair of an image when a person is same. The age accuracy estimation can be brought about by the suggested scheme based on novel learning to take benefit of data which is labelled weakly with the help of CNN which is an abbreviation of Convolution neural network. In case of repair of an image, the divergence suggested by Kullback-Leibler is applied and this is done to embed the information which is different on the basis of age. The loss of entropy and cross entropy is applied adaptively on all the images in order to get a single and unified peak value. To drive the neural network so as to understand the gradual ages from the information of age differentiation the combination of these losses are designed. With one hundred thousand images of faces which are attached along with their data taken we can also contribute to a data set. With the personal identity and time stamp each image is labelled. It is shown by the two aging faces data bases on the experimentation analysis that for this kind of learning system there are a lot of advantages and one can also achieve state to art performance.
Key-Words / Index Term
Age estimation, age difference, convolution neural networks, K-L divergence distance
References
Dyer,Locally adjusted robust registration for human age estimation.In Proceedings of the 2008 IEEE work shop on Applications of Computer vision,pages 1-6,2008
[2]. Jiwen Lu, Venice Erin Liong, and Jie Zhou. Cost-fragile neighborhood parallel incorporate learning for facial age estimation. Picture Processing, IEEE Exchanges on, 24(12):5356– 5368,2015
[3]. Y.Sun,X.Wang,X.Tang,Deepconvolutionalnetworkcascadeforfacialpointde-tection,in:CVPR,IEEE,2013,pp.3476–3483.
[4]. Y.Taigman,M.Yang,M.Ranzato,L.Wolf,Deepface:closingthegaptohumanlevelperformanceinfaceverification,in:ConferenceonComputerVisionandPatternRecognition(CVPR),2014,IEEE,2014,pp.1701–1708.
[5]. M.Yang,S.Zhu,F.Lv,K.Yu,Correspondencedrivenadaptationforhumanprofilerecognition,in:ConferenceonComputerVisionandPatternRecognition(CVPR),2011,IEEE,2011,pp.505–512.
[6]. Xin Geng, Chao Yin, and Zhi-Hua Zhou. Facial age estimation by picking up from stamp scatterings. Case Analysis and Machine Knowledge, IEEE Transactions on, 35(10):2401– 2412, 2013