Bayesian Classification for Social Media Text
Amit Kumar Mittal1 , Shivangi Mittal2 , Digendra Singh Rathore3
Section:Research Paper, Product Type: Journal Paper
Volume-6 ,
Issue-7 , Page no. 641-646, Jul-2018
CrossRef-DOI: https://doi.org/10.26438/ijcse/v6i7.641646
Online published on Jul 31, 2018
Copyright © Amit Kumar Mittal, Shivangi Mittal, Digendra Singh Rathore . This is an open access article distributed under the Creative Commons Attribution License, which permits unrestricted use, distribution, and reproduction in any medium, provided the original work is properly cited.
View this paper at Google Scholar | DPI Digital Library
How to Cite this Paper
- IEEE Citation
- MLA Citation
- APA Citation
- BibTex Citation
- RIS Citation
IEEE Style Citation: Amit Kumar Mittal, Shivangi Mittal, Digendra Singh Rathore, “Bayesian Classification for Social Media Text,” International Journal of Computer Sciences and Engineering, Vol.6, Issue.7, pp.641-646, 2018.
MLA Style Citation: Amit Kumar Mittal, Shivangi Mittal, Digendra Singh Rathore "Bayesian Classification for Social Media Text." International Journal of Computer Sciences and Engineering 6.7 (2018): 641-646.
APA Style Citation: Amit Kumar Mittal, Shivangi Mittal, Digendra Singh Rathore, (2018). Bayesian Classification for Social Media Text. International Journal of Computer Sciences and Engineering, 6(7), 641-646.
BibTex Style Citation:
@article{Mittal_2018,
author = {Amit Kumar Mittal, Shivangi Mittal, Digendra Singh Rathore},
title = {Bayesian Classification for Social Media Text},
journal = {International Journal of Computer Sciences and Engineering},
issue_date = {7 2018},
volume = {6},
Issue = {7},
month = {7},
year = {2018},
issn = {2347-2693},
pages = {641-646},
url = {https://www.ijcseonline.org/full_paper_view.php?paper_id=2486},
doi = {https://doi.org/10.26438/ijcse/v6i7.641646}
publisher = {IJCSE, Indore, INDIA},
}
RIS Style Citation:
TY - JOUR
DO = {https://doi.org/10.26438/ijcse/v6i7.641646}
UR - https://www.ijcseonline.org/full_paper_view.php?paper_id=2486
TI - Bayesian Classification for Social Media Text
T2 - International Journal of Computer Sciences and Engineering
AU - Amit Kumar Mittal, Shivangi Mittal, Digendra Singh Rathore
PY - 2018
DA - 2018/07/31
PB - IJCSE, Indore, INDIA
SP - 641-646
IS - 7
VL - 6
SN - 2347-2693
ER -
![]() |
![]() |
![]() |
410 | 290 downloads | 220 downloads |
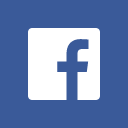
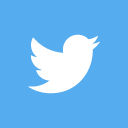
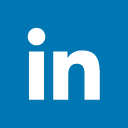
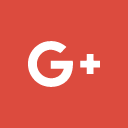
Abstract
The data mining is a technique by which the computational algorithms are trained for finding the similar patterns from the huge or raw set of data. The training of the algorithms is performed on the patterns which are required to extract from the data. The training of the algorithms can be supervised or unsupervised. The main advantage of the supervised learning algorithms, these are efficient, accurate and effective as compared to the unsupervised learning approaches. In this presented work the text classification is the key area of study. The text classification techniques are used to classify according to their categories or the domain specific knowledge. Thus the text classification has rich applications. Among a number of applications of the text classification the social media based text classification and the sentiment analysis of the user’s text is comparatively new work in the text mining. In this presented work the social media based text is mined for discovering the user sentiments or moods which are expressed using the twitter based text communication. Therefore big data analytics are used to performing the text classification. First the twitter data is hosted on the HDFS directory and then the features are computed using the Map-reduce technique. The collected features are then labelled using the NLP tool which is used to discover the part of speech composition of the text sentences. After parsing the text using NLP tool the Bayesian classifier is implemented for classification of the social media text. The implementation of the proposed technique is performed using the JAVA technology. After implementation the performance of the proposed system is evaluated in terms of accuracy and the complexity. Both the performance parameters show the proposed sentiment analysis technique is effective and accurate for classifying the social media text for orientation discovery of user text.
Key-Words / Index Term
classification; sentiment analysis; supervised learning text orientation; text mining
References
Data Gathering From Live Social Media Streams”, ICMR’14, April 01-04, 2014, Glasgow, United Kingdom. Copyright 2014 ACM 978-1-4503-2782-4/14/04
[2] A Comparison of Several Approaches to Missing Attribute Values in Data Mining, Jerzy W. Grzymala-Busse and Ming Hu, Springer-Verlag Berlin Heidelberg 2001, pp. 378−385,
[3] Ritika, “Research on Data Mining Classification”, International Journal of Advanced Research in Computer Science and Software Engineering, Volume 4, Issue 4, April 2014
[4] Abbas Jafari, S.S.Patil, “Use of Data Mining Technique To Design A Driver Assistance System”, Proceedings of 7th IRF International Conference, 27th April-2014, Pune, India, ISBN: 978-93-84209-09-4
[5] A.K. Jain, M.N. Murthy, P. J. Flynn, “Data Clustering: A Review”, © 2000 ACM 0360-0300/99/0900–0001
[6] Khaled Hammouda ,”A Comparative Study of Data Clustering Techniques”, Department of Systems Design Engineering, University of Waterloo, Waterloo, Ontario, Canada N2L 3G1
[7] Hans-Peter Kriegel, Peer Kröger, Jörg Sander, Arthur Zimek, "Density-based Clustering", WIREs Data Mining and Knowledge Discovery 1 (3): 231–240. doi:10.1002/widm.30
[8] B. V. Rama Krishna, B. Sushma, “Novel Approach to Museums Development & Emergence of Text Mining”, ISSN 2249-6343, International Journal of Computer Technology and Electronics Engineering (IJCTEE), Volume 2, Issue 2
[9] H. P. Luhn, “A Business Intelligence System”, Volume 2, Number 4, Page 314 (1958), Nontopical Issue, IBM Research Journals
[10] Andreas Hotho, Andreas Nurnberger, Gerhard Paaß, Fraunhofer AiS, “A Brief Survey of Text Mining”, Knowledge Discovery Group Sankt Augustin, May 13, 2005s
[11] Hien Nguyen, Eugene Santos, and Jacob Russell, “Evaluation of the Impact of User-Cognitive Styles on the Assessment of Text Summarization”, IEEE Transactions on Systems, Man, and Cybernetics—Part A: Systems and Humans, Vol. 41, No. 6, November 2011
[12] Umajancy. S, Dr. Antony Selvadoss Thanamani, “An Analysis on Text Mining –Text Retrieval and Text Extraction”, International Journal of Advanced Research in Computer and Communication Engineering, Vol. 2, Issue 8, August 2013
[13] Miloš Radovanović, Mirjana Ivanović, “Text Mining: Approaches And Applications”, Abstract Methods and Applications in Computer Science (no. 144017A), Novi Sad, Serbia, Vol. 38, No. 3, 2008, 227-234
[14] Siva S. Sivatha Sindhu, S. Geetha, A. Kannan, “Decision tree based light weight intrusion detection using a wrapper approach”, Expert Systems with Applications, 2011 Elsevier Ltd. All rights reserved.
[15] M. Jayakameswaraiah and S. Ramakrishna, “Implementation of an Improved ID3 Decision Tree Algorithm in Data Mining System”, International Journal of Computer Science and Engineering, Volume-2, Issue-3
[16] Biswajeet Pradhan, “A comparative study on the predictive ability of the decision tree, support vector machine and neuro-fuzzy models in landslide susceptibility mapping using GIS”, Computers & Geosciences, & 2012 Elsevier Ltd. All rights reserved.
[17] Neha Patel, Divakar Singh, “An Algorithm to Construct Decision Tree for Machine Learning based on Similarity Factor”, International Journal of Computer Applications (0975 – 8887) Volume 111 – No 10, February 2015
[18] T. Ramani, M. Ramzan Begam, “Survey: A Techniques implemented on Opinion Mining”, International Journal of Computer Science & Engineering Technology (IJCSET), Vol. 5 No. 10 Oct 2014
[19] Walaa Medhat, Ahmed Hassan, Hoda Korashy, “Sentiment analysis algorithms and applications: A survey”, Ain Shams Engineering Journal, (2014) 5, 1093–1113
[20] Tirivangani BHT Magadza, Addlight Mukwazvure, K.P Supreethi, “Exploring Sentiment Classification Techniques in News Articles”, IJITKM Volume 8 • Number 1 June-Dec 2014 pp. 55-58 (ISSN 0973-4414)
[21] Alya Al Nasseri, Allan Tucker, Sergio de Cesare, “Quantifying StockTwits semantic terms’ trading behavior in financial markets: An effective application of decision tree algorithms”, © 2015 The Authors. Published by Elsevier Ltd.
[22] Rodrigo Moraes, João Francisco Valiati, Wilson P. Gavião Neto, “Document-level sentiment classification: An empirical comparison between SVM and ANN”, Expert Systems with Applications, 2012 Elsevier Ltd. All rights reserved.
[23] Suge Wang, Deyu Li, Lidong Zhao, Jiahao Zhang, “Sample cutting method for imbalanced text sentiment classification based on BRC”, Knowledge-Based Systems, 2012 Elsevier B.V. All rights reserved..