Melanoma Detection Using Modified Extended LBP
Ritesh Maurya1
Section:Research Paper, Product Type: Journal Paper
Volume-6 ,
Issue-7 , Page no. 698-703, Jul-2018
CrossRef-DOI: https://doi.org/10.26438/ijcse/v6i7.698703
Online published on Jul 31, 2018
Copyright © Ritesh Maurya . This is an open access article distributed under the Creative Commons Attribution License, which permits unrestricted use, distribution, and reproduction in any medium, provided the original work is properly cited.
View this paper at Google Scholar | DPI Digital Library
How to Cite this Paper
- IEEE Citation
- MLA Citation
- APA Citation
- BibTex Citation
- RIS Citation
IEEE Style Citation: Ritesh Maurya, “Melanoma Detection Using Modified Extended LBP,” International Journal of Computer Sciences and Engineering, Vol.6, Issue.7, pp.698-703, 2018.
MLA Style Citation: Ritesh Maurya "Melanoma Detection Using Modified Extended LBP." International Journal of Computer Sciences and Engineering 6.7 (2018): 698-703.
APA Style Citation: Ritesh Maurya, (2018). Melanoma Detection Using Modified Extended LBP. International Journal of Computer Sciences and Engineering, 6(7), 698-703.
BibTex Style Citation:
@article{Maurya_2018,
author = {Ritesh Maurya},
title = {Melanoma Detection Using Modified Extended LBP},
journal = {International Journal of Computer Sciences and Engineering},
issue_date = {7 2018},
volume = {6},
Issue = {7},
month = {7},
year = {2018},
issn = {2347-2693},
pages = {698-703},
url = {https://www.ijcseonline.org/full_paper_view.php?paper_id=2495},
doi = {https://doi.org/10.26438/ijcse/v6i7.698703}
publisher = {IJCSE, Indore, INDIA},
}
RIS Style Citation:
TY - JOUR
DO = {https://doi.org/10.26438/ijcse/v6i7.698703}
UR - https://www.ijcseonline.org/full_paper_view.php?paper_id=2495
TI - Melanoma Detection Using Modified Extended LBP
T2 - International Journal of Computer Sciences and Engineering
AU - Ritesh Maurya
PY - 2018
DA - 2018/07/31
PB - IJCSE, Indore, INDIA
SP - 698-703
IS - 7
VL - 6
SN - 2347-2693
ER -
![]() |
![]() |
![]() |
432 | 268 downloads | 130 downloads |
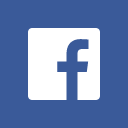
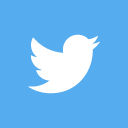
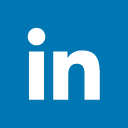
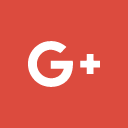
Abstract
Detecting skin cancer at an early age is very crucial for differentiating malignant melanoma from benign one. We presented a novel approach for automatic detection of skin cancer based on modified extended LBP feature. Extended LBP is a generalized form of LBP and we have proposed some modification in its functioning in order to make it more robust. Gabor filter bank is used to segment the lesion area based on the frequency and orientation pattern of the lesion in input image. We have trained and tested our proposed methodology on Support Vector Machine. We have used our own self-created database which consists of 225 images captured from different internet resources. The proposed framework is able to achieve sensitivity, specificity and overall accuracy of about 92.72%, 94.5% and 93.6% respectively.
Key-Words / Index Term
Extended Local Binary Pattern, Modified Extended LBP Gabor filter, Support Vector Machine, Image Segmentation
References
[1] Fredrik Georgsson and Tor-Björn Holmström ,Master Thesis in Computing Science “A Survey and Evaluation of Features for Diagnosis of Malignant Melanoma” August 2005.
[2] Pehamberger H, Binder M, Steiner A, Wolff K. “In vivo epiluminescence microscopy: improvement of early diagnosis of melanoma.” , J Invest Dermatol , 1993,vol.100,pp-356S–62S.
[3] Bafounta ML, Beauchet A, Aegerter P, Saiag P. “Is dermoscopy (epiluminescence microscopy) useful for the diagnosis of melanoma? Results of a meta-analysis using techniques adapted to the evaluation of diagnostic tests”. Arch Dermatol 2001,vol. 137,pp. 1343–50.
[4] McGovern TW, Litaker MS. “Clinical predictors of malignant pigmented lesions“a comparison of the Glasgow seven-point checklist and the American Cancer Society’s ABCDs of pigmented lesions” J DermatolSurgOncol 1992,vol 18,pp22–26.
[5] H. Harms, H.M. Aus, M. Haucke, U. Gunzer, “Segmentation of stained blood cell images measured at high scanning density with high magnification and high numerical aperture optics, Cytometry” 1992;vol. 7;pp. 522-531
[6]W. Stolz, T. Vogth, M. Landthaler, S. Hempfer, P. Bingler, W. Abmayr, “Differentiation between maligant melanomas and benign melanocytic nevi by computerized DNA cytometry of imprint specimens”, J CutanPathol 1994, vol. 21;pp. 7-15
[7]A.R. Brown, “Combined immunecytochemical staining and image analysis for the study of lymphocyte specificity and function in situ” J Immunol Methods 1990; vol. 130:pp 410-414
[8].http://www.mathworks.com/
[9]Binder M, Steiner A, Schwarz M, Knollmayer S, Wolff K, Pehamberger H: “Application of an artificial neural network in epiluminescence microscopy pattern analysis of pigmented skin lesions:a pilot study.” Br J Dermatol1994, vol. 130:pp.460-465.
[10]Piccolo D, Ferrari A, Peris K, Diadone R, Ruggeri B, Chimenti S “Dermoscopic diagnosis by a trained clinician vs. a clinician with minimal dermoscopy training vs. computer-aided diagnosis of 341 pigmented skin lesions: a comparative study”. Br JDermatol2002, vo.147, pp.481-486
[11]Blum A, Luedtke H, Ellwanger U, Schwabe R, Rassner G, Garbe C: “Digital image analysis for diagnosis of cutaneous melanoma”.
[12]. Medical Image analysis Methods. Edited by Lena Costaridou,Taylor &Francis
[13] T. Ojala, M. Pietikäinen, T. Mäenpää, “Multiresolution gray-scale and rotation invariant texture classification with local binary patterns”, IEEE Trans. Pattern Anal. Mach. Intell., Vol.24 ,pp.971–987.
[14] Li Liu a, , Lingjun Zhao a, Yunli Long a, Gangyao Kuang a, Paul Fieguth b , “Extended Local Binary Patterns for Texture Classification”, Image and Vision Computing, pg 86-99
[15]. Erkol, B.; Moss, R.H.; Stanley, R.J.; Stoecker, W.V.; Hvatum, E. “Automatic lesion boundary detection in dermoscopy images using gradient vector flow snakes”. Skin Res. Technol., vol.11, pp.17–26. 2005
[16]. Rajab, M.I.; Woolfson, M.S.; Morgan, S.P. “Application of region-based segmentation and neural network edge detection to skin lesions.”, Comput. Med. Imaging Graph.vol. 28, pp 61–68,2004
[17]. Zhou, H.; Schaefer, G.; Sadka, A.H.; Celebi, M.E. “Anisotropic mean shift based fuzzy c-means segmentation of dermoscopy images. “IEEE J. Sel. Top. Signal Proc. 2009, vol. 3,pp. 26–34.
[18]. Schmid, P. Segmentation of digitized dermatoscopic images by two-dimensional color clustering. IEEE Trans.Med. Imaging, vol.18, pp.164–171. 1999
[19].Celebi, M.E.;Wen, Q.; Hwang, S.; Iyatomi, H.; Schaefer, G.” Lesion border detection in dermoscopy images using ensembles of thresholding methods”. Skin Res. Technol.,vol. 19, pp.e252–e258.
[20].Sadek, S.; Al-Hamadi, A.; Michaelis, B.; Sayed, U. Human action recognition: A novel scheme using fuzzy log-polar histogram and temporal self-similarity. EURASIP J. Adv. Signal Proc., vol.1,pp. 1–9. 2011
[21]. Sadek, S.; Al-Hamadi, A.; Michaelis, B.; Sayed, U. An SVM approach for activity recognition based on chord-length-function shape features. In Proceedings of the IEEE International Conference on Image
Processing (ICIP’12), Orlando, FL, USA; pp. 767–770, , 30 September–3 October 2012
[22].Vapnik, V.N. The Nature of Statistical Learning Theory; Springer: New York, NY, USA, 1995.
[23]. Vapnik, V.N. An overview of statistical learning theory. IEEE Trans. Neural Netw.,vol. 10,pp. 988–999.
[24]. Sadek, S.; Al-Hamadi, A.; Michaelis, B.; Sayed, U. Towards Robust Human Action Retrieval in Video. In Proceedings of the British Machine Vision Conference (BMVC’10), Aberystwyth, UK, 31 August–3 September 2010.
[25]. Sadek, S.; Al-Hamadi, A.; Michaelis, B.; Sayed, U. Human Activity Recognition: A Scheme Using Multiple Cues. In Advances in Visual Computing, Proceedings of the International Symposium on Visual Computing (ISVC’10), Las Vegas, NV, USA, 29 November–1 December 2010; Springer: Berlin/Heidelberg, Germany, 2010; Volume 6454, pp. 574–583.
[26]. Elgamal, M. Automatic Skin Cancer Images Classification. Int. J. Adv. Comput. Sci. Appl. 2013, 4, 1–8.
[27]. Sheha, M.A.; Mabrouk, M.S.; Sharawy, A. Automatic detection of melanoma skin cancer using texture analysis. Int. J. Comput. Appl.,vol. 42,pp. 22–26. 2012
[28]. M. Zortea, S. O. Skrovseth, and F. Godtliebsen, “Automatic learning of spatial patterns for diagnosis of skin lesions.,” Conference Proceedings of the International Conference of IEEE Engineering in Medicine and Biology Society, vol. 2010, pp. 5601-5604, 2010.