Segmentation and Classification of Histologocal Structures in H & E Stained Images
S.B.Pawar 1 , V.S.Gaikwad 2
Section:Research Paper, Product Type: Journal Paper
Volume-6 ,
Issue-7 , Page no. 869-873, Jul-2018
CrossRef-DOI: https://doi.org/10.26438/ijcse/v6i7.869873
Online published on Jul 31, 2018
Copyright © S.B.Pawar, V.S.Gaikwad . This is an open access article distributed under the Creative Commons Attribution License, which permits unrestricted use, distribution, and reproduction in any medium, provided the original work is properly cited.
View this paper at Google Scholar | DPI Digital Library
How to Cite this Paper
- IEEE Citation
- MLA Citation
- APA Citation
- BibTex Citation
- RIS Citation
IEEE Style Citation: S.B.Pawar, V.S.Gaikwad, “Segmentation and Classification of Histologocal Structures in H & E Stained Images,” International Journal of Computer Sciences and Engineering, Vol.6, Issue.7, pp.869-873, 2018.
MLA Style Citation: S.B.Pawar, V.S.Gaikwad "Segmentation and Classification of Histologocal Structures in H & E Stained Images." International Journal of Computer Sciences and Engineering 6.7 (2018): 869-873.
APA Style Citation: S.B.Pawar, V.S.Gaikwad, (2018). Segmentation and Classification of Histologocal Structures in H & E Stained Images. International Journal of Computer Sciences and Engineering, 6(7), 869-873.
BibTex Style Citation:
@article{_2018,
author = {S.B.Pawar, V.S.Gaikwad},
title = {Segmentation and Classification of Histologocal Structures in H & E Stained Images},
journal = {International Journal of Computer Sciences and Engineering},
issue_date = {7 2018},
volume = {6},
Issue = {7},
month = {7},
year = {2018},
issn = {2347-2693},
pages = {869-873},
url = {https://www.ijcseonline.org/full_paper_view.php?paper_id=2527},
doi = {https://doi.org/10.26438/ijcse/v6i7.869873}
publisher = {IJCSE, Indore, INDIA},
}
RIS Style Citation:
TY - JOUR
DO = {https://doi.org/10.26438/ijcse/v6i7.869873}
UR - https://www.ijcseonline.org/full_paper_view.php?paper_id=2527
TI - Segmentation and Classification of Histologocal Structures in H & E Stained Images
T2 - International Journal of Computer Sciences and Engineering
AU - S.B.Pawar, V.S.Gaikwad
PY - 2018
DA - 2018/07/31
PB - IJCSE, Indore, INDIA
SP - 869-873
IS - 7
VL - 6
SN - 2347-2693
ER -
![]() |
![]() |
![]() |
280 | 205 downloads | 217 downloads |
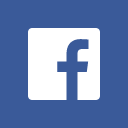
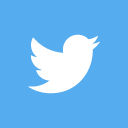
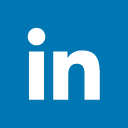
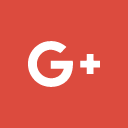
Abstract
Segmenting a broad class of histological structures is a prerequisite to determine the pathological basis of cancer, to clarify spatial interactions between histological structures in the tumor microenvironments, making precision medicine studies easy with deep molecular profiles, and provide an exploratory tool for pathologists. Histological structure determination helps elucidate spatial tumor biology. Role focuses on the segmentation of histological structures present in colored images with stains (H & E) of the breast tissue. Accurate segmentation of histological structures can help build a spatial interaction map to serve as an exploratory tool for pathologists. Breast cancer if detected early can be cured easily. Hence detection methods need to have more accurate diagnosis. Images obtained out of the scans done, processed to get the segments, which are then seen as clusters. These clusters are evaluated with classification techniques to reach the diagnosis result.
Key-Words / Index Term
histopathalogical image analysis, image segmentation, image statistics
References
[1] Luong Nguyen, “Spatial statistics for segmenting histological structures in HE stained tissue images", IEEE TMI, 2017.
[2] E. Bejnordi et al, “Automated detection of dcis in whole-slide he stained breast histopathology images", IEEE TMI, 2016.
[3] J. Vicory et al, “Appearance normalization of histology slides, Computerized Medical Imaging and Graphics",vol. 43, pp. 8998, 2015.
[4] X. Li and K. N. Plataniotis, “A complete color normalization approach to histopathology images using color cues computed from saturation weighted statistics"IEEE TBME, vol. 62, no. 7, pp.18621873, 2015.
[5] P. Isola et al, “Crisp boundary detection using pointwise mutual information,"ECCV 2014, 2014, pp. 799814.
[6] Vahadane et al, “Structure-preserving color normalization and sparse stain separation for histological images,"IEEE TMI, 2016.
[7] F. Liu and L. Yang, “A novel cell detection method using deep convolutional neural network and maximum-weight independent set,"MICCAI, pp. 349357, 2015.
[8] J. L. Fine, “21st century workow: A proposal,"Journal of Pathology Informatics, vol. 5, no. 1,p. 44, 2014.
[9] B.-R. Wei and R. M. Simpson, “Digital pathology and image analysis augment biospecimen annotation and biobank quality assurance harmonization,",Clinical biochemistry, vol. 47, no. 4, pp.274279, 2014
[10] M. T. McCann et al, “Images as occlusions of textures: a framework for segmentation,", Clinical biochemistry, vol. 47, no. 4, pp. 274279, 2014.
[11] N. M. Rajpoot, “HyMaP: A hybrid magnitude-phase approach to unsupervised segmentation of tumor areas in breast cancer histology images,",Journal of Pathology Informatics, vol.4,no.2,p.1,2013
[12] S. Tamilarasan, P.K. Sharma, “A Survey on Dynamic Resource Allocation in MIMO Heterogeneous Cognitive Radio Networks based on Priority Scheduling”, International Journal of Computer Sciences and Engineering, Vol.5, No.1, pp.53-59, 2017.