USING SOCIAL INTERACTIONS ON SOCIAL NETWORKS DETECTING USERS IN STRESS
K. R. Bhokare1 , N. M. More2
Section:Research Paper, Product Type: Journal Paper
Volume-6 ,
Issue-7 , Page no. 961-965, Jul-2018
CrossRef-DOI: https://doi.org/10.26438/ijcse/v6i7.961965
Online published on Jul 31, 2018
Copyright © K. R. Bhokare, N. M. More . This is an open access article distributed under the Creative Commons Attribution License, which permits unrestricted use, distribution, and reproduction in any medium, provided the original work is properly cited.
View this paper at Google Scholar | DPI Digital Library
How to Cite this Paper
- IEEE Citation
- MLA Citation
- APA Citation
- BibTex Citation
- RIS Citation
IEEE Style Citation: K. R. Bhokare, N. M. More, “USING SOCIAL INTERACTIONS ON SOCIAL NETWORKS DETECTING USERS IN STRESS,” International Journal of Computer Sciences and Engineering, Vol.6, Issue.7, pp.961-965, 2018.
MLA Style Citation: K. R. Bhokare, N. M. More "USING SOCIAL INTERACTIONS ON SOCIAL NETWORKS DETECTING USERS IN STRESS." International Journal of Computer Sciences and Engineering 6.7 (2018): 961-965.
APA Style Citation: K. R. Bhokare, N. M. More, (2018). USING SOCIAL INTERACTIONS ON SOCIAL NETWORKS DETECTING USERS IN STRESS. International Journal of Computer Sciences and Engineering, 6(7), 961-965.
BibTex Style Citation:
@article{Bhokare_2018,
author = {K. R. Bhokare, N. M. More},
title = {USING SOCIAL INTERACTIONS ON SOCIAL NETWORKS DETECTING USERS IN STRESS},
journal = {International Journal of Computer Sciences and Engineering},
issue_date = {7 2018},
volume = {6},
Issue = {7},
month = {7},
year = {2018},
issn = {2347-2693},
pages = {961-965},
url = {https://www.ijcseonline.org/full_paper_view.php?paper_id=2542},
doi = {https://doi.org/10.26438/ijcse/v6i7.961965}
publisher = {IJCSE, Indore, INDIA},
}
RIS Style Citation:
TY - JOUR
DO = {https://doi.org/10.26438/ijcse/v6i7.961965}
UR - https://www.ijcseonline.org/full_paper_view.php?paper_id=2542
TI - USING SOCIAL INTERACTIONS ON SOCIAL NETWORKS DETECTING USERS IN STRESS
T2 - International Journal of Computer Sciences and Engineering
AU - K. R. Bhokare, N. M. More
PY - 2018
DA - 2018/07/31
PB - IJCSE, Indore, INDIA
SP - 961-965
IS - 7
VL - 6
SN - 2347-2693
ER -
![]() |
![]() |
![]() |
391 | 311 downloads | 151 downloads |
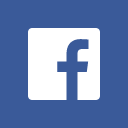
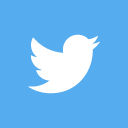
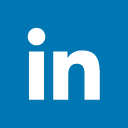
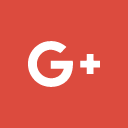
Abstract
Mental stress is becoming a threat to people’s health now a days. With the rapid pace of life, more and more people are feeling stressed. It is not easy to detect users stress in an early time to protect user. With the fame of web-based social networking, individuals are used it for sharing their day by day activities and interacting with friends, via web-based networking media stages, making it possible to use online social network data for stress detection. Facebook application contains different posts which shows different emotions. Conventional neural network(CNN) is used for topic extraction. Using Support Vector Method(SVM) we can classified users are in stress or not.After classification users are in stress or not ,k-nearest neighbours algorithm (KNN) is used for recommendation of hospital on a map.This system is proposed for users healthy mental state.
Key-Words / Index Term
Stress Detection, Factor Graph Model, Microblog, Social media, Healthcare, Social Interaction
References
[1] Yuan Zhang, Jie Tang, Jimeng Sun, Yiran Chen, and JinghaiRao.”Moodcast: Emotion prediction via dynamic continuous factor graph model” 2016 IEEE International Conference on Data Mining
[2] Liqiang Nie, Yi-Liang Zhao, Mohammad Akbari, JialieShen, and Tat-Seng Chua.”Bridging the vocabulary gap between health seekers and healthcare knowledge” 2013
[3] Frank R. Kschischang, Senior Member ”Factor Graphs and the Sum-Product Algorithm” ,IEEE TRANSACTIONS 2015
[4] Xiao jun Chang, Yi Yang1, Alexander G. Hauptmann, Eric P. Xing and Yao-Liang Yu “Semantic Concept Discovery for Large-Scale Zero-Shot Event Detection Proceedings” of the Twenty-Fourth International Joint Conference on Artificial Intelligence (IJCAI 2015)
[5] Jennifer Golbeck, Cristina Robles, Michon Edmondson, and Karen Turner.”Predicting personality from twitter”. In Passat/socialcom 2011, Privacy, Security, Risk and Trust, pages 149–156, 2011
[6] Sepandar D. Kamvar. “We feel fine and searching the emotional web”. In In Proceedings of WSDM, pages 117–126, 2011
[7] Dan C Ciresan, Ueli Meier, Jonathan Masci, Luca Maria Gambardella,furgenSchmidhuber.”Flexible,high performance convolutional neural networks for image classification”.In Proceedings of International Joint Conference on Artificial Intelligence, pages 1237–1242, 2011.
[8] Chi Wang, Jie Tang, Jimeng Sun, and Jiawei Han “Dynamic social influence analysis through time-dependent factor graphs”IEEE 2011
[9] AndreyBogomolov, Bruno Lepri, MichelaFerron, Fabio Pianesi, and Alex Pentland.”Daily stress recognition from mobile phone data, weather conditions and individual traits.” In ACM International Conference on Multimedia, pages 477–486, 2014.
[10] H. Lin, J. Jia, Q. Guo, Y. Xue, J. Huang, L. Cai, and L. Feng. “Psychological stress detection from cross-media microblog data using deep sparse neural network”.In proceedings of IEEE International Conference on Multimedia & Expo, 2014.
[11] Lexing Xie and Xuming He. “Picture tags and world knowledge: learning tag relations from visual semantic sources” 2013
[12] QuanGuo, JiaJia, GuangyaoShen, Lei Zhang, LianhongCai, and Zhang Yi. “Learning robust uniform features for cross-media social data by using cross autoencoders. “Knowledge Based System,102:64– 75, 2016