Review on Machine Learning Techniques for Big Data Management and Open Research Challenges
Gagandeep Kaur1 , Jasvir Singh2
Section:Review Paper, Product Type: Journal Paper
Volume-6 ,
Issue-7 , Page no. 1052-1055, Jul-2018
CrossRef-DOI: https://doi.org/10.26438/ijcse/v6i7.10521055
Online published on Jul 31, 2018
Copyright © Gagandeep Kaur, Jasvir Singh . This is an open access article distributed under the Creative Commons Attribution License, which permits unrestricted use, distribution, and reproduction in any medium, provided the original work is properly cited.
View this paper at Google Scholar | DPI Digital Library
How to Cite this Paper
- IEEE Citation
- MLA Citation
- APA Citation
- BibTex Citation
- RIS Citation
IEEE Style Citation: Gagandeep Kaur, Jasvir Singh, “Review on Machine Learning Techniques for Big Data Management and Open Research Challenges,” International Journal of Computer Sciences and Engineering, Vol.6, Issue.7, pp.1052-1055, 2018.
MLA Style Citation: Gagandeep Kaur, Jasvir Singh "Review on Machine Learning Techniques for Big Data Management and Open Research Challenges." International Journal of Computer Sciences and Engineering 6.7 (2018): 1052-1055.
APA Style Citation: Gagandeep Kaur, Jasvir Singh, (2018). Review on Machine Learning Techniques for Big Data Management and Open Research Challenges. International Journal of Computer Sciences and Engineering, 6(7), 1052-1055.
BibTex Style Citation:
@article{Kaur_2018,
author = {Gagandeep Kaur, Jasvir Singh},
title = {Review on Machine Learning Techniques for Big Data Management and Open Research Challenges},
journal = {International Journal of Computer Sciences and Engineering},
issue_date = {7 2018},
volume = {6},
Issue = {7},
month = {7},
year = {2018},
issn = {2347-2693},
pages = {1052-1055},
url = {https://www.ijcseonline.org/full_paper_view.php?paper_id=2559},
doi = {https://doi.org/10.26438/ijcse/v6i7.10521055}
publisher = {IJCSE, Indore, INDIA},
}
RIS Style Citation:
TY - JOUR
DO = {https://doi.org/10.26438/ijcse/v6i7.10521055}
UR - https://www.ijcseonline.org/full_paper_view.php?paper_id=2559
TI - Review on Machine Learning Techniques for Big Data Management and Open Research Challenges
T2 - International Journal of Computer Sciences and Engineering
AU - Gagandeep Kaur, Jasvir Singh
PY - 2018
DA - 2018/07/31
PB - IJCSE, Indore, INDIA
SP - 1052-1055
IS - 7
VL - 6
SN - 2347-2693
ER -
![]() |
![]() |
![]() |
364 | 378 downloads | 173 downloads |
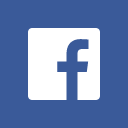
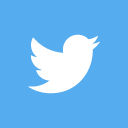
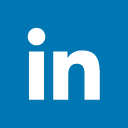
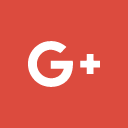
Abstract
In the recent years, the database on the cloud network is increased exponentially and this enormous volume of data is known as Big Data. The Big Data is described in five V’s known as volume, velocity, variety, Veracity, and Value. Hence, efficient algorithms and architectures are required to process and store the data. In this paper, a review study on machine learning techniques for database management is done. From the study, it is found that machine-learning algorithms provide efficient data processing and storage. In the last research issues are defined which helps the other author to contribute their work in this area.
Key-Words / Index Term
Big Data, Machine Learning, Internet of Things, Database Management
References
[1] Athmaja, S., Hanumanthappa, M. and Kavitha, V, “A survey of machine learning algorithms for big data analytics,” In Innovations in Information, Embedded and Communication Systems (ICIIECS), 2017 International Conference, pp. 1-4, 2017.
[2] Raghupathi, W. and Raghupathi, V., “Big data analytics in healthcare: promise and potential,” Health information science and systems, vol. 2, issue 1, p.3, 2014
[3] Suthaharan, S., “Big data classification: Problems and challenges in network intrusion prediction with machine learning,” ACM SIGMETRICS Performance Evaluation Review, vol. 41, pp.70-73, 2014
[4] Wei Tan, Brian Blake, Iman Saleh, and Schahram Dustar, “Social-Networks-Sourced Big Data Analytics,” IEEE Internet Computing, vol. 17, issue 5, pp.62-69, 2013.
[5] Edosio, U.Z., “Big data Analytics and its Application in E-commerce,” Proceedings E-Commerce Technologies. University of Bradford, 2014.
[6] Singh, S.P. and Jaiswal, U.C., “Machine Learning for Big Data: A New Perspective,” International Journal of Applied Engineering Research, vol. 13, issue 5, pp.2753-2762, 2018.
[7] Zhou, L., Pan, S., Wang, J. and Vasilakos, A.V., “Machine learning on big data: Opportunities and challenges,” Neurocomputing, vol. 237, pp.350-361, 2017.
[8] Swathi, R. and Seshadri, R., “Systematic survey on evolution of machine learning for big data,” In Intelligent Computing and Control Systems (ICICCS), 2017 International Conference on, pp. 204-209, 2017.
[9] Zhou, L., Pan, S., Wang, J. and Vasilakos, A.V., Machine learning on big data: Opportunities and challenges. Neurocomputing, vol. 237, pp.350-361, 2017.
[10] Varatharajan, R., Manogaran, G. and Priyan, M.K., “A big data classification approach using LDA with an enhanced SVM method for ECG signals in cloud computing,” Multimedia Tools and Applications, vol. 77, issue 8 , pp.10195-10215, 2018.
[11] Maillo, J., Ramírez, S., Triguero, I. and Herrera, F.,. “KNN-IS: An Iterative Spark-based design of the k-Nearest Neighbors classifier for big data,” Knowledge-Based Systems, vol. 117, pp.3-15, 2017.
[12] Chen, M., Hao, Y., Hwang, K., Wang, L. and Wang, L.,. “Disease prediction by machine learning over big data from healthcare communities,” IEEE Access, vol. 5, pp.8869-8879, 2017
[13] Sun, F., Huang, G.B., Wu, Q.J., Song, S. and Wunsch II, D.C.,. “Efficient and rapid machine learning algorithms for big data and dynamic varying systems,” IEEE Transactions on Systems, Man, and Cybernetics: Systems, vol. 47, issue 10, pp.2625-2626, 2017.
[14] Le, L.V., Sinh, D., Tung, L.P. and Lin, B.S.P., “A practical model for traffic forecasting based on big data, machine-learning, and network KPIs,” In Consumer Communications & Networking Conference (CCNC), 2018 15th IEEE Annual, pp. 1-4, 2018.
[15] Li, P., Chen, Z., Yang, L.T., Zhang, Q. and Deen, M.J., “Deep convolutional computation model for feature learning on big data in Internet of Things” IEEE Transactions on Industrial Informatics, vol. 14, issue 2, pp.790-798, 2018.
[16] Shen, Y., Han, T., Yang, Q., Yang, X., Wang, Y., Li, F. and Wen, H., “CS-CNN: Enabling Robust and Efficient Convolutional Neural Networks Inference for Internet-of-Things Applications,” IEEE Access, vol. 6, pp.13439-13448, 2018.
[17] Lakshmanaprabu, S.K., Shankar, K., Khanna, A., Gupta, D., Rodrigues, J.J., Pinheiro, P.R. and De Albuquerque, V.H.C., “Effective Features to Classify Big Data Using Social Internet of Things,” IEEE Access, vol. 6, pp.24196-24204, 2018.