Financial Market Predictions: Generative Vs Discriminative Methods
P. Misra1 , S. Chaurasia2
Section:Research Paper, Product Type: Journal Paper
Volume-6 ,
Issue-7 , Page no. 1373-1378, Jul-2018
CrossRef-DOI: https://doi.org/10.26438/ijcse/v6i7.13731378
Online published on Jul 31, 2018
Copyright © P. Misra, S. Chaurasia . This is an open access article distributed under the Creative Commons Attribution License, which permits unrestricted use, distribution, and reproduction in any medium, provided the original work is properly cited.
View this paper at Google Scholar | DPI Digital Library
How to Cite this Paper
- IEEE Citation
- MLA Citation
- APA Citation
- BibTex Citation
- RIS Citation
IEEE Style Citation: P. Misra, S. Chaurasia, “Financial Market Predictions: Generative Vs Discriminative Methods,” International Journal of Computer Sciences and Engineering, Vol.6, Issue.7, pp.1373-1378, 2018.
MLA Style Citation: P. Misra, S. Chaurasia "Financial Market Predictions: Generative Vs Discriminative Methods." International Journal of Computer Sciences and Engineering 6.7 (2018): 1373-1378.
APA Style Citation: P. Misra, S. Chaurasia, (2018). Financial Market Predictions: Generative Vs Discriminative Methods. International Journal of Computer Sciences and Engineering, 6(7), 1373-1378.
BibTex Style Citation:
@article{Misra_2018,
author = {P. Misra, S. Chaurasia},
title = {Financial Market Predictions: Generative Vs Discriminative Methods},
journal = {International Journal of Computer Sciences and Engineering},
issue_date = {7 2018},
volume = {6},
Issue = {7},
month = {7},
year = {2018},
issn = {2347-2693},
pages = {1373-1378},
url = {https://www.ijcseonline.org/full_paper_view.php?paper_id=2614},
doi = {https://doi.org/10.26438/ijcse/v6i7.13731378}
publisher = {IJCSE, Indore, INDIA},
}
RIS Style Citation:
TY - JOUR
DO = {https://doi.org/10.26438/ijcse/v6i7.13731378}
UR - https://www.ijcseonline.org/full_paper_view.php?paper_id=2614
TI - Financial Market Predictions: Generative Vs Discriminative Methods
T2 - International Journal of Computer Sciences and Engineering
AU - P. Misra, S. Chaurasia
PY - 2018
DA - 2018/07/31
PB - IJCSE, Indore, INDIA
SP - 1373-1378
IS - 7
VL - 6
SN - 2347-2693
ER -
![]() |
![]() |
![]() |
420 | 285 downloads | 240 downloads |
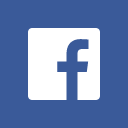
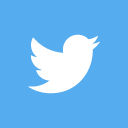
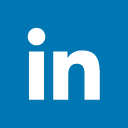
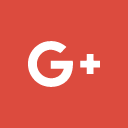
Abstract
Prediction of stock market is acclaimed by many as one of the most challenging areas for machine learning. The existence of quant industry that makes use of artificial intelligence based computational methods to predict the market provide enough evidence contrary to Efficient Market Hypothesis and Random Walk theory. Recent research on the financial market has focused on machine learning based approaches where instead of specifying the rules, learning algorithms are employed to make use of existing data. Financial markets provide one of the most organized data sets where data from each tick is recorded. Both generative and discriminative class of machine learning techniques have been explored in search of improved accuracy. Even with the abundance of structured financial data, complex, chaotic and nonlinear nature of the market that can ride on public emotions keeps the scope for probabilistic generative methods. This paper discusses the usability of machine learning techniques from both the classes: generative and discriminative along with the characteristics of data that enables them.
Key-Words / Index Term
Discriminative, Financial market, Generative, Machine Learning, Sentiment Analysis, Supervised Learning
References
[1] K. Wang, C. Zong, and K.-Y. Su, “Integrating Generative and Discriminative Character-Based Models for Chinese Word Segmentation,” ACM Trans. Asian Lang. Inf. Process., vol. 11, no. 2, pp. 1–41, 2012.
[2] A. Y. Ng and M. I. Jordon, On Discriminative vs. Generative Classifiers: A comparison of logistic regression and naive Bayes. MIT Press, 2002.
[3] P. M. Long, R. A. Servedio, and H. U. Simon, “Discriminative learning can succeed where generative learning fails,” Inf. Process. Lett., vol. 103, no. 4, pp. 131–135, 2007.
[4] J. L. Ticknor, “A Bayesian regularized artificial neural network for stock market forecasting,” Expert Syst. Appl., vol. 40, no. 14, pp. 5501–5506, 2013.
[5] L. Deng and N. Jaitly, “Deep Discriminative and Generative Models for Pattern Recognition,” in Handbook Of Pattern Recognition And Computer Vision (5th Edition), Fifth., C. C. Hau, Ed. World Scientific Press, 2015, pp. 1–26.
[6] T. S. B. Fletcher, “Machine learning for financial market prediction,” p. 207, 2012.
[7] C. M. Bishop, Pattern Recognition and Machine Learning (Information Science and Statistics). Springer-Verlag Berlin, Heidelberg ©2006, 2006.
[8] J. Xu, H. Li, and S. Zhou, “An Overview of Deep Generative Models,” IETE Tech. Rev., vol. 32, no. 2, pp. 131–139, 2015.
[9] J. Patel, S. Shah, P. Thakkar, and K. Kotecha, “Predicting stock market index using fusion of machine learning techniques,” Expert Syst. Appl., vol. 42, no. 4, pp. 2162–2172, 2015.
[10] B. K. Wong and Y. Selvi, “Neural network applications in finance: A review and analysis of literature (1990–1996),” Inf. Manag., vol. 34, no. 3, pp. 129–139, 1998.
[11] G. S. Atsalakis and K. P. Valavanis, “Surveying stock market forecasting techniques - Part II: Soft computing methods,” Expert Syst. Appl., vol. 36, no. 3 PART 2, pp. 5932–5941, 2009.
[12] R. C. Cavalcante, R. C. Brasileiro, V. L. F. Souza, J. P. Nobrega, and A. L. I. Oliveira, “Computational Intelligence and Financial Markets: A Survey and Future Directions,” Expert Syst. Appl., vol. 55, pp. 194–211, 2016.
[13] E. A. Gerlein, M. McGinnity, A. Belatreche, and S. Coleman, “Evaluating machine learning classification for financial trading: An empirical approach,” Expert Syst. Appl., vol. 54, pp. 193–207, 2016.
[14] A. Khadjeh Nassirtoussi, S. Aghabozorgi, T. Ying Wah, and D. C. L. Ngo, “Text mining for market prediction: A systematic review,” Expert Syst. Appl., vol. 41, no. 16, pp. 7653–7670, 2014.
[15] B. S. Kumar and V. Ravi, A survey of the applications of text mining in financial domain, vol. 114. Elsevier B.V., 2016.
[16] F. Z. Xing, E. Cambria, and R. E. Welsch, “Natural language based financial forecasting: a survey,” Artif. Intell. Rev., pp. 1–25, 2017.
[17] P. Hájek, “Combining bag-of-words and sentiment features of annual reports to predict abnormal stock returns,” Neural Comput. Appl., vol. 29, no. 7, pp. 343–358, 2018.
[18] Q. Li, J. Wang, F. Wang, P. Li, L. Liu, and Y. Chen, “The role of social sentiment in stock markets : a view from joint effects of multiple information sources,” Multimed. Tools Appl., 2016.
[19] J. Padmanabhan and M. J. Johnson Premkumar, “Machine Learning in Automatic Speech Recognition: A Survey,” IETE Tech. Rev., vol. 32, no. 4, pp. 240–251, 2015.