Modeling Customer’s Credit Worthiness using Enhanced Ensemble Model
M. Singh1 , G. K. Dixit2
Section:Research Paper, Product Type: Journal Paper
Volume-6 ,
Issue-7 , Page no. 1466-1470, Jul-2018
CrossRef-DOI: https://doi.org/10.26438/ijcse/v6i7.14661470
Online published on Jul 31, 2018
Copyright © M. Singh, G. K. Dixit . This is an open access article distributed under the Creative Commons Attribution License, which permits unrestricted use, distribution, and reproduction in any medium, provided the original work is properly cited.
View this paper at Google Scholar | DPI Digital Library
How to Cite this Paper
- IEEE Citation
- MLA Citation
- APA Citation
- BibTex Citation
- RIS Citation
IEEE Citation
IEEE Style Citation: M. Singh, G. K. Dixit, “Modeling Customer’s Credit Worthiness using Enhanced Ensemble Model,” International Journal of Computer Sciences and Engineering, Vol.6, Issue.7, pp.1466-1470, 2018.
MLA Citation
MLA Style Citation: M. Singh, G. K. Dixit "Modeling Customer’s Credit Worthiness using Enhanced Ensemble Model." International Journal of Computer Sciences and Engineering 6.7 (2018): 1466-1470.
APA Citation
APA Style Citation: M. Singh, G. K. Dixit, (2018). Modeling Customer’s Credit Worthiness using Enhanced Ensemble Model. International Journal of Computer Sciences and Engineering, 6(7), 1466-1470.
BibTex Citation
BibTex Style Citation:
@article{Singh_2018,
author = {M. Singh, G. K. Dixit},
title = {Modeling Customer’s Credit Worthiness using Enhanced Ensemble Model},
journal = {International Journal of Computer Sciences and Engineering},
issue_date = {7 2018},
volume = {6},
Issue = {7},
month = {7},
year = {2018},
issn = {2347-2693},
pages = {1466-1470},
url = {https://www.ijcseonline.org/full_paper_view.php?paper_id=2628},
doi = {https://doi.org/10.26438/ijcse/v6i7.14661470}
publisher = {IJCSE, Indore, INDIA},
}
RIS Citation
RIS Style Citation:
TY - JOUR
DO = {https://doi.org/10.26438/ijcse/v6i7.14661470}
UR - https://www.ijcseonline.org/full_paper_view.php?paper_id=2628
TI - Modeling Customer’s Credit Worthiness using Enhanced Ensemble Model
T2 - International Journal of Computer Sciences and Engineering
AU - M. Singh, G. K. Dixit
PY - 2018
DA - 2018/07/31
PB - IJCSE, Indore, INDIA
SP - 1466-1470
IS - 7
VL - 6
SN - 2347-2693
ER -
![]() |
![]() |
![]() |
513 | 428 downloads | 259 downloads |
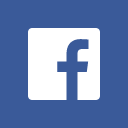
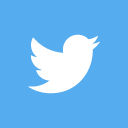
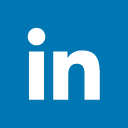
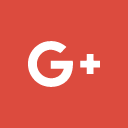
Abstract
The financial organizations such as banks assess credit worthiness of borrowers before providing new loans. Certain assessments are done on the basis of ‘probability of default’ for the potential borrower which is based on credit scoring of customer. Banks are having customer`s portfolios that are likely to go through current crisis without much difficulty, but at the same time it is difficult to determine the risks arising due to various factors. Most of the features in customer databases have little predictive effect on the credit worthiness of the customer. So, determining features that affects the credit worthiness is another important task before knowledge discovery. The predictive models, based on Ensemble Methods (EM): a ML paradigm under Data Mining (DM), have the capability to determine the relevant features and customer’s credit worthiness in efficient manner. In this paper, a good number of prediction models proposed in the literature are surveyed. To deal with major challenges like data incompleteness, noise and its vastness while building predictive models, this work proposes a predictive framework (classifier) for detection of Credit Worthiness. It employs a Supervised Attribute (Feature) selection method to select the worth of subset, filters and an Enhanced Ensemble Method based on Boosting for precise convergence. The proposed Enhanced Ensemble based classifier is implemented in Weka 3.8.2. Later in this work, to know the performance of proposed model, evaluation and comparison against several model is presented. The proposed framework selects minimum number of attributes and is good in terms of False Positive, Recall, True Positive and Prediction Rate than original base classifiers. Also, the model is good for applying on large datasets due the benefit of ensembles.
Key-Words / Index Term
Artificial Intelligence, Boosting, Classification, Credit Risk, Credit Worthiness, Ensemble Methods, Machine Learning, Predictive Models, Weka
References
[1] Polikar, R. (2006). Ensemble based systems in decision making. IEEE Circuits and Systems Magazine, 6(3), 21–45.
[2] Loris Nanni , Alessandra Lumini, “An experimental comparison of ensemble of classifiers for bankruptcy prediction and credit scoring”, Elsevier, Expert Systems with Applications 36 (2009) 3028–3033.
[3] Stuti Nathaniel, Anand Motwani, Arpit Saxena, "Cloud based Predictive Model for Detection of ‘Chronic Kidney Disease’ Risk", International Journal of Computer Sciences and Engineering, Vol.6, Issue.4, pp.185-188, 2018.
[4] Bhekisipho Twala, “Multiple classifier application to credit risk assessment”, Expert Systems with Applications 37 (2010) 3326–3336.
[5] Gang Wang, Jinxing Hao, Jian Mab, Hongbing Jiang, “A comparative assessment of ensemble learning for credit scoring”, Expert Systems with Applications 38 (2011) 223–230.
[6] Zhou, Z. H. (2009). Ensemble. In L. Liu & T. Özsu (Eds.), Encyclopedia of database systems. Berlin: Springer.
[7] Regina Esi Turkson, Edward Yeallakuor Baagyere, Gideon Evans Wenya, “A Machine Learning Approach for Predicting Bank Credit Worthiness”, ISBN: 978-1-4673-9187-0, IEEE 2016
[8] F. Pedregosa, G. Varoquaux, A. Gramfort, V. Michel, B. Thirion, O. Grisel, M. Blondel, P. Prettenhofer, R. Weiss, V. Dubourg, J. Vanderplas, A. Passos, D. Cournapeau, M. Brucher, M. Perrot, and E. Duchesnay, “Scikit-learn: Machine learning in Python,” Journal of Machine Learning Research, vol. 12, pp. 2825–2830, 2011.
[9] C.R.Durga devi, Dr.R.Manicka chezian, “A Relative Evaluation of the Performance of Ensemble Learning in Credit Scoring”, IEEE International Conference on Advances in Computer Applications (ICACA), 978-1-5090-3770-4/16, 2016
[10] Zhang, “Z., Research of credit risk of commercial bank`s personal loan based on CHAID decision tree”, Artificial Intelligence, Management Science and Electronic Commerce (AIMSEC), 2011.
[11] http://www.cs.waikato.ac.nz/~ml/weka/index.html
[12] Weka, University of Waikato, Hamilton, New Zealand.
[13] Anand Motwani, Goldi Bajaj, Sushila Mohane, "Predictive Modelling for Credit Risk Detection using Ensemble Method", International Journal of Computer Sciences and Engineering, Vol.6, Issue.6, pp.863-867, 2018.
[14] Ling Kock Sheng, and Teh Ying Wah, “A comparative study of data mining techniques in predicting consumers’ credit card risk in banks”, African Journal of Business Management Vol. 5 (20), pp. 8307-8312, 16 September, 2011
[15] http://archive.ics.uci.edu/ml/datasets/default+of+credit+card+clients