Predicting Rating from Textual Reviews
Arshiya Begum1 , Ruksar Fatima2
Section:Review Paper, Product Type: Journal Paper
Volume-6 ,
Issue-7 , Page no. 1512-1516, Jul-2018
CrossRef-DOI: https://doi.org/10.26438/ijcse/v6i7.15121516
Online published on Jul 31, 2018
Copyright © Arshiya Begum, Ruksar Fatima . This is an open access article distributed under the Creative Commons Attribution License, which permits unrestricted use, distribution, and reproduction in any medium, provided the original work is properly cited.
View this paper at Google Scholar | DPI Digital Library
How to Cite this Paper
- IEEE Citation
- MLA Citation
- APA Citation
- BibTex Citation
- RIS Citation
IEEE Style Citation: Arshiya Begum, Ruksar Fatima, “Predicting Rating from Textual Reviews,” International Journal of Computer Sciences and Engineering, Vol.6, Issue.7, pp.1512-1516, 2018.
MLA Style Citation: Arshiya Begum, Ruksar Fatima "Predicting Rating from Textual Reviews." International Journal of Computer Sciences and Engineering 6.7 (2018): 1512-1516.
APA Style Citation: Arshiya Begum, Ruksar Fatima, (2018). Predicting Rating from Textual Reviews. International Journal of Computer Sciences and Engineering, 6(7), 1512-1516.
BibTex Style Citation:
@article{Begum_2018,
author = {Arshiya Begum, Ruksar Fatima},
title = {Predicting Rating from Textual Reviews},
journal = {International Journal of Computer Sciences and Engineering},
issue_date = {7 2018},
volume = {6},
Issue = {7},
month = {7},
year = {2018},
issn = {2347-2693},
pages = {1512-1516},
url = {https://www.ijcseonline.org/full_paper_view.php?paper_id=2635},
doi = {https://doi.org/10.26438/ijcse/v6i7.15121516}
publisher = {IJCSE, Indore, INDIA},
}
RIS Style Citation:
TY - JOUR
DO = {https://doi.org/10.26438/ijcse/v6i7.15121516}
UR - https://www.ijcseonline.org/full_paper_view.php?paper_id=2635
TI - Predicting Rating from Textual Reviews
T2 - International Journal of Computer Sciences and Engineering
AU - Arshiya Begum, Ruksar Fatima
PY - 2018
DA - 2018/07/31
PB - IJCSE, Indore, INDIA
SP - 1512-1516
IS - 7
VL - 6
SN - 2347-2693
ER -
![]() |
![]() |
![]() |
458 | 262 downloads | 265 downloads |
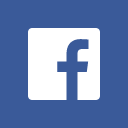
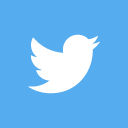
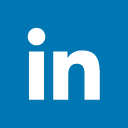
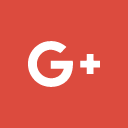
Abstract
As of late, we have seen a twist of audit sites. It displays an incredible chance to share our perspectives for different items we buy. Be that as it may, we confront the data over-burdening issue. Instructions to mine significant data from surveys to comprehend a client`s inclinations and make an exact proposal is vital. Customary recommender frameworks (RS) think about a few components, for example, client`s buy records, item classification, and geographic area. In this work, we propose a conclusion based rating expectation technique (RPS) to enhance forecast exactness in recommender frameworks. Initially, we propose a social client nostalgic estimation approach and ascertain every client`s notion on things/items. Besides, we consider a client`s own wistful properties as well as mull over relational nostalgic impact. At that point, we think about item notoriety, which can be surmised by the wistful appropriations of a client set that mirror clients` thorough assessment. Finally, we intertwine three components client supposition likeness, relational wistful impact, and thing`s notoriety closeness into our recommender framework to make a precise rating forecast. We direct an execution assessment of the three nostalgic factors on a genuine dataset gathered from Yelp.
Key-Words / Index Term
Meta-Data, Rating prediction, yelp
References
[1]. Li, Yu, Liu Lu, and Li Xuefeng. "A hybrid collaborative filtering method for multiple-interests and multiple-content recommendation in E-Commerce." Expert systems with applications 28.1 (2005): 67-77.
[2]. Liu, Bing. "Sentiment analysis and opinion mining." Synthesis lectures on human language technologies 5.1 (2012): 1-167.
[3]. Pang, Bo, and Lillian Lee. "Opinion mining and sentiment analysis." Foundations and Trends® in Information Retrieval 2.1–2 (2008): 1-135.
[4]. Meshram, Milind D. "Feature based opinion mining: an overview." Proceedings of National Conference on Emerging Trends: Innovations and Challenges in IT. Vol. 19. 2013.
[5]. Bucur, Cristian. "Opinion Mining platform for Intelligence in business." Economic Insights--Trends and Challenges 66.3 (2014).
[6]. Osimo, David, and Francesco Mureddu. "Research challenge on opinion mining and sentiment analysis." Universite de Paris-Sud, Laboratoire LIMSI-CNRS, Bâtiment 508 (2012).
[7]. Vinodhini, G., and R. M. Chandrasekaran. "Sentiment analysis and opinion mining: a survey." International Journal 2.6 (2012): 282-292.
[8]. Varghese, Raisa, and M. Jayasree. "A survey on sentiment analysis and opinion mining." International Journal of Research in Engineering and Technology 2.11 (2013): 312-317.
[9]. Kaur, Amandeep, and Vishal Gupta. "A survey on sentiment analysis and opinion mining techniques." Journal of Emerging Technologies in Web Intelligence 5.4 (2013): 367-371.
[10]. Lin, Chenghua, et al. "Weakly supervised joint sentiment-topic detection from text." IEEE Transactions on Knowledge and Data engineering 24.6 (2012): 1134-1145.
[11]. Pang, Bo, and Lillian Lee. "Opinion mining and sentiment analysis." Foundations and Trends® in Information Retrieval 2.1–2 (2008): 1-135.
[12]. Pang, Bo, Lillian Lee, and Shivakumar Vaithyanathan. "Thumbs up? sentiment classification using machine learning techniques." Proceedings of the ACL-02 conference on Empirical methods in natural language processing-Volume 10. Association for Computational Linguistics, 2002.
[13]. Dave, Kushal, Steve Lawrence, and David M. Pennock. "Mining the peanut gallery: Opinion extraction and semantic classification of product reviews." Proceedings of the 12th international conference on World Wide Web. ACM, 2003.
[14]. Blitzer, John, Mark Dredze, and Fernando Pereira. "Biographies, boom-boxes and blenders: Domain adaptation for sentiment classification." ACL. Vol. 7. 2007.
[15]. Whitelaw, Casey, Navendu Garg, and Shlomo Argamon. "Using appraisal groups for sentiment analysis." Proceedings of the 14th ACM international conference on Information and knowledge management. ACM, 2005.
[16]. Kennedy, Alistair, and Diana Inkpen. "Sentiment classification of movie reviews using contextual valence shifters." Computational intelligence 22.2 (2006): 110-125.
[17]. Ye, Qiang, Ziqiong Zhang, and Rob Law. "Sentiment classification of online reviews to travel destinations by supervised machine learning approaches." Expert systems with applications 36.3 (2009): 6527-6535.