Breast Cancer Prediction Using Soft Computing Techniques – A Survey
M. Durairaj1 , K. Priya2
Section:Survey Paper, Product Type: Journal Paper
Volume-6 ,
Issue-8 , Page no. 135-145, Aug-2018
CrossRef-DOI: https://doi.org/10.26438/ijcse/v6i8.135145
Online published on Aug 31, 2018
Copyright © M. Durairaj, K. Priya . This is an open access article distributed under the Creative Commons Attribution License, which permits unrestricted use, distribution, and reproduction in any medium, provided the original work is properly cited.
View this paper at Google Scholar | DPI Digital Library
How to Cite this Paper
- IEEE Citation
- MLA Citation
- APA Citation
- BibTex Citation
- RIS Citation
IEEE Style Citation: M. Durairaj, K. Priya, “Breast Cancer Prediction Using Soft Computing Techniques – A Survey,” International Journal of Computer Sciences and Engineering, Vol.6, Issue.8, pp.135-145, 2018.
MLA Style Citation: M. Durairaj, K. Priya "Breast Cancer Prediction Using Soft Computing Techniques – A Survey." International Journal of Computer Sciences and Engineering 6.8 (2018): 135-145.
APA Style Citation: M. Durairaj, K. Priya, (2018). Breast Cancer Prediction Using Soft Computing Techniques – A Survey. International Journal of Computer Sciences and Engineering, 6(8), 135-145.
BibTex Style Citation:
@article{Durairaj_2018,
author = {M. Durairaj, K. Priya},
title = {Breast Cancer Prediction Using Soft Computing Techniques – A Survey},
journal = {International Journal of Computer Sciences and Engineering},
issue_date = {8 2018},
volume = {6},
Issue = {8},
month = {8},
year = {2018},
issn = {2347-2693},
pages = {135-145},
url = {https://www.ijcseonline.org/full_paper_view.php?paper_id=2668},
doi = {https://doi.org/10.26438/ijcse/v6i8.135145}
publisher = {IJCSE, Indore, INDIA},
}
RIS Style Citation:
TY - JOUR
DO = {https://doi.org/10.26438/ijcse/v6i8.135145}
UR - https://www.ijcseonline.org/full_paper_view.php?paper_id=2668
TI - Breast Cancer Prediction Using Soft Computing Techniques – A Survey
T2 - International Journal of Computer Sciences and Engineering
AU - M. Durairaj, K. Priya
PY - 2018
DA - 2018/08/31
PB - IJCSE, Indore, INDIA
SP - 135-145
IS - 8
VL - 6
SN - 2347-2693
ER -
![]() |
![]() |
![]() |
782 | 363 downloads | 218 downloads |
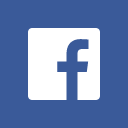
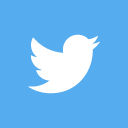
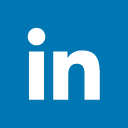
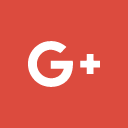
Abstract
Breast cancer is a disease where there is excessive growth or uncontrolled growth of cells of the breast tissue. Breast cancer is a type of cancer that is often found as lump, bloody nipple, pain or sore and change in size in the most of the cases. Breast cancer occurs when the cell tissues of the breast become abnormal and uncontrollably divided. These abnormal cells form a large lump of tissues, which consequently becomes a tumor. Digital imaging techniques like Scintimammography are used to analyse metabolic activities and vascular circulation for pre-cancerous analysis based on breast tissue. Finally biopsy result is used to ascertain cancer when other physical exam and mammograms show breast change. Soft computing techniques are interestingly gaining popularity in medical disease diagnosis and decision making. There are different soft computing techniques for medical data processing. These techniques can be used individually or hybridizing more to process medical data yields near accurate results in decision making by medical practioners. This paper reviews different breast cancer diagnosis methodologies which use information obtained from imaging techniques such as Magnetic Resonance Imaging (MRI), Mammogram, Ultrasound and Biopsy. Aim of this paper is to propose or identify methodologies that process cancerous images obtained from different imaging techniques and predict breast cancer with relatively better accuracy. In this paper, we reviewed research articles published in the recent years on breast cancer prediction using soft computing techniques. Comparative analysis of different methods in terms of accuracy, sensitivity, specificity and computational time is presented.
Key-Words / Index Term
Soft Computing, Machine Learning, Breast Cancer, Disease Diagnosis, Mammograms, MRI, Classification
References
[1] FeiHan, Guilian Shi, Chengwen Liang, Lin Wang, Kaiyang Li, “A simple and efficient method for breast cancer diagnosis based on infrared thermal imaging”, Cell biochemistry and biophysics, Vol. 71, pp. 491-498, 2015.
[2] Luciano Boquete, Sergio Ortego, Juan Manuel Miguel-Jimenez, Jose Manuel, Roman Blanco, “Automated detection of breast cancer in thermal infrared images, based on independent component analysis”’ Journal of medical systems, Vol. 36, pp. 103-111, 2012.
[3] Ali, Mona AS, Gehad Ismail Sayed, Tarek Gaber, Aboul Ella Hassanien, Vclav Snasel, Lincoln F. Silva, “Detection of breast abnormalities of thermograms based on a new segmentation method”, In Proceeding of Computer Science and Information Systems (FedCSIS), 2015 Federated Conference on IEEE, Vol. 5, pp. 255-261, 2015.
[4] W. Peng, R.V. Mayorga, E.M.A. Hussein, “An Automated Confirmatory System for Analysis of Mammograms”, Computer Methods and Programs in Biomedicine, Vol. , pp. 1-22, 2016.
[5] Hossein Ghayoumi Zadeh, Javad Haddadnia, Maryam Hashemian, Kazem Hassanpour, “Diagnosis of breast cancer using a combination of genetic algorithm and artificial neural network in medical infrared thermal imaging”, Iranian Journal of Medical Physics, Vol. 9, No. 4, pp. 265-274, 2012.
[6] Neha Tuteja, Parvinder Singh, MohitBansal, “Comparative analysis of different techniques for breast cancer detection in Mammograms”, International Journal of Advnce Research, Ideas and Innovtions in Technology, Vol. 4, No. 2, pp. 1361-1365, 2018.
[7] Anuj Kumar Singh, Bhupendra Gupta, “A novel approach for breast cancer detection and segmentation in a mammogram”, Procedia Computer Science, Vol. 54, pp. 676-682, 2015.
[8] Essam A. Rashed, Ismail A. Ismail, Sherif I. Zaki, “Multiresolution mammogram analysis in multilevel decomposition”, Pattern Recognition Letters, Vol. 28, pp. 286-292, 2007.
[9] Prathibha, B. N., and V. Sadasivam, “Mammogram analysis using the SVM classifier in combined transforms domain”, ICTACT Journal on Image and Video Processing, Vol. 1, No. 03, pp. 172-177, 2011.
[10] Nebi Gedik, AytenAtasoy, “A computer-aided diagnosis system for breast cancer detection by using a curvelet transform”, Turkish Journal of Electrical Engineering & Computer Sciences, Vol. 21, pp. 1002-1014, 2013.
[11] M. Duraraj and R. Deepika, “Comparative Analysis of Classification Algorithms for the Prediction of Leukemia Cancer”, International Journal of Advanced Research in Computer Science and Software Engineering, Vol. 4, No. 6, pp. 787–791, Aug. 2015.
[12] Ahmet Mert, NiyaziKilic, ErdemBilgili, Aydin Akan, “Breast Cancer Detection with Reduced Feature Set”, Computational and Mathematical Methods in Medicine, Vol. 2015, article Id 265138, pp. 1-12, 2015.
[13] Zhiming Li, MD, Lan Yu, MD, Xin Wang, MD, Haiyang Yu, MD, Yuanxiang Gao, MD,Yande Ren, MD, Gang Wang, MD, Xiaoming Zhou, MD, “Diagnostic performance of mammographic texture analysis in the differentialdiagnosis of benign and malignant breast tumors”, Clinical Breast Cancer, doi: 10.1016/j.clbc.2017.11.004, 2017.
[14] M. J. Lado, C. Cadarso-Suarez, J. Roca-Pardinas and P. G. Tahoces, “Using generalized additive models for construction of nonlinear classifiers in computer-aided diagnosis systems”, In proceedings of the IEEE Transactions on Information Technology in Biomedicine, Vol. 10, No. 2, pp. 246-253, April 2006.
[15] Petroudi S., Constantinou I., Pattichis M., Tziakouri C., Marias K., Pattichis C, “Evaluation of Spatial Dependence Matrices on Multiscale Instantaneous Amplitude for Mammogram Classification”, In: Lacković I., Vasic D. (eds) 6th European Conference of the International Federation for Medical and Biological Engineering. IFMBE Proceedings, Vol. 45, 2015.
[16] Selvan, S, Cecil Xavier, C,Karssemeijer, Nico,Sequeira, Jean, A. Cherian, Rekha, Y. Dhala, Bharathi, “Parameter Estimation in Stochastic Mammogram Model by Heuristic Optimization Techniques”, Information Technology in Biomedicine,” IEEE Transactions on. Vol. 10, pp. 685 – 695, 2006.
[17] Li Zhang, Wei-Da Zhou, “Fisher-regularized support vector machine”, Information Sciences, Elsevier, Vol. 343, pp. 79-93, 2016.
[18] Cong Y.C., Brady M., Petroudi S, “Texture Based Mammogram Classification and Segmentation”, In: Astley S.M., Brady M., Rose C., Zwiggelaar R. (eds) Digital Mammography. IWDM 2006. Lecture Notes in Computer Science, Vol. 4046, Springer, Berlin, Heidelberg, 2006.
[19] M. Durairaj, R. Nandhakumar, “Feature Diminution by Hybrid Algorithm for improving the Success Rate for IVF Treatment,” Pakistan Journal of Biotechnology, Vol. 14, No. Sp-II, pp. 1100–1104, 2017.
[20] Woo Kyung Moona, I-Ling Chenb, Ann Yi, Min Sun Baea, Sung Ui Shina, Ruey-Feng Chang, “Computer-aided prediction model for axillary lymph node metastasisin breast cancer using tumor morphological and textural features on Ultrasound”, Computer Methods and Programs in Biomedicine, Elsevier, Vol. 162, pp. 129-137, 2018.