A Non-Dominated Sorting TLBO Algorithm for Multi-Objective Short-Term Hydrothermal Self Scheduling of GENCOs in a Competitive Electricity Market
Pasupulati Baburao1 , R Ashok Kumar2 , Balamurugan G3 , K Asokan4
Section:Research Paper, Product Type: Journal Paper
Volume-6 ,
Issue-8 , Page no. 191-203, Aug-2018
CrossRef-DOI: https://doi.org/10.26438/ijcse/v6i8.191203
Online published on Aug 31, 2018
Copyright © Pasupulati Baburao, R Ashok Kumar, Balamurugan G, K Asokan . This is an open access article distributed under the Creative Commons Attribution License, which permits unrestricted use, distribution, and reproduction in any medium, provided the original work is properly cited.
View this paper at Google Scholar | DPI Digital Library
How to Cite this Paper
- IEEE Citation
- MLA Citation
- APA Citation
- BibTex Citation
- RIS Citation
IEEE Citation
IEEE Style Citation: Pasupulati Baburao, R Ashok Kumar, Balamurugan G, K Asokan, “A Non-Dominated Sorting TLBO Algorithm for Multi-Objective Short-Term Hydrothermal Self Scheduling of GENCOs in a Competitive Electricity Market,” International Journal of Computer Sciences and Engineering, Vol.6, Issue.8, pp.191-203, 2018.
MLA Citation
MLA Style Citation: Pasupulati Baburao, R Ashok Kumar, Balamurugan G, K Asokan "A Non-Dominated Sorting TLBO Algorithm for Multi-Objective Short-Term Hydrothermal Self Scheduling of GENCOs in a Competitive Electricity Market." International Journal of Computer Sciences and Engineering 6.8 (2018): 191-203.
APA Citation
APA Style Citation: Pasupulati Baburao, R Ashok Kumar, Balamurugan G, K Asokan, (2018). A Non-Dominated Sorting TLBO Algorithm for Multi-Objective Short-Term Hydrothermal Self Scheduling of GENCOs in a Competitive Electricity Market. International Journal of Computer Sciences and Engineering, 6(8), 191-203.
BibTex Citation
BibTex Style Citation:
@article{Baburao_2018,
author = {Pasupulati Baburao, R Ashok Kumar, Balamurugan G, K Asokan},
title = {A Non-Dominated Sorting TLBO Algorithm for Multi-Objective Short-Term Hydrothermal Self Scheduling of GENCOs in a Competitive Electricity Market},
journal = {International Journal of Computer Sciences and Engineering},
issue_date = {8 2018},
volume = {6},
Issue = {8},
month = {8},
year = {2018},
issn = {2347-2693},
pages = {191-203},
url = {https://www.ijcseonline.org/full_paper_view.php?paper_id=2675},
doi = {https://doi.org/10.26438/ijcse/v6i8.191203}
publisher = {IJCSE, Indore, INDIA},
}
RIS Citation
RIS Style Citation:
TY - JOUR
DO = {https://doi.org/10.26438/ijcse/v6i8.191203}
UR - https://www.ijcseonline.org/full_paper_view.php?paper_id=2675
TI - A Non-Dominated Sorting TLBO Algorithm for Multi-Objective Short-Term Hydrothermal Self Scheduling of GENCOs in a Competitive Electricity Market
T2 - International Journal of Computer Sciences and Engineering
AU - Pasupulati Baburao, R Ashok Kumar, Balamurugan G, K Asokan
PY - 2018
DA - 2018/08/31
PB - IJCSE, Indore, INDIA
SP - 191-203
IS - 8
VL - 6
SN - 2347-2693
ER -
![]() |
![]() |
![]() |
581 | 352 downloads | 290 downloads |
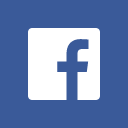
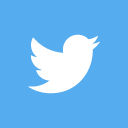
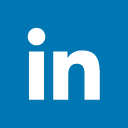
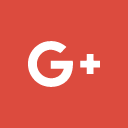
Abstract
In competitive electricity market worldwide raises many challenging tasks related to the economic and optimal operation of electric power systems. In deregulated market structure, the generation is being despatched by means of hourly power delivery. The penalty is improved on power producers, if they fail to attain the planned energy delivery. The inadequate hydel resources associated with environmental constraints of thermal plants necessiates a precise scheduling system to satisfy the ever growing power demand. The power generator in a hydrothermal has to manage the conflicting objectives of profit maximization and emission minimization. Normally, the multi-objective optimization problem is tuned for optimising the two or more conflicting objectives subject to some constraints. Short-term hydrothermal scheduling (STHTS) problem deals with more objective functions such as profit maximization and emission minimization. Hence it is necessary to evolve a constructive framework based on intelligent techniques. In this paper, a stochastic multi-objective model is derived for the flexible scheduling of hydrothermal plants with valve-point loading effects. A non-dominated sorting teaching learning based optimization (NSTLBO) algorithm is presented for solving STHTS problem. The proposed algorithm is applied to derive a pair of non-dominated results and then the fuzzy based methodology has been argued to choose the best solution. It is tested on a three thermal and four hydro test system with twenty four hour time period. The results are extracted by means of total profit and emission from the plants. Comparative studies have also been done to validate the viability of the proposed method.
Key-Words / Index Term
Deregulation, Hydrothermal Scheduling, Profit Maximization, Emission Limitations, Non-dominated Sorting TLBO algorithm
References
[1] A. J. Wood and B. F. Wollenberg, Power Generation, Operation, and Control, vol. 37. 1996.
[2] M. Shahidehpour, H. Yamin, and Z. Li, Market Operations in Electric Power Systems : Forecasting, Scheduling, and Risk Management, vol. 9. 2002.
[3] Mohammad Shahidehpour, Muwaffaq, Alomoush, Restructured electrical power systems, Operation, Trading, and volatility, John Wiley & Sons (New York, 2000).
[4] J. L. Golden, C. J. Stansberry, R. L. Vice, J. T. Wood, J. Ballance, G. Brown, K. Kamya, E. K. Nielsen, H. Nakajima, M. Ookubo, I. Iyoda, and G. W. Cauley, “Potential Impacts of Clean Air Regulations on System Operations,” IEEE Trans. Power Syst., vol. 10, no. 2, pp. 647–656, 1995.
[5] M. Basu, “A simulated annealing-based goal-attainment method for economic emission load dispatch of fixed head hydrothermal power systems,” Int. J. Electr. Power Energy Syst., vol. 27, no. 2, pp. 147–153, 2005.
[6] M. Basu, “Goal-attainment method based on simulated annealing technique for economic-environmental-dispatch of hydrothermal power systems with cascaded reservoirs,” Electr. Power Components Syst., vol. 32, no. 12, pp. 1269–1286, 2004.
[7] C. L. Chiang, “Optimal economic emission dispatch of hydrothermal power systems,” Int. J. Electr. Power Energy Syst., vol. 29, no. 6, pp. 462–469, 2007.
[8] K. K. Mandal and N. Chakraborty, “Short-term combined economic emission scheduling of hydrothermal systems with cascaded reservoirs using particle swarm optimization technique,” Appl. Soft Comput. J., vol. 11, no. 1, pp. 1295–1302, 2011.
[9] G. Nadakuditi, V. Sharma, and R. Naresh, “Non-dominated Sorting Disruption-based Gravitational Search Algorithm with Mutation Scheme for Multi-objective Short-Term Hydrothermal Scheduling,” Electr. Power Components Syst., vol. 44, no. 9, pp. 990–1004, 2016.
[10] A. Ahmadi, J. Aghaei, H. A. Shayanfar, and A. Rabiee, “Mixed integer programming of multiobjective hydro-thermal self scheduling,” Appl. Soft Comput. J., vol. 12, no. 8, pp. 2137–2146, 2012.
[11] S. Padmini, R. Jegatheesan, and D. F. Thayyil, “A Novel Method for Solving Multi-Objective Hydrothermal Unit Commitment and Sheduling for GENCO Using Hybrid LR-EP Technique,” in Procedia Computer Science, 2015, vol. 57, pp. 258–268.
[12] R. V. Rao, D. P. Rai, and J. Balic, “Multi-objective optimization of machining and micro-machining processes using non-dominated sorting teaching–learning-based optimization algorithm,” Journal of Intelligent Manufacturing, pp. 1–23, 2016.
[13] P. Attaviriyanupap, H. Kita, E. Tanaka, and J. Hasegawa, “A hybrid LR-EP for solving new profit-based UC problem under competitive environment,” IEEE Trans. Power Syst., vol. 18, no. 1, pp. 229–237, 2003.
[14] K. Asokan, and R. Ashok Kumar, “An Innovative approach for self Scheduling of Generation companies to maximize the Profit by considering Reserve generation”, Australian Journal of Basic and Applied Sciences, vol. 8(Issue 6):179-185, 2014.
[15] F. Zou, L. Wang, X. Hei, D. Chen, and B. Wang, “Multi-objective optimization using teaching-learning-based optimization algorithm”, Engineering Applications of Artificial Intelligence, vol. 26(Issue 4):1291-300, 2013.
[16] D. Li, C. Zhang, X. Shao, and W. Lin, “A multi-objective TLBO algorithm for balancing two-sided assembly line with multiple constraints,” Journal of Intelligent Manufacturing, vol. 27, no. 4. pp. 725–739, 2016.
[17] R. Venkata Rao and G. G. Waghmare, “A comparative study of a teaching-learning-based optimization algorithm on multi-objective unconstrained and constrained functions,” J. King Saud Univ. - Comput. Inf. Sci., vol. 26, no. 3, pp. 332–346, 2014.
[18] P. K. Roy, “Teaching learning based optimization for short-term hydrothermal scheduling problem considering valve point effect and prohibited discharge constraint,” Int. J. Electr. Power Energy Syst., vol. 53, no. 1, pp. 10–19, 2013.
[19] R. V. Rao and V. Patel, “An elitist teaching-learning-based optimization algorithm for solving complex constrained optimization problems,” Int. J. Ind. Eng. Comput., vol. 3, no. 4, pp. 535–560, 2012.
[20] R. V. Rao, V. J. Savsani, and J. Balic, “Teaching-learning-based optimization algorithm for unconstrained and constrained real-parameter optimization problems,” Eng. Optim., vol. 44, no. 12, pp. 1447–1462, 2012.
[21] R. V. Rao and V. Patel, “An improved teaching-learning-based optimization algorithm for solving unconstrained optimization problems,” Sci. Iran., vol. 20, no. 3, pp. 710–720, 2013.
[22] K. Yu, X. Wang, and Z. Wang, “An improved teaching-learning-based optimization algorithm for numerical and engineering optimization problems,” J. Intell. Manuf., vol. 27, no. 4, pp. 831–843, 2016.
[23] S. C. Satapathy, A. Naik, and K. Parvathi, “Weighted Teaching-Learning-Based Optimization for Global Function Optimization,” Appl. Math., vol. 4, no. 3, pp. 429–439, 2013.
[24] Q. Niu, H. Zhang, and K. Li, “An improved TLBO with elite strategy for parameters identification of PEM fuel cell and solar cell models,” Int. J. Hydrogen Energy, vol. 39, no. 8, pp. 3837–3854, 2014.
[25] T. Niknam, R. Azizipanah-Abarghooee, and J. Aghaei, “A new modified teaching-learning algorithm for reserve constrained dynamic economic dispatch,” IEEE Trans. Power Syst., vol. 28, no. 2, pp. 749–763, 2013.