A Novel Approach for Classifying Gene Expression Datasets
A. Immaculate Mercy1 , M. Chidambaram2
Section:Research Paper, Product Type: Journal Paper
Volume-6 ,
Issue-8 , Page no. 302-305, Aug-2018
CrossRef-DOI: https://doi.org/10.26438/ijcse/v6i8.302305
Online published on Aug 31, 2018
Copyright © A. Immaculate Mercy, M. Chidambaram . This is an open access article distributed under the Creative Commons Attribution License, which permits unrestricted use, distribution, and reproduction in any medium, provided the original work is properly cited.
View this paper at Google Scholar | DPI Digital Library
How to Cite this Paper
- IEEE Citation
- MLA Citation
- APA Citation
- BibTex Citation
- RIS Citation
IEEE Style Citation: A. Immaculate Mercy, M. Chidambaram, “A Novel Approach for Classifying Gene Expression Datasets,” International Journal of Computer Sciences and Engineering, Vol.6, Issue.8, pp.302-305, 2018.
MLA Style Citation: A. Immaculate Mercy, M. Chidambaram "A Novel Approach for Classifying Gene Expression Datasets." International Journal of Computer Sciences and Engineering 6.8 (2018): 302-305.
APA Style Citation: A. Immaculate Mercy, M. Chidambaram, (2018). A Novel Approach for Classifying Gene Expression Datasets. International Journal of Computer Sciences and Engineering, 6(8), 302-305.
BibTex Style Citation:
@article{Mercy_2018,
author = {A. Immaculate Mercy, M. Chidambaram},
title = {A Novel Approach for Classifying Gene Expression Datasets},
journal = {International Journal of Computer Sciences and Engineering},
issue_date = {8 2018},
volume = {6},
Issue = {8},
month = {8},
year = {2018},
issn = {2347-2693},
pages = {302-305},
url = {https://www.ijcseonline.org/full_paper_view.php?paper_id=2692},
doi = {https://doi.org/10.26438/ijcse/v6i8.302305}
publisher = {IJCSE, Indore, INDIA},
}
RIS Style Citation:
TY - JOUR
DO = {https://doi.org/10.26438/ijcse/v6i8.302305}
UR - https://www.ijcseonline.org/full_paper_view.php?paper_id=2692
TI - A Novel Approach for Classifying Gene Expression Datasets
T2 - International Journal of Computer Sciences and Engineering
AU - A. Immaculate Mercy, M. Chidambaram
PY - 2018
DA - 2018/08/31
PB - IJCSE, Indore, INDIA
SP - 302-305
IS - 8
VL - 6
SN - 2347-2693
ER -
![]() |
![]() |
![]() |
404 | 269 downloads | 255 downloads |
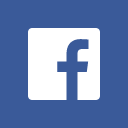
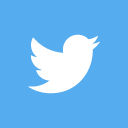
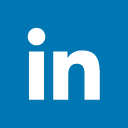
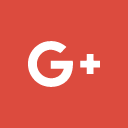
Abstract
Classification of Gene expression data is one of the challenging tasks in the domain of Bio-medical recognition. Working on high dimensional data sets always poses complexity on accuracy and on the computational fronts. A Novel approach for classifying the gene expression data has been proposed which paves path for better efficiency and effectiveness measure using an enhanced algorithm for analyzing the sequential patterns by use of a novel algorithm which surpasses the existing methods. This approach provides a better heuristics for working with both supervised and the semi-supervised data. The proposed methodology increases the efficiency by making use of the threshold values which has been used for pruning the data sets which gives rise to a higher confidence on the data sets. The classification thus achieved could help us understand the patterns using the prediction algorithm and then grouping them based on the class labels. This work and the technique that is to be used could serve us in predicting interesting knowledge on the input gene data set. As the data set is of high dimension it throws open the corridors for various analysis on the acquired classes and considerably alleviate the computation cost.
Key-Words / Index Term
Classification, Gene Expression, Supervised, Semi-supervised, pruning
References
[1] Heba Abusamra. “A comparative study of featue selection and clasification methods for gene expression data of glioma”, Procedia Science Direct , Elsevier Issue.10.1016/j.procs.2013.10.003. For Conference.
[2] Jia Lv,Qinke Peng,Xiao Chen, Zhi Sun , “A multi-objective heuristic algorithm for gene expression microaray data classification”, Elsevier, Expert Systems with Applications 59(2016)13-19.
[3] Krisztian Buza, “Classification of Gene Expression dat: A Hubness-aware semi-supervised approach”, Elsevier, Computer Methods and Programs in Biomedicine 127(2016) 105-113.
[4] Hung-Yi Lin, “Gene Discretization based on EM clustering and adaptive sequential forward gene selection for molecular classification”,Elsevier, Applied Soft Computing 48(2016) 683-690..
[5] Sara Tarek,Reda Abd Elwahab,Mahmoud Shoman, “Gene Expression based cancer classification”, Egyptian Informatics Journal 2016.
[6] Devi Arockia Vanitha ,Devaraj D,Venkatesulu, “Gene Expression Data classification using support Vector Machine and Mutual Information–based Gene selection”, Procedia Computer science 47(2015)13-21.
[7] Konstantina Kourou, Costas Papaloukas,Dimitrois I.Fotiadis, “Intergration of pathway Knowledge and Dynamic Bayesian Networks for the prediction of Oral Cancer Recurrence”, IEEE 2016.
[8] Thanh Nguyen, Saeid Nahavandi, “Modified AHP for Gene Selection and Cancer Classification using Type-2 Fuzzy Logic”, IEEE Transtions on Fuzzy Systems, Vol 24 No.2 April 2016.
[9] Jesus Maillo,Sergio Ramirez,Issac Triguero,Fransico Herrera, “kNN-IS: An interative Spark-based design of the K-nearest Neighbors classifiers for big data” Knowledge based Systems, Elsevier 000(2016)1-13.
[10] Pradeep K.Sharma, Vaibhav Sharma, Jagrati Nagdiya, “ A Proposed Method for Mining High Quality itemset with Transactional weighted utility using Genetic alogirthm Technique”, IJSRCSE, Vol -5, Issue 1, pp 31-35, 2017.
[11] T. Senthilselvi, R.Parimala, “Improving Clustering Accuracy using Feature Extraction Method “, IJSRCSE, Vol-6, Issue-2, pp 15-19, 2108.